As organizations recover from pandemic disruptions, leveraging AI in logistics operations can strengthen supply chains and deliver significant benefits, such as reducing inventory by almost 30%, logistics costs by 20%, and procurement spend by 15%.1
Success in logistics AI requires improving processes, simplifying technology, and enhancing team experiences. Check out to explore top AI use cases with real-life examples in the logistics industry and how they improve logistics operations.
Logistics planning
Logistics requires significant planning that requires coordinating suppliers, customers, and different units within the company. Machine learning solutions can facilitate planning activities as they are good at dealing with scenario analysis and numerical analytics, both of which are crucial for planning.
1. Demand forecasting
Accurate demand forecasting is at the heart of efficient logistics planning. Traditional methods like ARIMA (AutoRegressive Integrated Moving Average) and exponential smoothing often fall short when dealing with high-variability or real-time data. AI in logistics introduces AI algorithms that integrate real-time feeds with historical data to forecast demand more precisely. These algorithms account for seasonal patterns, promotional impacts, shipping industry trends, and regional consumption behavior to produce dynamic and context-aware forecasts.
By leveraging predictive planning powered by AI systems, logistics companies can:
- Optimize transportation routes by deploying the most efficient route for deliveries. With access to real-time traffic data and historical delivery outcomes, route planning becomes far more responsive to on-the-ground conditions. This leads to a notable decrease in fuel consumption, delivery times, and carbon emissions, while also improving the management of delivery routes.
- Minimize inventory levels at local distribution hubs by aligning stock quantities with future demand. Lower inventory management costs translate directly to reduced operating costs, especially as holding inventory ties up capital that could otherwise be invested more strategically.
- Align workforce deployment more accurately through advanced predictive analytics. By anticipating logistics operations volume in advance, companies can reduce overtime expenses and avoid under- or over-staffing.
- Enhance customer satisfaction by reducing the frequency of stock-outs or delays. With more accurate predictions, companies can better meet customer demand and offer better customer service, a key differentiator in today’s competitive logistics landscape.
2. Supply planning
Supply planning is a critical function within the broader supply chain management ecosystem, encompassing the coordination of materials, production, and distribution to meet predicted demand. In traditional logistics operations, supply planning is often reactive, relying on periodic updates and rigid parameters. However, the integration of artificial intelligence, particularly AI systems and machine learning algorithms, has made it possible to evolve toward a more adaptive, data-driven model.
AI in logistics empowers organizations to analyze data from a diverse set of sources—including historical sales data, real-time demand signals, customer data, and transportation routes—to keep supply plans continuously aligned with actual demand. This transition from static to dynamic supply planning enhances the responsiveness and flexibility of the entire logistics sector, addressing supply chain challenges in real-time.
Dynamic adjustment of supply parameters
By using predictive analytics and AI technology, logistics companies can dynamically adjust parameters such as reorder points, safety stock levels, and production schedules. This is especially valuable in managing highly variable demand scenarios, seasonal fluctuations, and sudden changes in transportation volumes or production capacity. Instead of relying on pre-set rules or manual data entry, self-learning digital systems update planning rules autonomously, leading to more precise and timely decision-making.
For example:
- When AI algorithms detect a surge in forecasted demand through inputs like real-time traffic data, historical data, or changing market trends, they can trigger upstream adjustments in material procurement and production schedules, preventing bottlenecks and delays.
- Conversely, if customer demand drops unexpectedly, AI can recommend a temporary reduction in replenishment volumes, reducing the risk of overproduction and minimizing inventory levels that contribute to excess holding costs and waste.
Waste reduction
Dynamic supply chain planning powered by AI implementation not only improves alignment between supply and demand but also drives significant efficiency improvements across the value chain:
- Reduced operational costs: Organizations can operate with leaner inventories, cutting down on inventory management expenses and freeing capital for other investments.
- Lower carbon emissions: Efficient planning means fewer unnecessary shipments and better-loaded delivery vehicles, which directly supports more sustainable practices in the logistics industry.
- Improved operational efficiency: AI-driven tools improve synchronization between logistics processes, reducing idle time, optimizing production runs, and enabling the identification of the most efficient route for the delivery of raw materials or finished goods.
- Fewer manual processes: By implementing AI technology, companies significantly reduce dependency on human expertise for routine analysis, allowing staff to focus on more strategic roles such as supplier collaboration or data security and compliance.
Enhancing supply chain visibility
With AI-powered logistics, companies gain better supply chain visibility, ensuring that any disruptions—whether related to delays in transportation, raw material shortages, or supplier reliability—are quickly identified and mitigated. These systems use machine learning to correlate a wide range of data points, allowing for more agile responses and sustained service levels even under stress.
