Artificial intelligence, particularly generative AI, offers promising solutions to address these challenges. By leveraging the power of generative AI, supply chain professionals can analyze massive volumes of historical data, generate valuable insights, and facilitate better decision-making processes.
Microsoft announced Microsoft Dynamics 365 Copilot, an AI-driven assistant integrated into CRM and ERP systems.1
Copilot in Microsoft Supply Chain Center has a news model that gathers all the supplier-related news that can potentially affect supply chains, such as natural disasters and geopolitical situations.
When notified, supply chain managers can send AI-generated and targeted emails to suppliers with Azure OpenAI Service. This is the first direct implementation of generative AI systems into supply chain operations. Figure 1 demonstrates how the assistant works, representing a significant advancement in supply chain visibility.
Figure 1. The technical mechanism of Copilot in Microsoft Supply Chain Center
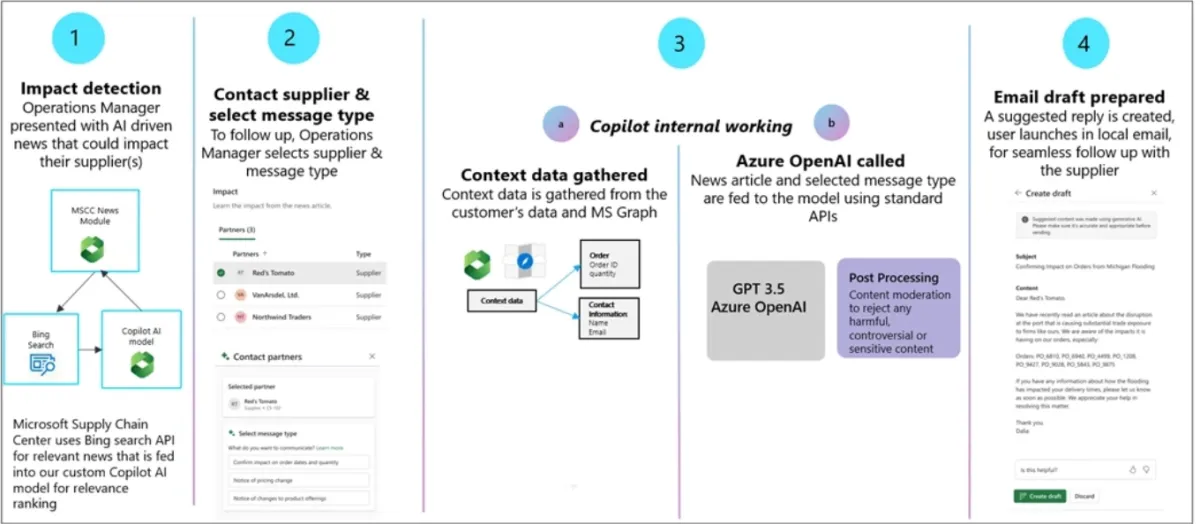
Source: Microsoft Dynamics 365 Blog2
In this article, we will list and explain the top 10 potential generative AI supply chain use cases.
Generative AI Supply Chain Use Cases
1- Demand forecasting
Generative AI creates models that can analyze large amounts of historical sales data, incorporating factors such as seasonality, promotions, and economic conditions. By training the AI model with this data, it can generate more accurate demand forecasts. This helps businesses better manage their inventory, allocate resources, and anticipate market trends.
Real-life Example: AI-Powered Inventory Forecasting
Amazon uses advanced AI algorithms to forecast demand and optimize inventory levels across its vast network of warehouses. The system analyzes massive datasets, including historical sales data, customer behavior, and external factors such as holidays and promotions.
