AI Data Collection in 2024: Guide, Challenges & Methods
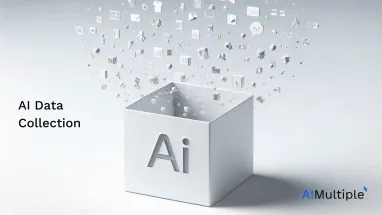
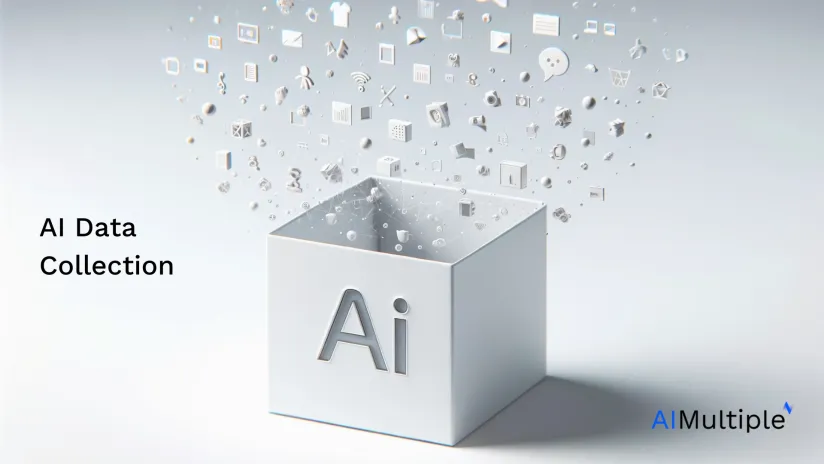
The adoption of generative AI and other AI-powered solutions is rapidly growsing1 Organizations need to collect and harvest large amounts of data, either by themselves or by working with AI data collection services, to successfully leverage these technologies, specifically to train and improve them. Due to this growing need for data, AI data collection is gaining more interest over the past few years.
This article provides an in-depth guide to AI data collection to help business leaders and developers streamline their journey to an AI-enhanced world.
Figure 1: Rising interest in AI data collection in the past few years
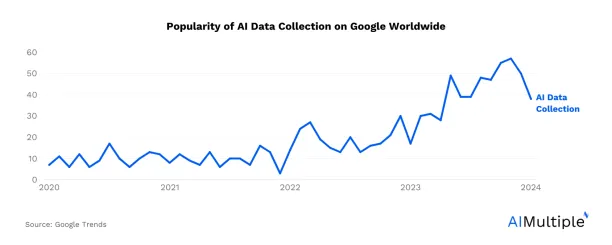
What is AI data collection?
Data collection/harvesting is the process of extracting data from different sources such as websites, online surveys, user feedback forms, customer social media posts, ready-made datasets, etc. This collected data can then be used in training & improving AI/ML models.
Collecting high-quality data is one of the most important steps in developing a robust AI/ML model. In other words, the accuracy of an AI model is as good as the quality of its data. The garbage in, garbage out principle applies here. Therefore, to gather reliable data, practices to ensure data consistency and quality should be in place. To learn more about data collection quality assurance, check out this quick read.
Google Gemini example
Google Gemini’s image generation tool is a good example of biased AI data for training large language models (LLMs). The tool started to create racially inappropriate images and was recalled by Google.2
How is it done?
Data collection is done by gathering data from different sources and storing it for further use. For instance, to collect relevant data for security monitoring systems, the collectors need to gather video footage from surveillance cameras at different times of the day. On the other hand, automated methods of collecting online data, which we will explain later in the article, can also be used to collect existing data, such as images or video footage from online sources.
The process of collecting data for AI also involves generating new data since some AI models require human-generated data or specific types of data to learn how to perform tasks like humans. For instance, generative AI models require large volumes of human-generated data to be able to learn how to generate content like humans.
6 steps to collect data
To learn more about the 5 steps of collecting data.
