Figure 1. Interest in data governance.1
Data governance is a crucial aspect of the management of data within an organization. With the rise of machine learning (ML) and artificial intelligence (AI) applications, it has become even more critical for businesses (Figure 1). This is because data governance strategies can improve:
- Data quality
- Data security
- Data integrity
Nevertheless, machine learning data governance is not frequently searched on Google and as a result, many business leaders may not know about the recent developments in machine learning data governance (Figure 2). This article will explore the importance of data governance in machine learning to inform business leaders on its:
- Key principles
- Benefits
- Use Cases
- Best practices
- Future of data governance to establish a robust data governance framework
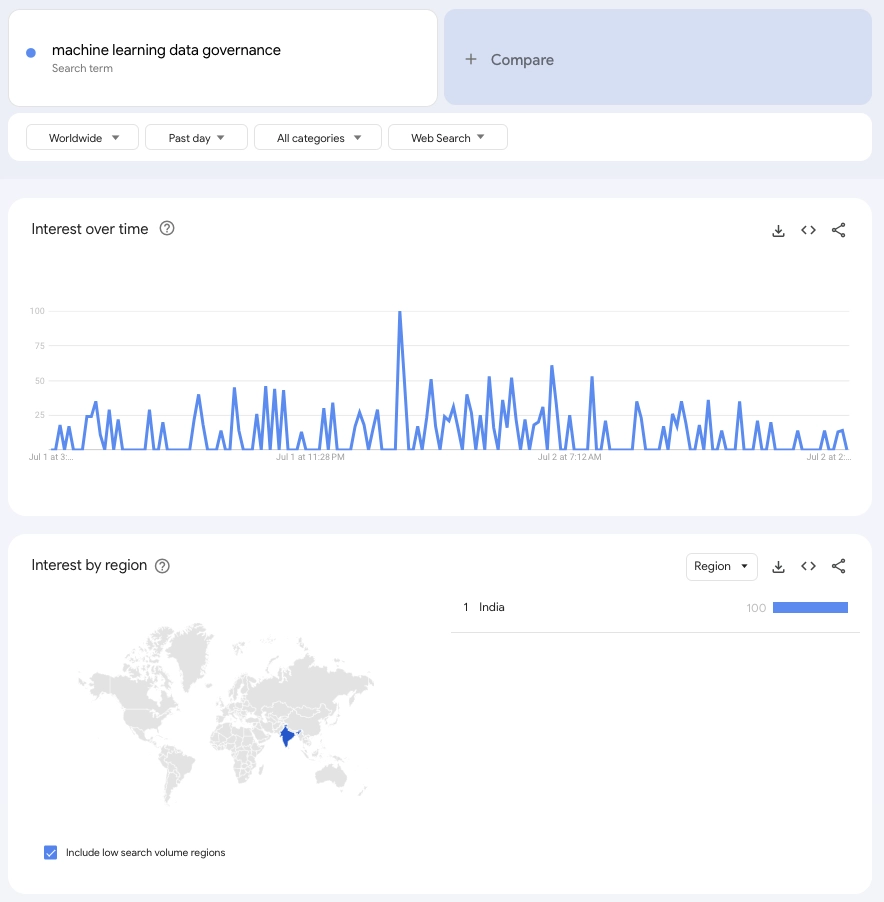
Figure 2. Interest in machine learning data governance.2
What is machine learning data governance?
Machine learning data governance is the set of policies, processes, and technologies that ensure the proper management and use of data in machine learning applications. It involves:
- Data collection
- Data storage
- Data processing
- Data sharing in a controlled way to:
Key principles of machine learning data governance
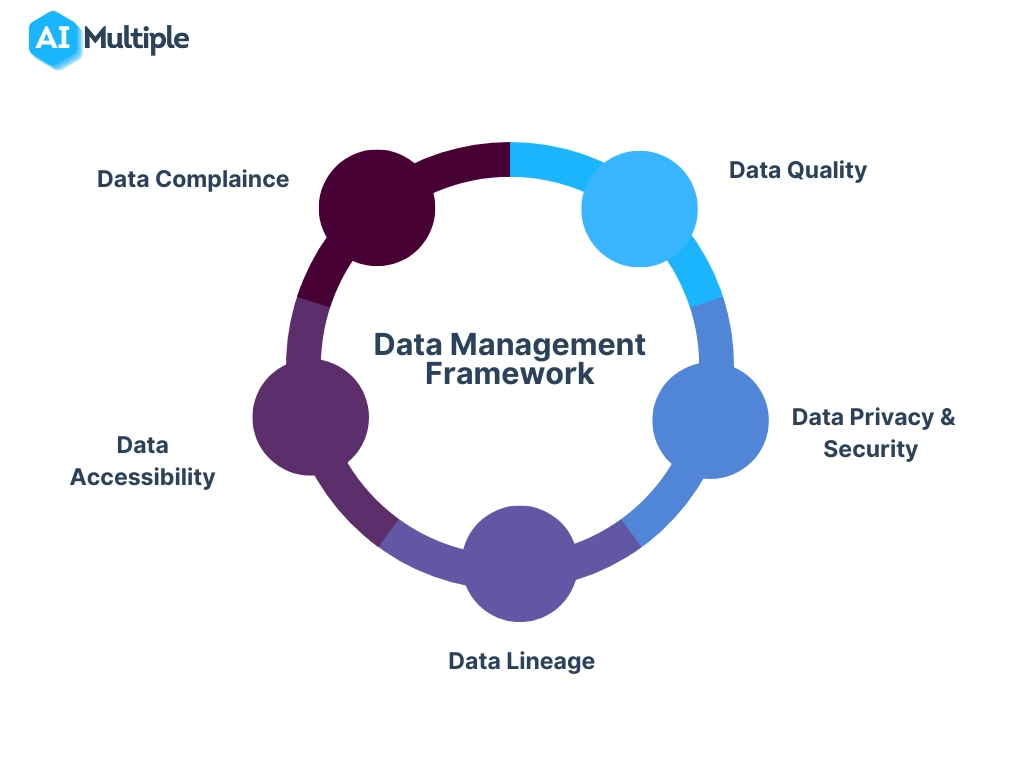
Figure 3. Data management framework.