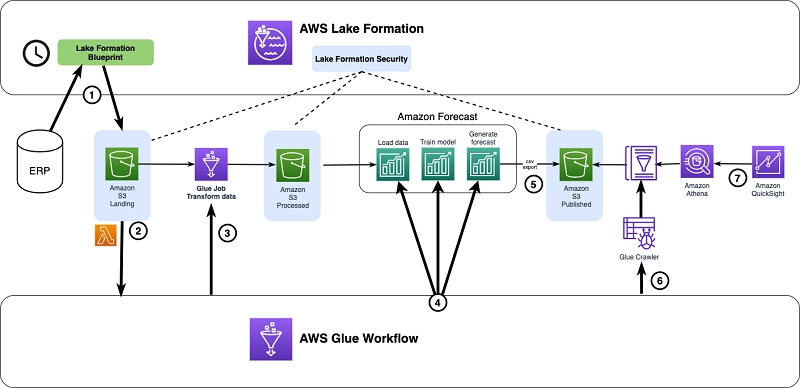
By integrating generative AI, Amazon can predict which products will be in demand at specific times, ensuring that its warehouses are stocked efficiently. This not only reduces storage costs but also helps avoid stockouts during peak demand periods like Prime Day or the holiday season.
Amazon’s inventory turnover is one of the highest in the industry, allowing the company to maintain a leaner inventory while still meeting customer expectations for fast delivery.
2- Supply chain optimization
Generative AI models can analyze various sources of visual or textual data, such as traffic conditions, fuel prices, and weather forecasts, to identify the most efficient routes and schedules for transportation. The AI can generate multiple possible scenarios, and based on the desired optimization criteria, it can suggest the best options for cost savings, reduced lead times, and improved operational efficiency across the supply chain.
Real-life Example: Unilever Supplier Risk Assessment
Unilever, a global consumer goods giant, has integrated AI into its supply chain to mitigate supplier risks. By leveraging machine learning and generative AI models, Unilever can monitor external events such as political unrest, natural disasters, and market shifts that might affect its suppliers. This system generates risk scores for each supplier and suggests alternative sourcing options when disruptions are likely.
For more information on such technologies, you can check our article on the AI uses cases for supply chain optimization.
3- Supplier risk assessment
By processing large volumes of data, including historical supplier performance, financial reports, and news articles, generative AI supply chain models can identify patterns and trends related to supplier risks. This helps businesses evaluate the reliability of suppliers, anticipate potential disruptions, and take proactive steps to mitigate risk, such as diversifying their supplier base or implementing contingency plans.
4- Anomaly detection
By analyzing data across various aspects of the supply chain, generative AI supply chain models can identify unusual patterns or deviations from the norm. This can help businesses quickly detect potential issues, such as bottlenecks, quality problems, or unexpected changes in demand, and address them before they escalate.
5- Product development
Generative AI can process market data, customer feedback, and competitor information to generate insights about potential gaps or opportunities in the market.
6- Sales and operations planning
Generative AI solutions can integrate data from sales, marketing, production, and distribution to generate more accurate and comprehensive plans. This helps businesses align their strategies across departments, optimize resource allocation, and better respond to changes in demand and market conditions.
7- Price optimization
Generative AI supply chain models can analyze factors such as customer demand, competitor pricing, and market conditions to generate optimal pricing strategies. These strategies can help businesses maximize revenue, profit margins, and market share while maintaining a competitive edge.
8- Transportation and routing optimization
Generative AI can play a significant role in transportation and routing optimization within supply chain management. By analyzing vast amounts of data from various sources, AI can generate efficient transportation plans, save time, and improve the overall efficiency of supply chain logistics.
Generative AI can enable:
- Route optimization with minimized expenses and timely deliveries
- Vehicle and fleet optimization with vehicle wear and tear, and resource utilization
- Dynamic routing with adaptation to disruptions and delays
These innovative solutions can help maintain a resilient supply chain.
