Handwriting Recognition in 2024: In-depth Guide
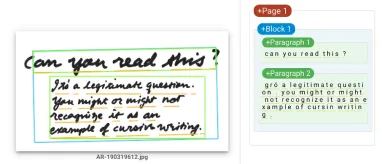
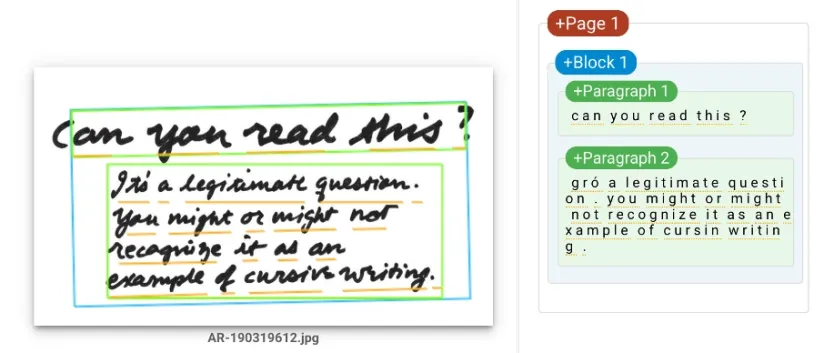
Today, OCR technology provides higher than 99% accuracy with typed characters in high-quality images. However, the diversity in human writing types, spacing differences, and irregularities of handwriting causes less accurate character recognition, as you can see in the featured image. Thus, tools that read handwriting cannot provide the same degree of accuracy that OCR systems offer on typed characters.
However, thanks to the active research in this field, handwriting recognition improves its accuracy by introducing highly sophisticated algorithms designed to solve this problem.
What is handwriting recognition?
Handwriting recognition, also known as handwriting OCR or cursive OCR, is a subfield of OCR technology that translates handwritten letters to corresponding digital text or commands in real-time. To perform this task, these systems benefit from pattern matching to identify various styles of handwritten letters. Wikipedia defines handwriting recognition as:
the ability of a computer to receive and interpret intelligible handwritten input from sources such as paper documents, photographs, touch-screens, and other devices.
What is included in handwriting?
By handwriting, we refer to texts that are written in manuscript form and cursive form. Texts in manuscript style are easier to recognize as the characters are written separately as block letters. However, cursive handwriting involves joined characters as they are written. This aspect necessitates handwriting recognition tools to perceive each separate character correctly and identify them accurately. Below are the examples of manuscript and cursive writing.
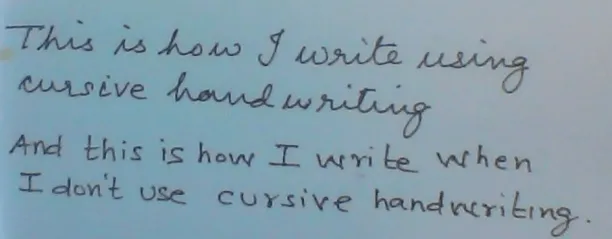
Handwriting on digital screens can be identified by handwriting recognition tools, as well. This kind of handwriting can be tracked as it is written. The software can leverage your dynamic motion to provide more accurate results. Below you can see an example of digital handwriting recognition, provided by Microsoft Azure Ink Recognizer API.
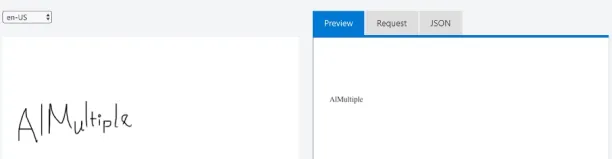
What are the challenges of handwriting recognition?
Even though traditional OCR tools have been in the market since the 70s, there are still not many tools that can handle handwriting recognition. As everyone has their own style of writing, traditional OCR tools cannot perceive everyone’s handwriting. Besides computer vision technology, highly complex deep learning algorithms are required to identify all these variations successfully. Below is a list of challenges that handwriting recognition tools frequently encounter:
- Higher image quality is critical for handwriting recognition, however OCR solutions need to deal with a variety of quality of images:
- Images of handwritten text come at different levels of quality based on the camera used in the process.
- These images also generally feature some form of background image which generates noise for OCR programs and increases processing time.
- These are not issues for computer generated text. They tend to be shared digitally as high quality images with no background noise.
- a variety of individual handwritings, including different styles and different alphabets
- characters might be skewed which makes recognition harder
- neighboring symbols can be connected
There are some approaches to surpass these challenges and improve the handwriting recognition tool’s accuracy:
- Using higher-quality images that are easier for character recognition as inputs
- Removing background using machine learning algorithms or improved photography practices
- Developing more advanced recognition algorithms to manage handwriting OCR tasks more accurately
- Designing documents in an OCR-friendly way.
How to design your documents for accurate handwriting recognition?
There are a variety of factors to consider while designing documents. The most important one is the data to be captured from documents. As there are different ways to represent the same type of data, you need to consider the speed, accuracy, and user-friendliness of each option while constructing your document.
Leverage segmentation techniques
The characters written on the document should be separated enough and clearly for higher accuracy levels. To ensure that, businesses can make use of segmentation methods, which you can see below.