1. Data quality
It is critical for producing reliable and meaningful results to ensure that the data used for machine learning applications is:
- Accurate
- Complete
- Consistent
For example, the use of high-quality data for validation, data cleansing, and data enrichment processes can aid in the maintenance of high data quality standards.
2. Data privacy and security
Protecting access to sensitive data and adhering to data protection regulations such as GDPR and CCPA can be critical. Encryption, access control, and regular audits of systems can all help to secure data and protect privacy.
3. Data lineage
Tracking the origin and transformations of data as it moves through the ML pipeline can be essential for understanding the impact of data on the model’s performance and for maintaining the traceability of data pipelines. Data lineage is particularly important in machine learning applications, as it allows organizations to identify data sources and data transformations that contribute to a model outcome.
4. Data accessibility
It is critical for the smooth operation of ML applications to ensure that data is easily accessible to authorized system users. Data accessibility can be improved by establishing clear data access policies and implementing efficient data storage and data model solutions.
5. Data compliance
Compliance with relevant industry regulations and ethical guidelines such as Health Insurance Portability and Accountability Act (HIPAA) is critical for avoiding legal and ethical issues related to the use of data in ML applications.
5 Benefits of machine learning data governance
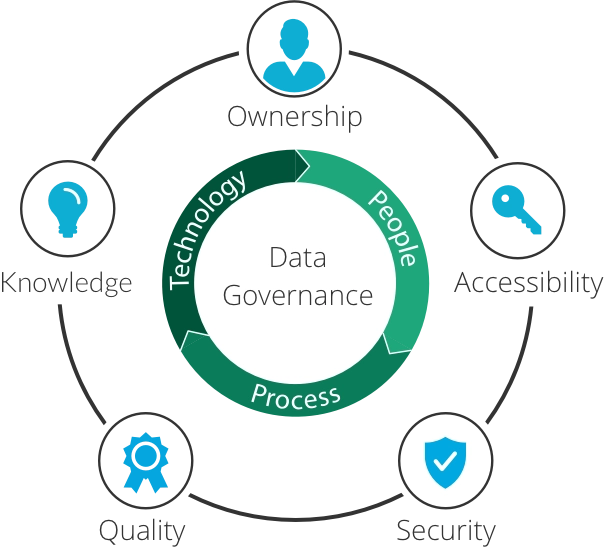
Figure 4. Data governance benefits.3
1. Improved model performance
High-quality, well-governed data can lead to more accurate and reliable machine learning models, which in turn, drive better decision-making and business outcomes.
2. Regulatory compliance
Robust data governance helps organizations meet the requirements of data protection regulations. This can reduce the risk of non-compliance penalties and reputational damage.
3. Enhanced trust and transparency
Implementing data governance policies and practices demonstrate an organization’s commitment to ethical data usage, fostering trust among customers, partners, and regulators.
4. Reduced data-related risks
Organizations can reduce risks associated with data breaches, data misuse, and biased model outcomes by managing data quality, privacy, data definitions, and data security.
5. Increased collaboration and efficiency
A well-defined data governance framework fosters collaboration among data scientists, engineers, and other stakeholders. This can speed up the development and deployment of machine-learning applications.