Real-life Example: AI-Powered Route Optimization (ORION)
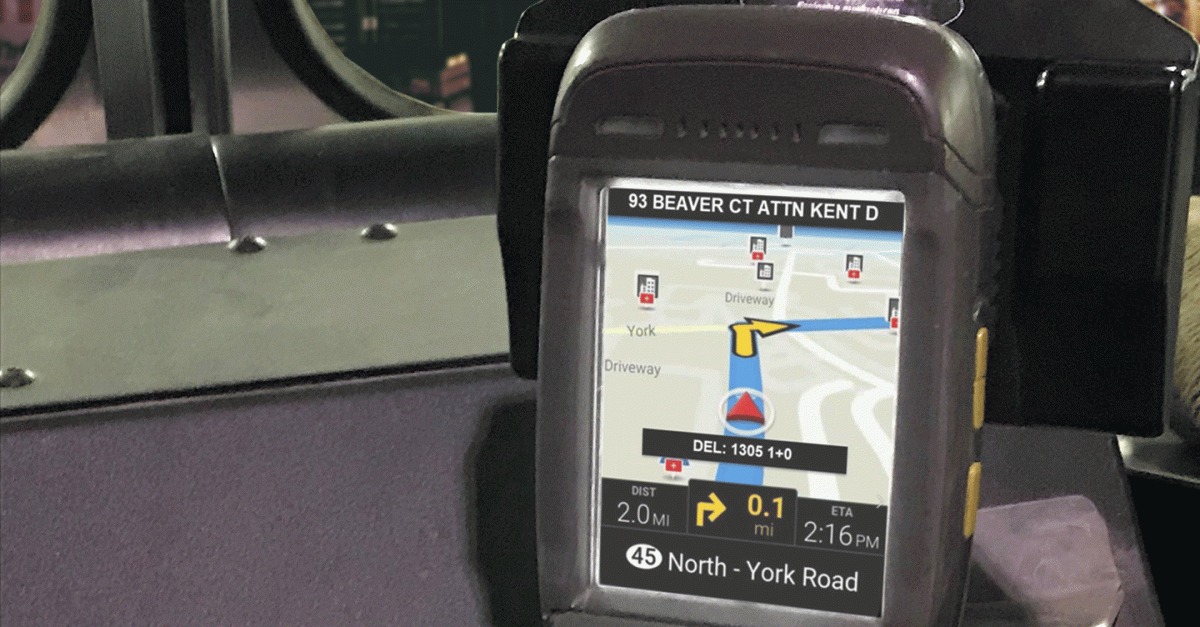
UPS uses its On-Road Integrated Optimization and Navigation (ORION) system, which leverages AI and advanced algorithms to optimize delivery routes in real-time. The generative AI models consider factors such as package volume, delivery windows, real-time traffic conditions, and even weather. The system continuously generates the most efficient routes, helping drivers minimize fuel consumption and delivery times.
ORION has helped UPS save over 10 million gallons of fuel annually, reducing costs and carbon emissions while improving on-time delivery performance.
9- Inventory Management
Generative AI models can analyze demand patterns, lead times, and other factors to determine the optimal inventory levels at various points in the supply chain. By generating suggestions for reorder points and safety stock levels, AI can help businesses with warehouse management by minimizing stockouts, reducing excess inventory, and lowering carrying costs.
10- Financial optimization in supply chain
Moreover, the use of generative AI in supply chain financial services and operations can significantly benefit supply chain management by improving efficiency, reducing risks, and enhancing decision-making processes.
The utilization of generative AI for financial operations of the supply chain can help supply chain leaders to solve many problems.
Credit risk assessment
Generative AI can analyze large volumes of data, including credit history, financial statements, and market information, to assess the creditworthiness of suppliers, partners, or customers. This helps supply chain stakeholders to manage financial risks, make informed decisions about extending credit, and identify potential defaults or disruptions in the chain.
Fraud detection and prevention
Generative AI models can analyze transaction data, identify patterns and anomalies, and detect potential fraud cases in the supply chain. This helps businesses minimize financial losses, protect their reputation, and ensure the integrity of their supply chain operations.
Risk management
AI can analyze various types of risks, such as currency fluctuations, interest rate changes, or geopolitical events, and generate insights to help businesses develop risk mitigation strategies. This can help supply chain stakeholders better manage financial risks and maintain supply chain stability.
FAQ
What is generative AI, and how is it used in supply chains?
Generative AI supply chain refers to artificial intelligence models that can create new content, designs, or solutions based on large data sets. In supply chains, it is used to predict demand, optimize routes, automate procurement, detect defects, and design products, among other tasks.
What industries are benefiting from the generative AI supply chain?
Industries such as retail, manufacturing, automotive, logistics, and consumer goods are significantly benefiting from generative AI in their supply chains. AI helps these sectors optimize operations, reduce costs, and improve customer satisfaction through predictive insights and process automation.
Comments
Your email address will not be published. All fields are required.