Top 6 AI Data Collection Challenges & Solutions in 2024
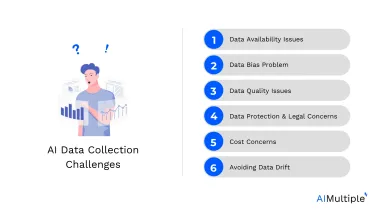
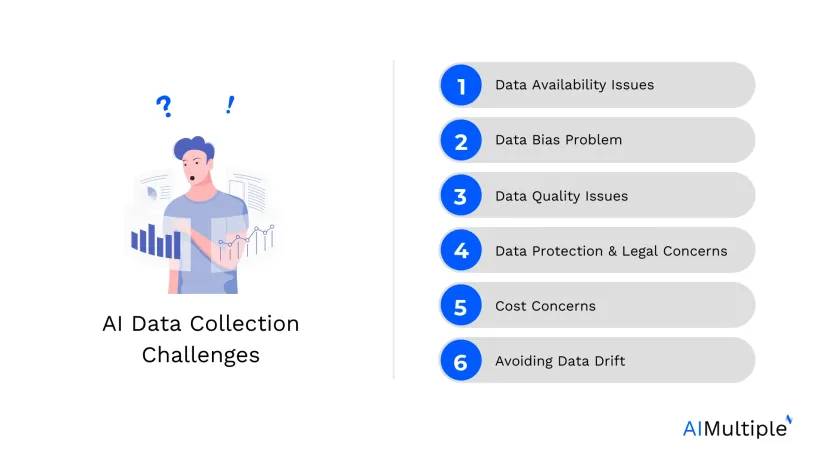
AI adoption was at a slight decline last year (Figure 1); one of the reasons for that could be the various challenges in implementing AI. Training data collection has been identified as one of the main barriers to AI adoption. To avoid data-related challenges, businesses are opting to work with data collection services.
This article explores 6 data collection problems and solutions to help streamline the AI adoption and development process for businesses and tech leaders.
Figure 1. AI adoption 20221
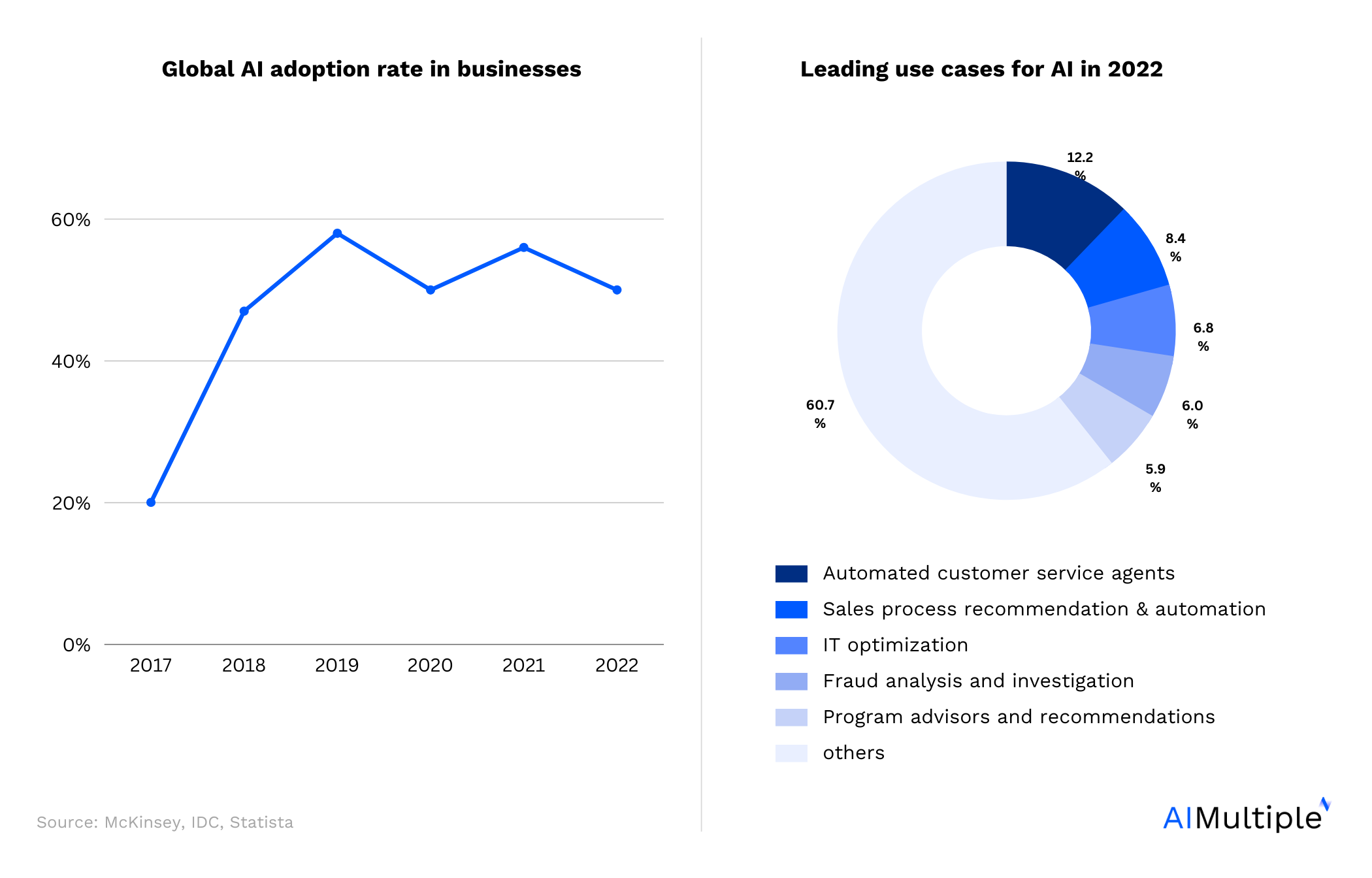
1. Dataset availability issues
Data can be considered as fuel for an AI/ML system. Determining the right dataset and ensuring data availability are crucial steps of the data collection process. One of the key challenges that can occur while determining the dataset is the data being myopic, which means it does not cover the full scope of the project and is not aligned with the real-world activities the model will perform.
A study2 identified that in the machine learning development community, the majority of the datasets used to train models are reused or borrowed. This creates misalignments in the project objectives and results in an inaccurate finished product.
Challenges like these make it harder for developers to obtain relevant data to train their AI models.
Solutions
To overcome such challenges, the following points can be considered:
- Assign a responsible person: Assign a dedicated team for data collection. A dedicated team will know the project inside out and will be able to choose the right dataset for the job.