1. Identifying the need:
The most crucial initial step in the data collection process. Determine the scope of the project to select the right dataset type.
You can compare data collection services based on data typed in the following articles:
- 10+ Image Data Collection Services
- 7+ Video Data Collection Services & Selection Criteria
- 10+ Speech Data Collection Services
2. Selecting the method
Select the collection method which is most suitable for your project. A multilingual large-scale dataset can be better gathered through crowdsourcing, whereas secretive healthcare data can be gathered in-house.
3. Quality assurance
As you gather the raw data, ensure it’s cleaned and improved. Make sure the final dataset is of high quality.
4. Storing the data
A sound storage plan is essential regardless of your chosen method for collecting data. Consider privacy concerns, storage capacity, post-storage data management, etc.
5. Annotating the data
This involves labeling or tagging data for machine readability. Even though this step does not directly involve gathering the data, it helps prepare the dataset for final usage.
To learn more about data annotation
Defining data needs is important to ensure that the dataset aligns with the project’s scope and that irrelevant data from existing datasets is removed.
While the main focus of this article is to focus on data collected for AI/ML development, some other uses of data collection are:
- Fueling marketing campaigns,
- Conducting primary and secondary research
- Conducting an online survey
Learn more about other reasons for data collection in this article.
What are the challenges?
The whole process of gathering data can be challenging. According to a survey (Figure 2) conducted by McKinsey on 100 companies that implemented AI in their business, 24% stated that collecting and harvesting relevant data was the largest barrier in their AI implementation and development process. The following data collection problems can arise:
- There is an ocean of data out there; however, not all of it can be easily accessed. Since data can be sensitive and private, there are various regulations and policies that prevent organizations from accessing or using it. For instance, healthcare data is rather difficult to find due to privacy issues.
- There are also ethical and legal data collection considerations that, if disregarded, can lead to expensive lawsuits.
- The data available for training purposes can also be biased and can provide erroneous outcomes. To learn more about AI bias, check out this comprehensive article.
- Even if the data is safe to use and unbiased, it can still be unusable because it can be incomplete, irrelevant, or outdated.
- Using raw data is not possible while training AI/ML models due to data quality issues. Therefore, preprocessing the data is an important step after collecting it to protect data integrity and ensure quality control.
- Data collection costs can also be a challenge; therefore, business leaders need to consider them in the initial planning process of the project. For instance, the costs can include recruitment costs, data collection equipment costs, etc.
To learn more about data collection/sourcing challenges and solutions, check out this quick read.
Watch the video below to learn the importance of data for AI algorithms.
Figure 2. Barriers to AI adoption.3
How does it differ from data mining, web scraping, and data extraction?
This section looks at the key differences and similarities between data collection and data mining, data extraction, and web scraping to overcome confusions that might arise while using these terms.
Data collection/harvesting vs. data mining
- Data collection is the process of harvesting data from different sources and storing it for further use, such as training AI/ML models.
- Data mining is the process of extracting and identifying patterns in a large dataset by using mathematical models. This step usually comes after data collection.
Data collection/harvesting vs. web scraping
- These terms are sometimes used interchangeably but have a minor difference. While data collection involves offline and online methods of gathering or generating data, web scraping only gathers data from online sources. Web scraping is usually used to gather:
- Social media data
- Data from corporate websites
- News sources, etc.
Data collection/harvesting vs. data extraction
- While data collection gathers the data, data extraction is the process of turning unstructured or semi-structured data into structured data.
What are the top 6 AI data collection methods?