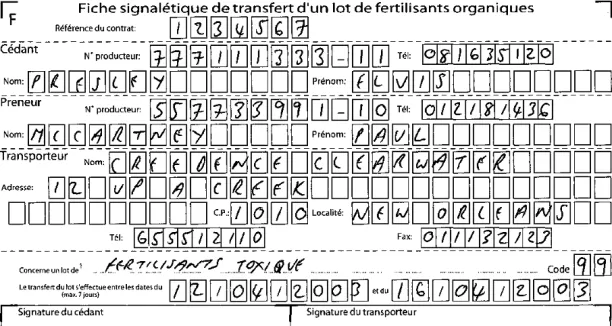
Use checkboxes if possible
Although written answers provide unique information, you sometimes need a simple selection from an existing set of choices. Instead of insisting on using handwriting recognition, using checkboxes would help you to limit the variety of potential answers while reducing possible errors and saving a significant amount of time.
For example, if you need a Yes/No answer or multiple selections from an existing set, using checkboxes will increase the accuracy.
Use Color Dropout Documents
In a color dropout form, the document layout is printed in a different color, most commonly red. Scanners can be calibrated to remove these colors, allowing only handwriting to appear. As a result, handwriting recognition tools don’t need to distinguish between handwritten characters and segmentation lines.
Source: Datacap.hk
Other Tips
You should also leverage from tips below to increase the handwriting recognition accuracy in your designed documents.
- Keep data within the margins
- Avoid colorful backgrounds
- Benefit from alignment elements to prevent skewed documents
- Barcodes will help you to find existing data instead of handwritten references
Is there active research on handwriting recognition?
As handwriting recognition capability highly depends on neural networks, advances in these algorithms profoundly affect the performance of handwriting recognition tools. Thus, active research on handwriting recognition is generally based on neural network algorithms.
Google’s research on handwriting recognition starts with several training steps:
- Introduction of all possible characters from different alphabets
- Training the tool for segmenting each character in a text
- Training the tool for feature extraction to accurate character identification
Google is also using language processing algorithms to improve handwriting recognition performance. For example, if the tool needs to decide between “i” and “l,” it can analyze the whole word and decide on the suitable character to provide accurate results.
OCR software usually has several handwriting recognition engines integrated into the software. These engines work synchronously to generate the most accurate character representation corresponding to the input.
Handwriting Recognition Vendors
As handwriting recognition is a subfield of OCR, the criteria for choosing the right handwriting recognition are similar to OCR tools. While selecting a handwriting recognition vendor, you should consider the following factors:
- Character recognition accuracy
- Continuous learning capabilities
- Computation speed in case results need to be delivered real-time
- User-friendliness of the interface if the interface will be used by humans
In addition to these, procurement best practices such as ensuring minimum Total Cost of Ownership (TCO), flexibility, data security best practices and avoiding vendor lock-in are important.
Below you can find a short list of handwriting vendors. You should also keep in mind that these vendors can also provide OCR services for your business. If you want to have the full list, you can visit our related page.
- Abbyy
- Google Cloud Vision API
- Hanvon Technology
- Hanwang Technology
- Infrrd.ai
- MicroBlink
- Microsoft Azure Read API
- Mitek
- MyScript
- Selvasai
- Unitek.ai
- Vidado
If you want to read more about handwriting recognition tools, these articles can also interest you:
- Optical Character Recognition (OCR): In-depth Guide
- Current State of OCR: Is It a Solved Problem?
- Data Extraction: In-depth guide for business users
- Document Automation Guide for Businesses
If you have questions on handwriting recognition tools, feel free to contact us:

Cem has been the principal analyst at AIMultiple since 2017. AIMultiple informs hundreds of thousands of businesses (as per similarWeb) including 60% of Fortune 500 every month.
Cem's work has been cited by leading global publications including Business Insider, Forbes, Washington Post, global firms like Deloitte, HPE, NGOs like World Economic Forum and supranational organizations like European Commission. You can see more reputable companies and media that referenced AIMultiple.
Throughout his career, Cem served as a tech consultant, tech buyer and tech entrepreneur. He advised businesses on their enterprise software, automation, cloud, AI / ML and other technology related decisions at McKinsey & Company and Altman Solon for more than a decade. He also published a McKinsey report on digitalization.
He led technology strategy and procurement of a telco while reporting to the CEO. He has also led commercial growth of deep tech company Hypatos that reached a 7 digit annual recurring revenue and a 9 digit valuation from 0 within 2 years. Cem's work in Hypatos was covered by leading technology publications like TechCrunch and Business Insider.
Cem regularly speaks at international technology conferences. He graduated from Bogazici University as a computer engineer and holds an MBA from Columbia Business School.
To stay up-to-date on B2B tech & accelerate your enterprise:
Follow onNext to Read
13 Rossum AI Competitors/Alternatives in 2024
OCR in 2024: Benchmarking Text Extraction/Capture Accuracy
Guide to Optical Character Recognition (OCR) in 2024
Hi Cem, your article is very clear and practical. Thank you for sharing your knowledge! It will be very useful for me.
See Transkribus from readcoop for handwritten text recognition for cursive writing.
Which service or software would you recommend in this case:
– manuscript/diary 100s of pages written by one author
– other language than English (German in this case)
I need the software to learn my handwriting, that is not in English and preferably with a good tool to correct all the error.
Thank you for reaching out. You can try Google Cloud Vision. It is not bad at handwriting recognition and is free to try. I don’t know if it can get user feedback to improve its models. Let us know if you find that functionality.
Comments
Your email address will not be published. All fields are required.