Moreover, generative AI supply chain tools can further augment planning by creating simulations of alternative supply scenarios, enabling planners to evaluate trade-offs between cost, speed, and risk without physically executing changes. This kind of predictive planning supports a more resilient supply chain, capable of navigating the volatility that defines the modern logistics landscape.
Automated Warehousing
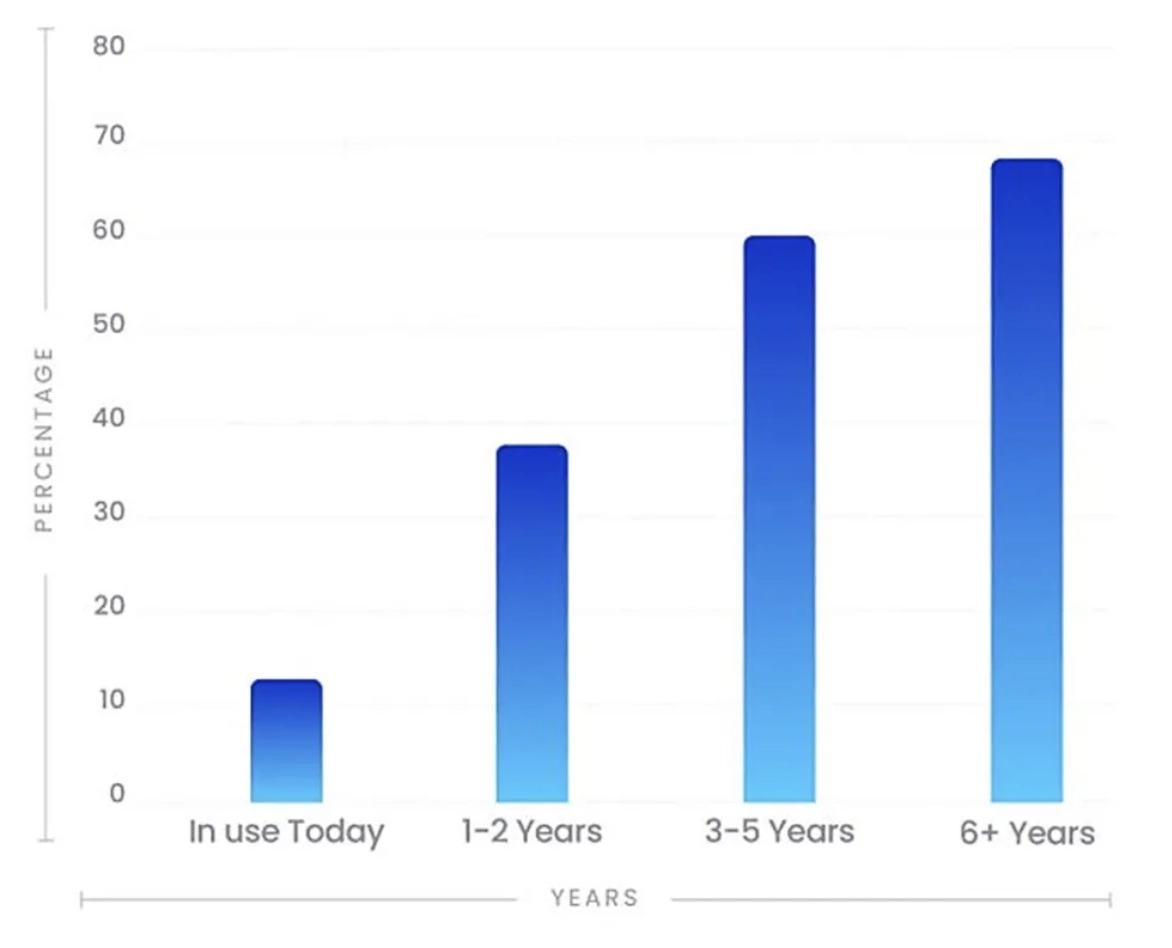
Figure 1: The MHI annual industry report projects that by 2026, the adoption of AI-powered warehouse solutions by businesses will reach 60+% % as compared to 2020.2
3. Warehouse robots
Warehouse robots are another AI technology that is being invested in heavily to enhance businesses’ supply chain management.
These robots can manage operations by automating tasks such as picking, packing, sorting, and inventory management, resulting in faster order processing, improved accuracy, and lower labor costs. By leveraging advanced AI algorithms, warehouse robots can adapt to dynamic environments, optimize workflows, and ensure coordination with other automated systems.
