Optimal decisions require high-quality data. To achieve and sustain high data quality, companies must implement effective data quality assurance procedures. See what data quality assurance is, why it is essential, and the best practices for ensuring data quality.
What is data quality assurance?
Data quality assurance is the process of identifying and removing anomalies through data profiling, eliminating obsolete information, and performing data cleaning. Throughout the lifecycle of data, it is vulnerable to distortion due to the influence of people and other external factors. To protect its value, it is important to have an enterprise-wide data quality assurance strategy. Such a strategy encompasses both corporate governance measures and technical interventions.
Why is data quality assurance important now?
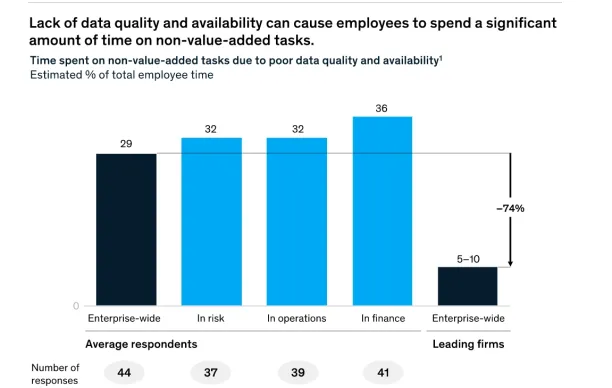
We rely on AI/ML models to gain insights and make predictions about the future. Data quality is directly related to the effectiveness of AI/ML models, as high-quality data means that knowledge about the past is less biased. Consequently, this leads to better forecasts.
As the image above suggests, low-quality data or data scarcity leads workers to spend more effort on tasks that do not add value. This is because, without AI/ML models, every task must be done manually, regardless of its yield. So, ensuring data quality is of great importance in guaranteeing the efficiency of business operations.
What are the best practices for ensuring data quality?
Ensuring data quality requires the efforts of both management and IT technicians. The following list includes some important practices:
- Enterprise data framework: By implementing a data quality management framework within the company, which tracks and monitors the company’s data strategy, the organization can achieve data quality. Data quality management generates data quality rules that are compatible with machine learning (ML) data governance, ensuring the suitability of data used for analysis and decision-making.
- Relevance: The data should be interpretable. This means that the company has appropriate data processing methods, the data format is interpretable by the company’s software, and the legal conditions permit the company to use such data.
- Accuracy: Ensuring the accuracy of the data by techniques like data filtering and outlier detection.
- Consistency of data: By checking internal and external validity of the data, you can ensure consistency.
- Timeliness: The more up-to-date the data, the more precise the calculations.
- Compliance: It is essential to verify whether the data used complies with relevant legal obligations.
1. Enterprise data framework
Data is an asset and a strategic tool for companies. Therefore, it is reasonable for companies to implement a data quality management framework that prioritizes the quality and security of data sustainably. Data quality and security department might rise above the three pillars as follows:
- Central data management office: This office determines the overall strategy for data monitoring and management. The office also supports the underlying teams with the necessary budget and tools to interpret the data.
- Domain leadership: Responsible for executing tasks determined by the Central Data Management Office.
- Data council: A platform that enables the necessary communication between the divisional leaders and the central data management office to take the enterprise data strategy to the implementation level.
2. Relevance
When importing data, it is essential to determine whether the data is relevant to the business problem the company is trying to solve. If the data originates from third parties, it must be ensured that the data is interpretable and accurate. This is because the format of the imported data is not always interpretable by the company’s software.
- Ensure data relevance: Only import data that directly addresses your business problems.
- Confirm compatibility: Verify that the data format is compatible with your company’s software and meets legal conditions for use.
3. Accuracy & completeness
To assess data completeness and healthy data distribution, companies can use some statistical tools. For example, a non-response rate of less than 2% indicates fairly complete data. Data filtering is another important task to ensure data completeness. Due to recording errors, the dataset may contain values that are impossible to observe in reality. For example, the age of a customer could be given as 572. Such variables must be cleaned.
The second step to ensure data accuracy is to identify outliers using distribution models. Then the analysis can be performed taking the outliers into account. In some cases, eliminating outliers can be beneficial for ensuring data quality. However, it is important to note that such outliers may be valuable depending on the task.
- Data filtering and cleaning: Remove or correct anomalies such as impossible values (e.g., a customer age of 572).