- Communicate the goals: Ensure that the team understands and knows the objectives and goals of the project.
- Consider all data collection methods: It can be beneficial to consider all the data collection methods that are being used by data collectors. If prepackaged datasets do not cover the scope of the project, then opt for another data collection method that best suits the project.
- Generate new data through crowdsourcing: If it’s challenging to gather already-made data, you can generate new data through a crowdsourcing platform. Partner with a crowdsourcing data service provider to efficiently generate your own data tailored to the scope of the project.
Here is our guide to finding the right data crowdsourcing platform for your projects.
Here are data collection services based on data types:
- 10+ Image Data Collection Services
- 10+ Speech Data Collection Services
- 7+ Video Data Collection Services & Selection Criteria
2. Data bias problem
Collecting biased data can lead to a biased and erroneous AI/ML model and thus should be avoided. The data collector can also unintentionally transfer this bias into the AI model through the dataset.
For instance, if the dataset used to train a patient referral system does not include male patients or patients with lower income levels, it will provide biased and erroneous outcomes when implemented in a real clinic.
The case of Google’s Gemini
A recent example is Google Gemini’s image generation tool and how it was largely and inappropriately generating image results featuring people of color.3
The figure below illustrates how an Alzheimer’s diagnosis system removed a group of patients because they were not included in the training dataset.