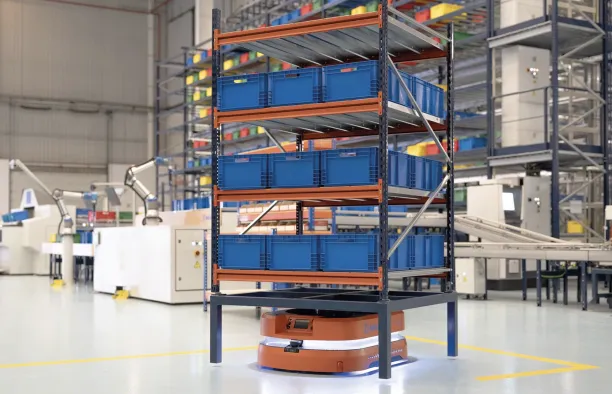
Figure 2: An example of autonomous warehouse robots helping transport shelving units.3
Real-life example
The retail giant Amazon has deployed over 200,000 robots in its warehouses to enhance operational efficiency. These robots work alongside human employees, handling tasks like picking, sorting, and transporting goods.
By integrating robotics, Amazon has improved efficiency, reduced costs, and enhanced its ability to manage high volumes of orders, especially during peak seasons. This large-scale automation demonstrates the transformative potential of robotics in modern warehousing.4
4. Damage detection & visual inspection
In the logistics industry, damaged goods not only drive up operating costs but also erode customer satisfaction, leading to potential churn and reputational harm. Traditional inspection methods relying on manual processes are time-consuming and prone to human error, especially as transportation volumes and order frequency rise.
By implementing AI technology, particularly computer vision, logistics companies can automate visual inspections within warehouse management and packaging workflows. These machine learning and data science-driven tools analyze thousands of images in real time to detect anomalies, flagging issues that might escape human notice.
Using AI in logistics for damage detection, logistics managers can:
- Precisely identify the type and size of damage by analyzing visual patterns through machine learning algorithms trained on historical data.
- Prevent escalation by isolating affected items early, reducing waste, and enabling timely rerouting or replacement.
- Generate actionable insights by combining customer data, historical sales data, and product condition images to improve predictive planning and packaging methods.
This use of AI-powered logistics improves supply chain visibility, enhances logistics operations, and ensures a higher quality standard across the supply chain, all while contributing to better customer satisfaction and reduced operational costs.
Real-life example
Google Cloud Visual Inspection AI automates quality control by detecting product defects using advanced AI and computer vision.
The solution runs autonomously on-premises or in the cloud, supporting ultra-high resolution images for precise defect detection. Customers report up to 10 times accuracy improvements over traditional machine learning (ML) and require significantly fewer labeled images to train models.
Beyond anomaly detection, it identifies, classifies, and locates multiple defects in a single image, enabling automated follow-up tasks.5
5. Predictive maintenance
Predictive maintenance involves predicting potential machine failures in a factory by analyzing real-time data collected from IoT sensors on machines.
Machine learning-powered analytics tools enhance predictive analytics and identify patterns in sensor data, enabling technicians to take action before failure occurs.
See the video below for an example of DINGO, a global leader in Predictive Maintenance solutions, partnered with QUT to enhance its predictive maintenance capabilities through machine learning, achieving impactful business outcomes within 2–3 months.
Autonomous Things
Autonomous things are devices that work without human interaction with the help of AI. Autonomous things include self-driving vehicles, drones, and robotics. We can expect to see an increase in autonomous devices in the logistics industry, given the industry’s suitability for AI applications.
6. Self-driving vehicles
Self-driving cars have the potential to transform logistics by decreasing heavy dependence on human drivers.
Technologies such as platooning support drivers’ health and safety while reducing carbon emissions and fuel usage of vehicles.
Major companies like Tesla, Google, and Mercedes-Benz are investing heavily in the concept of autonomous vehicles; it is only a matter of time before autonomous trucks are seen on roads around the world. However, according to BCG estimations, only around 10% of light trucks will drive autonomously by 2030.6
Real-life example
The Tesla Semi is an all-electric Class 8 truck designed to transform freight transport with its performance, efficiency, and sustainability. It offers a range of up to 500 miles on a single charge and can accelerate from 0 to 60 mph in 20 seconds, fully loaded.
Equipped with advanced safety features like enhanced autopilot and energy-efficient systems, the Semi reduces operational costs and emissions. Its fast-charging capabilities via the Tesla Megacharger network make it an innovative solution for modern logistics.
