Data Mesh vs. Data Lake: Choosing the Right Tools in 2024
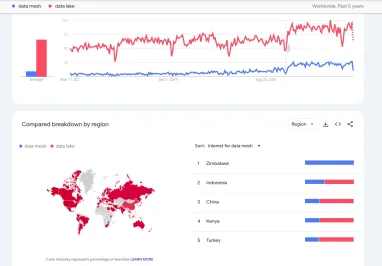
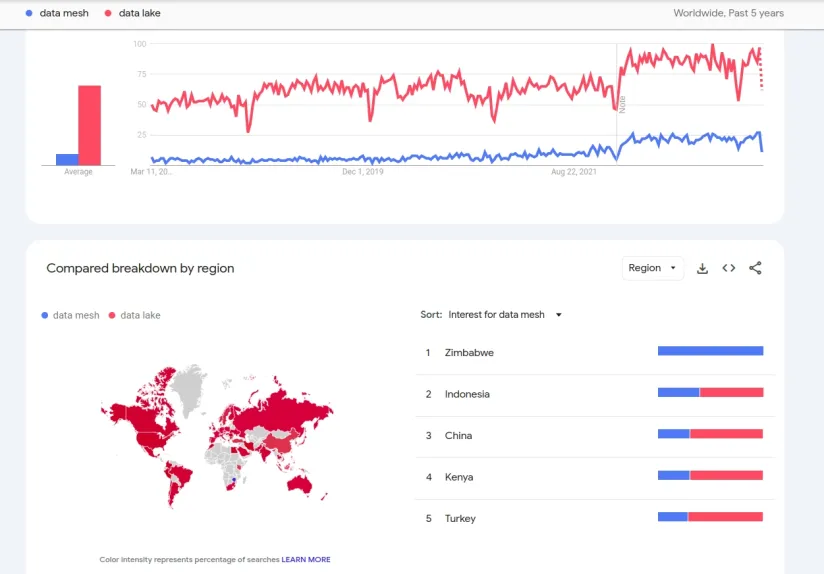
Figure 1. Interest in data mesh and data lake in the last 5 years.1
Data management has become a critical part of any organization’s success, and as data volumes continue to grow, traditional data management strategies have become less effective. Two relatively new concepts in the field of data management are data mesh and data lake.
Since 2021, interest in these technologies has grown dramatically, but few people are aware of how they differ. (Figure 1). In this article, we’ll explore the differences between data lakes and data meshes, including:
- Their advantages and disadvantages
- The challenges organizations face when implementing them
- The future of data management
What is a data mesh?
A data mesh is a decentralized approach to data management that places data ownership and responsibility on individual teams or domains rather than a central data team.
In a data mesh architecture, data is treated as a product that is designed, built, and maintained by individual teams. These teams are responsible for the quality and governance of the data they own, and they provide access to that data through well-defined APIs. Interest in data mesh has significantly increased since 2020.
3 benefits of data mesh
The advantages of a data mesh include:
- Increased agility: A data mesh architecture enables each team to own and manage their data, which can result in faster development cycles.
- Faster time-to-market: Since individual teams are responsible for managing their data, they can independently develop their services or applications, reducing the time it takes to bring new products or services to market.
- Better alignment with business needs: A data mesh approach encourages teams to focus on specific business domains and deliver value to the organization by addressing the unique needs of each domain.
A common data mesh use case
A common use case for a data mesh is to have data infrastructure to support microservices architecture, where each microservice has its own data store and manages its own data.
Challenges of a data mesh
- Risk of data silos: Implementing a data mesh design can lead to data silos. Since each team in a data mesh approach owns and manages its own data, it can lead to data being stored in multiple different systems or data stores, which can create data silos. This can make it difficult to share data across different teams or domains, resulting in duplication of effort and potentially conflicting data.
- Increased data management complexity: Managing multiple data stores and APIs can lead to increased complexity. This can make it difficult for organizations to maintain and manage their data mesh architecture. To ensure that the system is properly designed, built, and maintained, data mesh may necessitate the use of additional resources and specialized skills.
What is a data lake?
A data lake is a centralized repository of raw data that can be stored in any format, such as structured and unstructured. Data is ingested into the data lake in its native format, and then transformed and processed as needed.
3 benefits of data lake
Data lake architecture is designed to store large amounts of data and allow for fast querying and analysis. The advantages of a data lake include:
- Scalability: Data lakes are designed to handle large volumes of data, making them highly scalable. As the volume of data increases, data lakes can easily expand to accommodate new data sources and larger data sets, without the need for major infrastructure upgrades.
- Flexibility: Data lakes are flexible in the types of data they can store, as they can store data in its raw, unprocessed format. This allows data analysts and data scientists to work with data in its native format, rather than having to conform to a specific data schema. Additionally, data lakes can handle structured, semi-structured, and unstructured data, providing flexibility in how data is stored and processed.
- Cost-effectiveness: Data lakes are typically less expensive than traditional data warehousing solutions, as they use low-cost storage options such as cloud-based object storage. This makes it easier for organizations to store and manage large volumes of data without incurring significant infrastructure costs. Additionally, since data is stored in its raw format, there is no need for costly ETL (extract, transform, load) processes to convert data into a specific format.
A common data lake use case
A common use case for a data lake is to consolidate data from multiple sources to support analytics and machine learning applications. For example, a retail company might use a data lake to store data from various sources, such as sales transactions, customer feedback, and inventory records from data warehouses.
Challenges of data lakes
However, data lakes can also suffer from:
- Data quality issues: Since data lakes can ingest raw data in its native format, there is a higher risk of data quality issues such as data duplication, inconsistent data, and data errors.
- Lack of data governance: A data lake may not have a centralized team responsible for data governance, which can result in inconsistencies in data definitions, data access policies, and data security policies.