Figure 2. Limited or biased training data leads to erroneous results
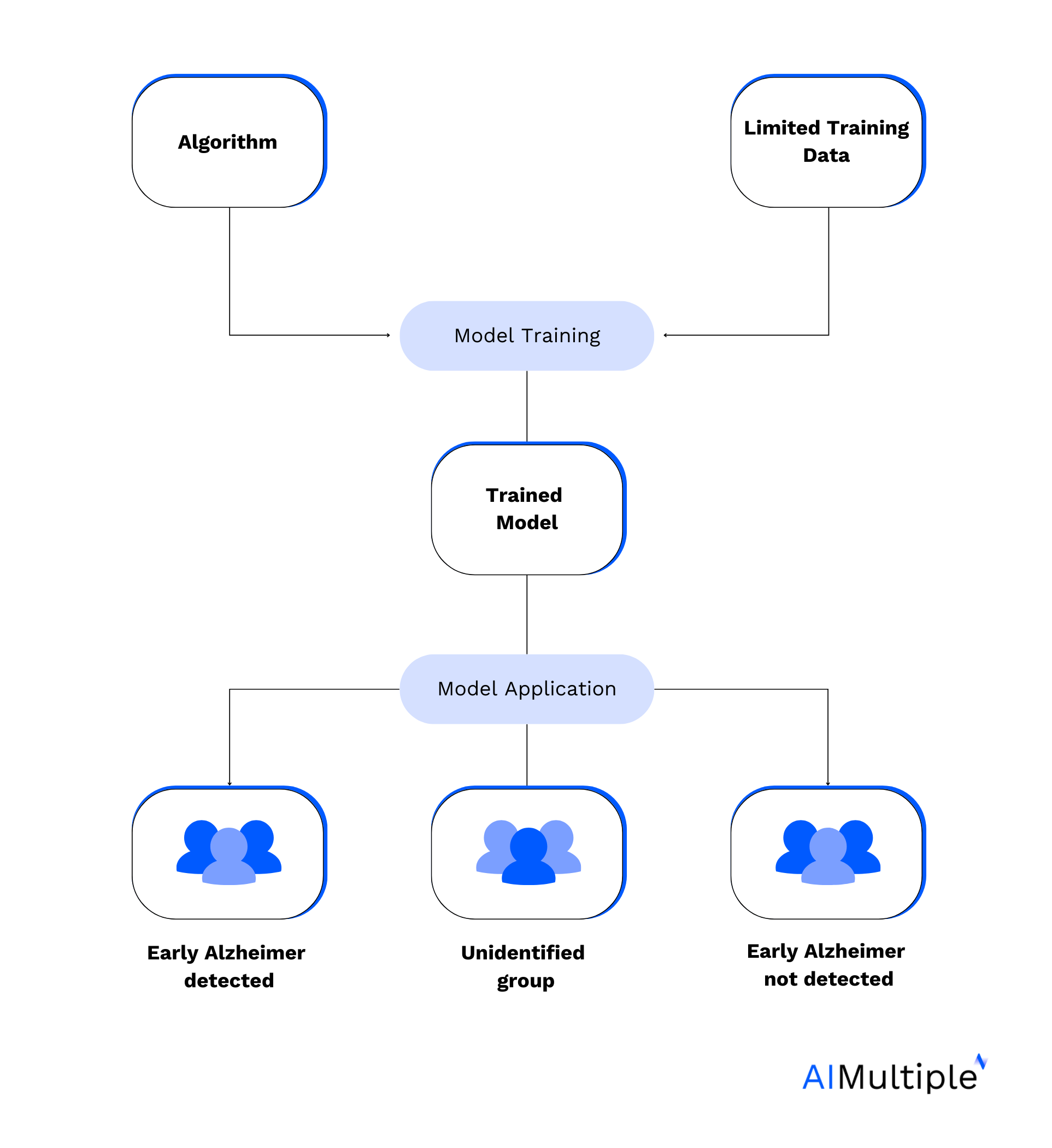
Solutions
The following steps can be taken to overcome data bias while harvesting data:
- Ensure the dataset is complete: Ensure that the dataset is comprehensive and all-inclusive. For instance, a quality inspection system must be trained with data on both defective and working items.
- Ensure the collection team is diverse: Ensure that the data collectors for data collection and revision include people from diverse backgrounds. The dataset must represent the total population on which the AI/ML system will be deployed on.
- Leverage crowdsourcing: Utilize crowdsourcing to expand the range of the data since it offers fast access to large amounts of human-generated data. While recruiting the data collectors, you can check the geographic and demographic data, such as country, educational background, ethnicity, language proficiency, employment, and other relevant factors.