7. Delivery drones
For logistics purposes, delivery drones are useful machines when businesses need to deliver products to areas where ground transportation is not possible, safe, reliable, or sustainable.
Especially in the healthcare industry, where pharmaceutical products have a short shelf life, delivery drones can help businesses reduce waste costs and prevent investments in costly storage facilities.
See the video below for the “Deliver Future” project, a collaboration between DHL, GIZ on behalf of BMZ, and Wingcopter, that successfully tested the use of drones to supply medicines to isolated areas in eastern Africa.
Analytics
8. Dynamic pricing
Dynamic pricing is a data-driven strategy in which product prices are continuously adjusted in response to fluctuations in demand, supply, competitor pricing, and related product trends. In the fast-paced logistics landscape, where cost structures and customer behavior evolve rapidly, static pricing models can lead to lost revenue opportunities or inefficient resource allocation.
Modern pricing software, powered by machine learning algorithms and AI technology, enables companies to analyze data, including historical sales data, customer data, and competitor benchmarks, in real-time. These AI systems detect patterns across thousands of data points to forecast market movements and execute timely price adjustments.
By leveraging AI in logistics, businesses can:
- Respond to supply chain challenges with real-time pricing changes that reflect shifts in inventory levels, transportation costs, or shipping industry dynamics.
- Utilize predictive analytics to forecast future demand and employ pricing models that optimize both sales volume and profit margins.
- Reduce reliance on manual processes and eliminate lag in response time by allowing self-learning digital systems to make pricing decisions automatically.
The result is improved operational efficiency, better alignment with market trends, and the ability to offer competitive pricing that enhances customer satisfaction while helping to reduce operating costs across the logistics sector.
9. Route optimization / Freight management
AI models help businesses analyze existing routing and track route optimization. Route optimization utilizes shortest-path algorithms in the field of graph analytics to determine the most efficient route for logistics trucks.
Therefore, the business will be able to reduce shipping costs and speed up the shipping process. Route optimizers are also effective tools for reducing a corporation’s carbon footprint.
Real-life example
Valerann’s Smart Road System is an AI-powered traffic management platform designed to enhance safety, efficiency, and connectivity on roads. It collects and analyzes real-time data from a network of smart sensors embedded in road infrastructure, providing critical insights into road conditions, traffic flow, and potential hazards.
This information is delivered to autonomous vehicles, traffic operators, and road users, enabling them to make better decisions and manage transportation systems more proactively. Valerann’s system supports a wide range of applications, including accident prevention, congestion reduction, and optimized traffic control.7
Back office management
Every business unit has back-office tasks, and logistics is no different. For example, there are numerous logistics-related forms, such as a bill of lading, from which structured data must be manually extracted. Most businesses do this manually.
10. Automating document processing
Invoice, bill of lading, and rate sheet documents facilitate communication between buyers, suppliers, and logistics service providers.
Document automation technologies can be utilized to enhance the efficiency of document processing by automating data input, error reconciliation, and document preparation.
11. Automating other manual office tasks
Hyperautomation, also referred to as intelligent business process automation, means using a combination of AI, robotic process automation (RPA), process mining, and other technologies to automate processes in an end-to-end manner. With these technologies, businesses can automate several back-office tasks, such as
- Scheduling and tracking: AI systems can schedule transportation, organize cargo pipelines, assign and manage employees to specific stations, and track packages within the warehouse.
- Report generation: Logistics companies can utilize RPA tools to automatically generate regular reports that are required to inform managers and ensure everyone in the company is aligned. RPA solutions can easily auto-generate reports, analyze their contents, and, based on the contents, email them to relevant stakeholders.
- Email processing: Based on the contents in auto-generated reports, RPA bots can analyze the content and send emails to relevant stakeholders.
12. Customer service
Customer service plays a crucial role in logistics companies, as customers often contact them for any issues they experience with delivery. Customer service chatbots are capable of handling low-to-medium call center tasks such as:
- Requesting a delivery
- Amending an order
- Tracking shipment
- Responding to an FAQ
Chatbots are also valuable tools for analyzing customer experience; chatbot analytics metrics enable businesses to gain a deeper understanding of their customers, allowing them to enhance the customer journey they deliver.
Real-life example
Streebo’s logistics chatbot is a Generative AI-powered solution tailored for the logistics and delivery industry. It helps automate key business processes while enhancing customer engagement and support.