Use cases of machine learning data governance
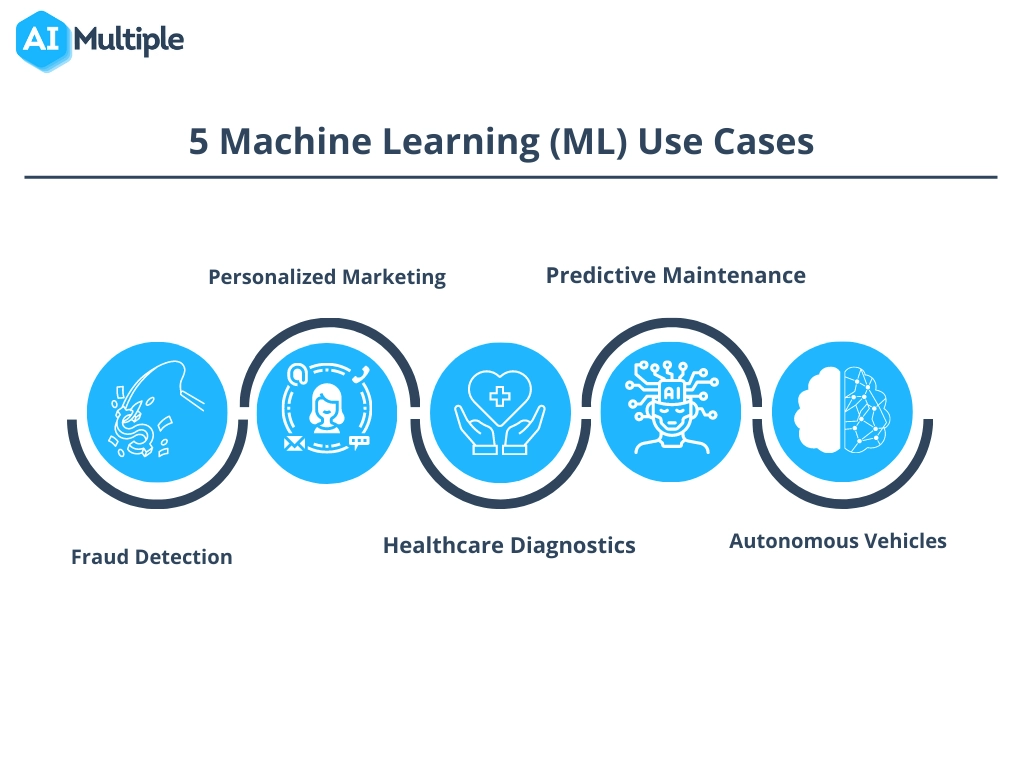
Figure 5. Machine learning use cases.
1. Fraud detection
Financial institutions use machine learning to detect fraudulent activities. Data governance can ensure that the data feeding to these algorithms is accurate, complete, and secure.
2. Personalized marketing
Retailers and e-commerce companies leverage machine learning for personalized marketing campaigns. Effective data governance can ensure customer data privacy while delivering relevant content.
3. Healthcare diagnostics
Machine learning algorithms are increasingly used in medical diagnostics. Data governance can be crucial for maintaining data quality, privacy, and regulatory compliance in healthcare applications.
4. Predictive maintenance
Manufacturing companies can use machine learning to predict equipment failures and optimize maintenance schedules. Data governance can ensure the reliability of the sensor data and other IoT inputs used in these applications.
5. Autonomous vehicles
Data governance is critical to ensuring the quality, accuracy, and security of the massive amounts of data used in the development and operation of self-driving cars.
Best practices for implementing machine learning data governance
1. Develop a data governance strategy
Creating a data governance strategy that defines your organization and data stewards goals, roles, responsibilities, and processes can help provide a clear roadmap for effective data management in machine learning applications.
2. Establish data ownership and accountability
Clearly defining data ownership and assigning responsibilities for data quality, privacy, and compliance can aid in the effective implementation of data governance policies.
3. Implement data catalogs and metadata management
Creating a data catalog and maintaining metadata about datasets used in machine learning applications can aid in:
- Understanding data lineage
- Improving data discoverability
- Preserving data quality
4. Adopt data privacy by design
Integrating data privacy and security considerations into the due process and design of ML applications and processes can aid in the proactive management of potential risks and compliance with data protection regulations.
5. Automate data governance processes
Data governance tasks such as data validation, cleansing, and enrichment can be automated to improve efficiency and maintain high data quality standards.
6. Monitor and audit
Monitoring and auditing data governance processes on a regular basis can help:
- Identify potential issues
- Maintain data quality
- Ensure compliance with applicable regulations
Using data fabric tools can be especially useful in monitoring and auditing data governance.
Future of data governance in machine learning
1. AI-Driven data governance
As machine learning technology advances, we can anticipate the emergence of AI-driven data governance solutions. These solutions will automate and optimize data governance processes, allowing organizations to more efficiently manage increasingly complex data ecosystems.
2. Evolving regulatory landscape
Governments and regulators can continue to develop new policies and guidelines as more organizations adopt machine learning and AI. To remain compliant and maintain stakeholder trust, many organizations will need to adapt their data governance strategies.
3. Data privacy and ethics
The increasing importance of data privacy and ethical considerations in machine learning can highlight the need for strong data governance frameworks. To maintain a competitive advantage, organizations will need to adopt transparent, accountable, and fair data usage practices.
4. Data democratization
Effective data governance will be critical for maintaining data quality and security. Effective data governance can empower employees to leverage data-driven insights when organizations increasingly democratize access to data and analytics tools.
5. Integration of data governance and model governance
The integration of data governance and model governance can become increasingly important as machine learning models become more complex and widespread. This can ensure that both the data and the models used are managed effectively.
FAQ
Why does data governance affect the cost of machine learning?
Model performance is tightly linked to data quality. Poor governance leads to inefficiencies such as repeated labeling and unnecessary cloud compute usage. These can affect the cost of machine learning initiatives.
Comments
Your email address will not be published. All fields are required.