3. Data quality issues
The challenges do not end just after finding the right data. Ensuring data quality while collecting it can be challenging mainly because the data initially collected can be messy and needs processing to be prepared to train machine learning models. Gartner identifies ensuring data quality as one of the top 3 challenges in AI/ML adoption (Figure 3).
This means that your team will be spending a significant amount of time pre-/post-processing and annotating the data. For instance, after collection, the data may need to be cleaned, reduced, transcribed, etc., and these can be manual and error-prone tasks that require a significant amount of man-hours.
Solutions
To overcome such challenges, the following measures can be added to your quality assurance processes:
- Leverage data preprocessing tools: If your dataset contains data of private nature and is of small-scale, then dedicating an internal team and equipping them with data preprocessing tools can be a suitable solution to ensure data quality.
- Leverage outsourcing or crowdsourcing: You can opt for outsourcing or crowdsourcing with a service provider if you can not afford to dedicate a team to process data for quality issues. You can also use this solution if your data is not of private nature.
- Implement quality assurance practices: After collecting the data, check for missing data, data integrity, data irrelevance, data redundancy, etc.
Figure 3. Top 3 challenges to AI/ML adoption4
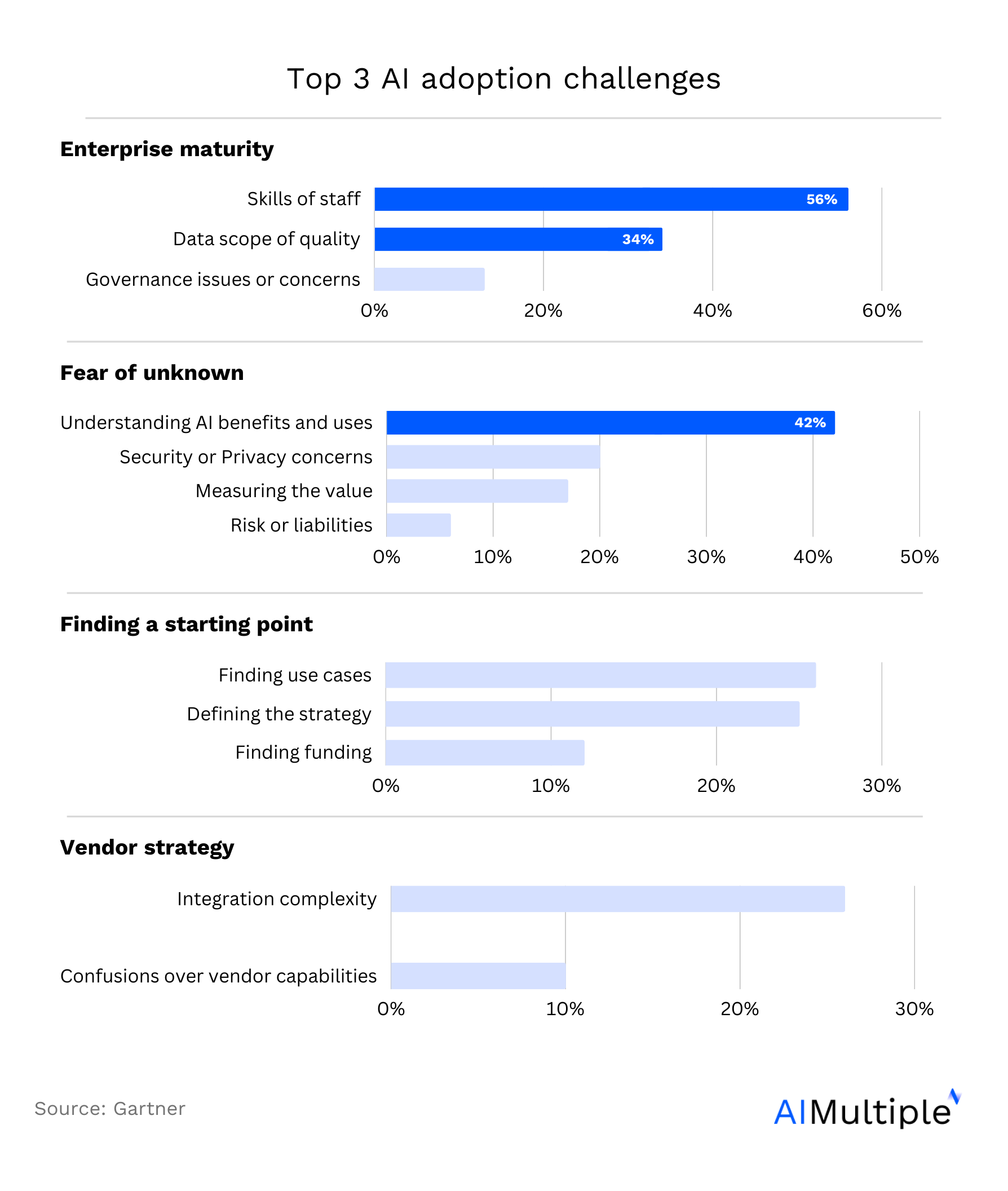
4. Data protection and legal concerns
This section explains some ethical and legal constraints to data collection:
Ensuring data protection
Not all data is readily and publicly available to use. Some data is sensitive in nature and can not be accessed easily, thus making it challenging to collect.