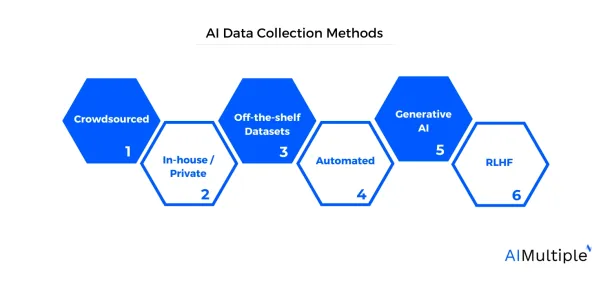
This section answers the question: where does AI get data from? And what are the methods of collecting data:
1. Crowdsourced data collection
This is an effective primary data collection and generation method. Crowdsourcing refers to working with a large network of people to gather or generate data.
Suppose an image recognition system requires image data of road signs. Through public crowdsourcing, its developers can obtain these images from the public by providing some instructions to users of the network and creating a data-sharing platform.
However, this method can not be used for projects involving sensitive or confidential data. Working with a third-party crowdsourcing platform or service provider can add cost-effectiveness and improved data quality to this method’s positives.
You can also work with a data crowdsourcing platform that specializes in AI data collection and generation.
Here are some popular names in the data crowdsourcing space:
2. In-house / private data collection
This is also one of the primary data collection methods. In this method, the AI/ML developers collect their own data privately instead of working with the general public. The company recruits its own data generators/collectors, processes the collected data by itself, and stores it in its private servers. An example of private sourcing is surveying. In-house data collection can be time-consuming if done manually and in-house.
3. Off-the-shelf data
This is a method of obtaining third-party data which was generated or gathered in the past. Prepackaged data may be considered a quick fix for accessing data, but it can consume more time and effort than expected by developers. With prepackaged data, companies often need to make customizations, create APIs for integration, and write code. All this can be time and resource-consuming.
4. Automated data collection
This section answers the question; how does AI collect data itself? The answer is through automated tools. Automation is another popular method of gathering data more efficiently. This is done by using software to gather data from online data sources automatically. Some methods of automating data harvesting include; Web-scraping, web crawling, using APIs, etc.
While automation can improve the accuracy of the data collection process, it can only be used to gather secondary data and can not be used for primary data collection. Check out this quick read to learn more about data collection automation, its methods, and its top pros & cons.
5. Generative AI
After the launch of OpenAI’s ChatGPT, generative AI took the tech industry by storm. Generative AI is a new way of preparing AI training datasets. These models can create synthetic data that resembles real-world data. This synthetic data can be used to augment existing training datasets or even create new ones.
For example, a generative model could produce additional images, text, or other data points that are then mixed with real data to train another machine-learning model. This is especially useful when you have limited labeled data, as it helps improve the model’s accuracy and generalization capabilities.
6. RLHF or Reinforcement learning from human feedback
Reinforcement Learning from Human Feedback, or RLHF, is another new concept that can be used to gather AI training data. In RLHF, an initial model is trained using basic rewards or imitation learning. This model generates trajectories—sequences of actions and states. Humans review these trajectories to provide feedback, such as correcting actions or ranking them. This feedback is then used (as new training data) to fine-tune the model.To learn more about the different methods of collecting/harvesting data, check out this quick read.
To learn more about the different methods of collecting/harvesting data, check out this quick read.
You can also check our data-driven list of data collection/harvesting services to find the option that best suits your project needs. To evaluate data collection vendors, you can also download our free guide:
Further reading
- Top 6 Data Collection Best Practices
- Top 4 Data Collection Challenges and Their Solutions
- Top 5 Data Collection Trends for Data-Driven Businesses
If you need help finding a vendor or have any questions, feel free to contact us:
Transparency statement
AIMultiple sponsors include technology service providers that receive links from this article.
External resources
- 1. Stanford University. (2023). Artificial intelligence (AI) adoption worldwide 2022, by industry and function. Statista. Accessed: 15/August/2023.
- 2. Why Google’s ‘woke’ AI problem won’t be an easy fix. BBC. Accessed: 27/March/2024.
- 3. McKinsey Survey. (2018). AI adoption advances, but foundational barriers remain. McKinsey & Company. Accessed: 14/June/2022.

Comments
Your email address will not be published. All fields are required.