- Outlier detection: Utilize statistical models to identify and appropriately handle outliers, which may require adjustment or removal depending on the analysis.
4. Consistency of data
It is essential to verify both the internal and external consistency of the data to determine whether the data is insightful or not.
If data is stored in multiple databases, data lakes, or warehouses, you must ensure consistency to maintain uniformity of the information. To assess internal consistency, companies can utilize statistical values such as the discrepancy rate and the kappa statistic, which evaluate the internal consistency of the data. For example, a kappa value between 0.8 and 1 indicates significantly consistent data, while values between 0.4 and 0 indicate untrustworthy data.
Checking external consistency requires literature searches. If other researchers report similar results with your data interpretation, it can be said that the data are externally consistent.
- Internal consistency: Regularly verify uniformity across different databases or data warehouses using statistical measures like discrepancy rates and the kappa statistic.
- External consistency: Validate your findings with external research to ensure your data interpretations align with industry benchmarks.
5. Timeliness
Business decisions concern the future. To better predict the future, data engineers prefer data that contains current trends of the research topic. In this context, it is important that the data is up to date. When data is imported from third parties, it can be difficult to ensure that the data is current. In this regard, an agreement that provides for live data flow would be beneficial. Versioning data can also be useful for companies to compare trends from the past with the present.
- Up-to-date data: Prioritize current data that reflects recent trends to support future-oriented decision-making.
- Live data flow & versioning: Consider agreements for live data feeds and implement data versioning to track changes over time.
6. Compliance & security
Legal hurdles can be problematic. Therefore, the company must ensure that the interpretation of the imported data will not result in legal investigations that could harm the company. Data center automation can also help companies to comply with data regulations. By integrating specific government APIs, these tools can track and respond to regulatory changes.
- Legal compliance: Ensure your data handling processes meet regulatory standards such as GDPR or HIPAA.
- Automate data center processes: Utilize automation and government API integrations to stay compliant with regulatory changes and mitigate legal risks.
Case Studies
1. Air France–KLM
Challenge
Air France–KLM faced increasing regulatory pressures around customer data privacy (e.g., GDPR) and needed to improve the accuracy and integrity of its passenger data records.
Solution
They implemented Talend Data Quality to clean, standardize, and validate customer profiles. The system flagged duplicates, corrected formatting issues, and ensured legal compliance.
This improved the consistency of customer communications and ensured full compliance with evolving data regulations, ultimately supporting a smoother and more trustworthy customer experience. 1
2. Procter & Gamble (P&G)
Challenge
P&G managed master data across 48 different SAP systems globally, leading to inconsistent product, vendor, and customer data. This inconsistency disrupted reporting and increased operational complexity.
Solution
The company developed a centralized data governance framework with strong master data management protocols. Using a unified data quality platform, they introduced validation rules, cleansing processes, and metadata tracking.
The initiative led to significant improvements in data consistency and control, resulting in reduced redundancy and enhanced analytics reliability at scale. 2
3. Quantzig – Data Quality Management
Challenge
Businesses face incomplete data across their various departments. This fragmentation affected reporting accuracy and slowed down decision-making processes.
Solution
Quantzig implemented a structured data quality enhancement solution. The approach included automated profiling, rule-based validation, and enrichment using machine learning.
The business standardized data formats, improved accuracy using outlier detection, and ensured consistency across platforms through real-time dashboards. This holistic framework improved decision-making speed and enabled higher operational efficiency.
Top data quality assurance tools
1. Talend Data Quality
- Data profiling & cleansing: Provides comprehensive tools for identifying and correcting data errors.
- Integration: Seamlessly integrates with Talend’s broader data integration suite, enabling smooth data flow across systems.
2. Informatica Data Quality
- Comprehensive data cleansing: Offers advanced features for data standardization, matching, and cleansing.
- Scalability: Designed to handle large volumes of data, making it suitable for enterprise-level applications.
3. IBM InfoSphere QualityStage
- Data consolidation: Facilitates the cleaning, matching, and consolidation of data from multiple sources.
- Advanced algorithms: Leverages sophisticated algorithms to improve data accuracy and reliability.
You can read our article on training data platforms that includes a list of the top vendors.
If you need assistance in selecting data quality assurance vendors, we can help:
Comments
Your email address will not be published. All fields are required.