For instance, in order to train a computer vision system for radiology, thousands of medical images are required. This type of data can be expensive to collect and can have various ethical constraints attached.
Avoiding legal issues
Data collection is not as easy as it used to be. As people and government bodies recognize the risks of data exploitation, they make more efforts to regulate data collection and improve data protection.
A recent report by the Linux Foundation identified data ethics and privacy as one of the biggest challenges in AI adoption.
Source: The Linux Foundation Report5
Solutions
In order to avoid these issues, considering the answers to the following questions prior to data collection can be helpful:
- What data will be collected? To answer this, you need to check what type of data is required. For instance, is it biometrics data, such as face images of people, voice data, thumbprint scans, etc.? This can help clarify which ethical and legal factors to consider
- How should legal stipulations (related to the collection of the dataset) be mitigated? Certain data collection methods require unique legal considerations. We recommend studying the country-specific regulations and making sure your current data collection practices align with them.
- How will the data be collected? Different data collection methods have different legal considerations attached to them. For instance, there are certain rules regarding automated data collection systems and tools, such as web scraping, in different countries.
- How will the data be stored? Since cyber threats are rising, it is important to consider where the data will be safest. Will the cloud be a better option or physical hard drives?
- How will the data be used? To answer this question, you need to understand how the data will be used. Who within the organization will have access to the collected data? After figuring this out, you need to communicate this information to the data provider. Answering these questions and clearly explaining them to the participants can make the whole data collection process transparent. It is also important to check the data collection rules from the relevant regulatory body followed in the country in which the data is being collected.
5. Cost concerns
Large datasets require a large number of data collectors. In this case, the costs can pose a barrier. For instance, if a company opts for in-house data collection for an ML project, it will have to perform the following tasks:
- Hire a dedicated team of data collectors
- Ensure the level of diversity and skillsets match the requirements of the project
- Go through onboarding and training for the data collectors
- Acquire all relevant resources for data collection
- Track and manage the progress of data collection tasks from all participants
If the project is of large scale or requires a large dataset, its data collection process can be unaffordable or even financially overwhelming for some businesses. Conversational AI models, for instance, are powered by large language models (LLMs), which contain a vast amount of data, sometimes even multilingual.
Solutions
The following considerations can help overcome this challenge:
- Estimate costs early: Consider data collection costs during the planning phase of the AI/ML development project.
- Select a cost-effective data collection method: If the costs cannot be adjusted in the budget, consider outsourcing the operation since third-party data collection services offer competitive prices.
- Prioritize data requirements: Use prepackaged datasets if the project does not require highly personalized data. These are relatively cheaper to purchase.
6. Avoiding data drift
This is an issue that developers need to tackle on a continuing basis. Data drift can pose the following challenges:
Temporal dynamics
Real-world data often evolves over time due to changing environments, behaviors, or technologies. What’s considered relevant or accurate now might not be in the future. For example, customer preferences might change, new diseases might emerge, or technological advancements might introduce entirely new data patterns.
Maintaining up-to-date models
As data drifts, models trained on older data can become less accurate or entirely obsolete. This necessitates constant monitoring of model performance and regular retraining with fresh data.