The chatbot works across multiple channels, including web, mobile apps, WhatsApp, Facebook Messenger, email, and SMS. This omnichannel capability ensures that customers can interact with the business wherever it’s most convenient for them.
It also supports over 38 languages, making it accessible to a global user base. The underlying AI technology integrates with powerful NLP engines like IBM Watson, Google Dialogflow, Amazon Lex, and Microsoft Azure’s CLU, enabling smart and natural conversations.
Out of the box, it comes pre-trained for logistics-specific scenarios. Businesses can quickly utilize it for tasks such as shipment tracking, order booking and modification, delivery scheduling, and basic customer service inquiries.
On the operational side, it provides real-time inventory visibility, assists with stock management, and supports route optimization to reduce delivery time and costs.8
Real-life example
Shipping giant CMA CGM and French AI startup Mistral AI have formed a five-year, €100 million partnership aimed at enhancing customer service in shipping and logistics, as well as improving fact-checking capabilities in CMA CGM’s French media outlets like BFM TV. This initiative is part of CMA CGM’s broader AI investment strategy, which now totals €500 million.
The collaboration aims to reduce response times for customer service representatives, who handle over one million emails weekly, with implementations expected within 6 to 12 months.
Additionally, the partnership underscores a commitment to French innovation amid global trade tensions and competition from low-cost Chinese AI models.9
Check out ChatGPT use cases in customer service to learn more about AI applications in customer service.
Sales & marketing
Sales and marketing activities of logistics service providers can also be enhanced through the use of artificial intelligence. Some applications are:
13. Lead scoring
Lead scoring means enabling sales reps to focus on the right prospects. AI-powered tools can be used to help automatically assign scores to leads based on their profiles, behavior, and interests.
AI-based lead scoring systems utilize machine learning algorithms to quickly process data and accurately determine which leads are most likely to convert into paying customers.
14. Routine marketing
AI can be utilized to assist logistics service providers in automating routine marketing tasks, including email marketing and content creation.
15. Sales and marketing analytics
AI can offer more precise sales and marketing analytics. AI-powered tools can help logistics service providers analyze customer behavior and utilize predictive analytics to better understand what their customers are likely to do next.
AI-enabled systems can also be utilized to monitor market changes, enabling logistics service providers to stay ahead of the competition and make data-driven decisions that result in greater efficiency.
For more AI applications in marketing, check out Generative AI in Marketing: Benefits & Use Cases.
The future of logistics AI operations
According to DHL Freight’s Logistics Trends report,10 AI will be at the core of future logistics operations. It will move beyond basic automation to enable dynamic decision-making, predictive planning, and real-time optimization across supply chains.
As AI systems become more advanced, they will drive greater efficiency, reduce environmental impact through smarter routing and energy use, and help logistics firms respond swiftly to disruptions.
The integration of AI with sustainable technologies and enhanced cybersecurity will define the next era of intelligent, resilient, and eco-conscious logistics.
Conclusion
AI is enhancing logistics by improving key processes, including demand forecasting, supply planning, and route optimization.
For instance, AI algorithms enable companies to predict future demand by combining historical data with real-time inputs, resulting in more effective planning and inventory management. This allows businesses to adjust their supply plans dynamically, reducing waste and inventory costs.
AI also enables real-time adjustments to transportation routes, leading to more efficient deliveries, reduced fuel consumption, and lower carbon emissions.
In warehouses, AI-powered robots handle tasks such as picking and sorting, thereby increasing accuracy and speeding up order fulfillment. Visual inspection systems detect product defects early, improving quality control and reducing waste.
Additionally, AI tools in customer service, like chatbots, automate responses to common queries, freeing up resources while enhancing customer satisfaction. These real-life applications demonstrate how AI is helping logistics companies reduce costs, increase efficiency, and improve service delivery, making operations more responsive and adaptable to changing conditions.
External Links
- 1. Harnessing the power of AI in distribution operations.
- 2. 2025 MHI Annual Industry Report - The Digital Supply Chain Ecosystem.
- 3. Autonomous Mobile Robots (AMRs) - Mecalux.com.
- 4. Amazon now has 200,000 robots working in its warehouses. Robotics & Automation News
- 5. Improve manufacturing quality control with Visual Inspection AI | Google Cloud Blog. Google Cloud
- 6. The Future of Commercial Vehicles. BCG Global
- 7. https://www.valerann.com/
- 8. Logistics Chatbot: Top Use Case Examples and Benefits. STREEBO
- 9. reuters.com.
- 10. Logistics Trends 2025 | DHL Freight. DHL Freight Connections
Comments
Your email address will not be published. All fields are required.