- Difficulty in managing access to data: Since a data lake can contain sensitive data from different sources, it can be challenging to manage access to data for different teams and individuals.
Data lake vs data mesh: Differences and similarities
In the context of data management, data lakes, and data mesh are two different approaches that have fundamentally different data architectures, including:
- The degree of centralization,
- The focus on data consolidation or distribution
- The role of governance
- The number of sources of truth
1. Centralized vs decentralized
Data lakes are centralized, meaning that all data is stored in a single repository or data warehouse. In contrast, data mesh is decentralized, meaning that data ownership is distributed across individual teams or domains.
2. Consolidating vs distributing
Data lakes focus on consolidating data from multiple sources into a single repository or data warehouse. Distributed data mesh, on the other hand, focuses on distributing data ownership to individual teams or domains, allowing for more flexible and agile data management.
3. Centralized governance vs individual teams
Data lakes rely on a central data team to manage data governance, ensuring that data is accurate, consistent, and secure. In contrast, data mesh relies on individual teams to manage data quality and governance, which can lead to more decentralized and autonomous data management practices.
4. Single source of truth vs multiple versions of the truth
Data lakes provide a single source of truth, meaning that all data in the repository is considered to be the authoritative version of the data. Data mesh allows for multiple versions of the truth, meaning that each team or data consumer can have its own version of the data that it considers to be authoritative.
5. Summary table of data lake and data mesh differences
Feature | Data mesh | Data lake |
---|---|---|
Centralized vs. decentralized | Data ownership is distributed across individual teams or domains | Data is stored in a single repository or data warehouse |
Consolidating vs. distributing | Focuses on distributing data ownership to individual teams or domains,
allowing for more flexible and agile data management
| Focuses on consolidating data from multiple sources into a single
repository or data warehouse
|
Centralized governance vs. individual Teams | Relies on individual teams to manage data quality and governance,
which can lead to more decentralized and autonomous data management practices
| Relies on a central data team to manage data governance,
ensuring that data is accurate, consistent, and secure
|
Single source of truth vs. multiple versions of the truth | Allows for multiple versions of the truth, meaning that each
team or domain may have its own version of the data that it considers to be authoritative
| Provides a single source of truth, meaning that all data
in the repository is considered to be the authoritative version of the data
|
Table 1: Data lake vs. data mesh.
Shared goals of data lake and data mesh
Data lake and data mesh are two approaches to enterprise data management that share common goals, including:
- Providing easy access to high-quality data: Both data lake and data mesh share the goal of providing easy access to high-quality data for analysis and decision-making.
- Improved collaboration: Both approaches can facilitate better communication and collaboration between teams by providing access to high-quality data and empowering teams to manage their own data.
3 Best practices in implementing data lake and data mesh
Implementing data lakes and data mesh can be challenging for organizations. The following best practices can be helpful to overcome common challenges belonging to data lake and data mesh:
- Ensure data quality: Ingesting large amounts of raw data can result in data inconsistency and errors. Organizations must implement data profiling, data cleansing, and data lineage practices to ensure that data is accurate, reliable, and trustworthy.
- Plan for scaling: As data volumes continue to grow rapidly, scalability can become a concern for data engineers and business users. Organizations must ensure that their data management practices can handle increasing data volumes without sacrificing performance, speed, or reliability.
- Think about data security: Data lakes and data mesh contain sensitive data that must be protected. Data lakes and data mesh often contain sensitive and confidential data, such as customer data or trade secrets. This data must be protected from unauthorized access, data breaches, cyber-attacks, and other security threats (Figure 4).
Choosing the right approach
One approach may be more appropriate than the other depending on the specific needs of an organization. When deciding between a single data lake and data mesh, organizations should carefully consider their needs and goals; in some cases, a combination of both approaches can be the best solution.
Future of data management
Data lakes and data mesh are evolving rapidly, and new technologies and approaches to data platforms are emerging that will shape the future of data management. The following technological approaches are expected to be more widely used in data management next to data lakes and data mesh:
1. Data catalogs
Data catalogs provide a centralized metadata repository that allows users to discover and access data across multiple data sources. Data catalogs have been more widely interesting since 2020s.
2. AI and machine learning (ML)
AI and ML are used for automating data management tasks such as data profiling, data cleansing, and data governance.
3. Recent data mesh architectures
Data mesh architectures that can provide more flexible and autonomous data management practices, while still ensuring data quality, security, and governance can be more widely used in the future.
For more on data lakes and data mesh, and data sharing please contact us at:
External Links

Cem has been the principal analyst at AIMultiple since 2017. AIMultiple informs hundreds of thousands of businesses (as per similarWeb) including 60% of Fortune 500 every month.
Cem's work has been cited by leading global publications including Business Insider, Forbes, Washington Post, global firms like Deloitte, HPE, NGOs like World Economic Forum and supranational organizations like European Commission. You can see more reputable companies and media that referenced AIMultiple.
Throughout his career, Cem served as a tech consultant, tech buyer and tech entrepreneur. He advised businesses on their enterprise software, automation, cloud, AI / ML and other technology related decisions at McKinsey & Company and Altman Solon for more than a decade. He also published a McKinsey report on digitalization.
He led technology strategy and procurement of a telco while reporting to the CEO. He has also led commercial growth of deep tech company Hypatos that reached a 7 digit annual recurring revenue and a 9 digit valuation from 0 within 2 years. Cem's work in Hypatos was covered by leading technology publications like TechCrunch and Business Insider.
Cem regularly speaks at international technology conferences. He graduated from Bogazici University as a computer engineer and holds an MBA from Columbia Business School.
To stay up-to-date on B2B tech & accelerate your enterprise:
Follow on
Comments
Your email address will not be published. All fields are required.