Lack of historical data
Sometimes, particularly with emerging phenomena or rapid shifts in context, there’s a lack of historical data that accurately represents current or future states. For instance, during the early stages of the COVID-19 pandemic, there was limited data available on the virus, making it challenging to train predictive modeling systems.
Solutions
Here are some measures to consider:
- Monitoring and detection: Continuously monitor model performance and data statistics. Tools and platforms that offer real-time monitoring can alert developers to significant changes in the input data distribution, signaling potential data drift.
- Regular retraining: Periodically retrain models using fresh data to ensure they remain current and relevant. Automated pipelines can be established to collect, preprocess, and retrain models on new data at regular intervals.
- Adaptive algorithms: Use online learning or active learning techniques, which enable models to adapt on-the-fly as new data becomes available. These approaches can adjust model parameters incrementally, making them more resilient to changing data distributions.
- Work with a data collection partner: If your model needs consistent data collection or new data generation, working with a data collection partner can help streamline this process. The partner can provide on-demand datasets according to the developer’s needs.
You can also check our data-driven list of data collection/harvesting services to find the best option that suits your project.
-
What are the top AI challenges today?
One of the key challenges in AI today revolves around data collection challenges, particularly ensuring data quality and overcoming data bias inherent in the data collection process. Effective data collection requires sophisticated data collection methods that can assure the integrity and quality of data collected. This is crucial as the data initially collected directly impacts the effectiveness of AI models, necessitating consistent data collection practices to ensure that the collected data is representative of the target population. Moreover, the reliance on diverse data sources, including administrative data, demographic data, and external data sources, amplifies the complexity of maintaining data quality and integrity.
Another significant challenge lies in the ethical and methodological considerations of collecting data, particularly sensitive information. Data protection measures and quality assurance processes must be rigorously applied to safeguard the privacy and security of information gathered, especially when dealing with customer data or conducting qualitative research such as focus groups and interviews. The data collection instrument must be meticulously designed to minimize missing data and ensure data comparability, which is critical for the accurate analysis and application of AI. Furthermore, the ever-evolving landscape of AI demands continuous review of current data collection practices and the adoption of innovative collection methods to adapt to the changing needs and enhance the efficiency and effectiveness of data-driven decision-making in core business functions.
For more on AI challenges. -
Why is data important for AI?
Data collection is foundational for AI, as it provides the raw material that fuels AI systems and algorithms. Effective data collection methods ensure the acquisition of high-quality data, which is crucial for training AI models accurately. Sophisticated data collection systems and consistent collection practices are necessary to gather a diverse range of data, including demographic information and data from external sources, ensuring the AI systems can understand and interact with the complexities of the real world. Overcoming data bias through careful data collection practices and ensuring data integrity through quality assurance processes are essential to build AI systems that are reliable and fair.
Moreover, the data collection process directly influences the performance and applicability of AI in various domains, from customer service to healthcare.Collecting data through diverse methods, such as qualitative interviews and surveys, and ensuring the availability of comprehensive data collections, allows AI to gain insights into specific challenges and provide effective services. Data scientists rely on the data initially collected to conduct in-depth data analysis, guiding AI models to make informed decisions that cater to internal business needs and enhance service delivery, demonstrating the critical role of data collection in the advancement of AI technology.
Further reading
- Top 6 Data Collection Best Practices
- AI Data Collection: Quick Guide, Challenges & Top 4 Methods
- Data Collection Automation: Pros, Cons, & 3 Methods
- Crowdsourcing Platforms Comparison & Selection Guide
If you need help finding a vendor or have any questions, feel free to contact us:
External resources
- 1. Statista (2023). Artificial Intelligence: in-depth market analysis. Statista. Accessed: 16/August/2023
- 2. Koch, B. et al. (2021) Reduced, reused and recycled: The life of a dataset in machine learning research. Cornell University. Accessed: 16/August/2023.
- 3. Why Google’s AI tool was slammed for showing images of people of colour. Aljazeera. Accessed: 25/March/2024.
- 4. Goasduff, Laurence. (2019). 3 Barriers to AI Adoption. Gartner. Accessed. 16/August/2023.
- 5. Ibrahim Haddad & Dr. Seth Dobrin. (2022). Artificial Intelligence and Data in Open Source. The Linux Foundation & IBM. Accessed: 16/August/2023.

Comments
Your email address will not be published. All fields are required.