Guide to RLHF in 2024
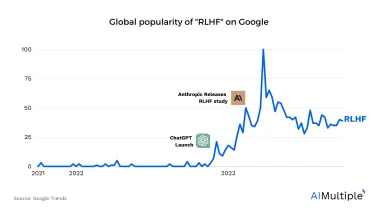
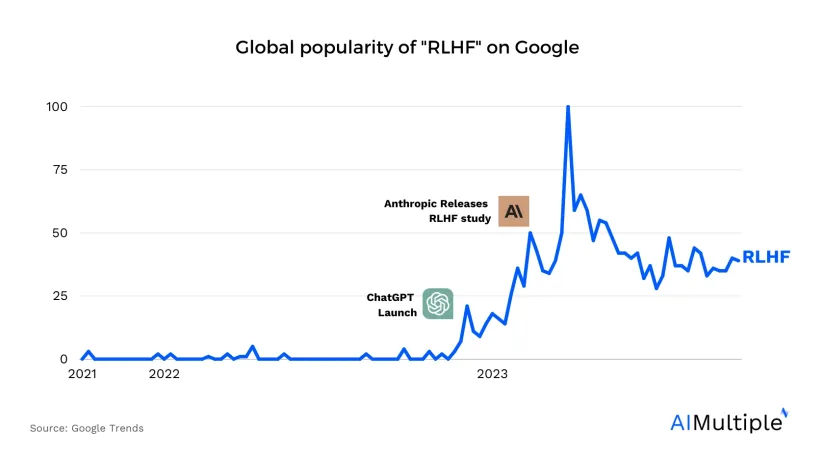
In the rapidly advancing world of artificial intelligence (AI), developers strive to create machines capable of learning autonomously. The field of reinforcement learning, a subset of machine learning, plays a crucial role in these efforts, setting the stage for AI systems to learn from their actions.
A recent development in RL is becoming popular – Reinforcement Learning from Human Feedback. This approach intertwines human insights with advanced algorithms, providing an efficient and effective way to train AI models, and is gaining popularity.
In this article, we explore the benefits and challenges of RLHF and provide the top 5 companies on the market that offer RLHF services.
Here is a guide to finding the right RLHF platform for your AI project.
What is reinforcement learning from human feedback (RLHF)?
Reinforcement learning from human feedback, or RLHF, is a method where an AI learns optimal actions based on human-provided feedback. To grasp the concept of RLHF better, it’s essential to understand reinforcement learning (RL).
What is reinforcement learning?
RL is a type of machine learning where an agent (RL algorithm) learns to make decisions by taking actions in an environment (anything the agent interacts with) to achieve a goal. The agent receives rewards or penalties based on the actions taken, learning over time to maximize the reward.
Figure 1: A basic reinforcement learning model
Reinforcement Learning from Human Feedback
RLHF, on the other hand, refines this process by integrating human feedback into the learning loop. Instead of depending solely on the reward function predefined by a programmer, RLHF leverages human intelligence to guide the learning process.
Simply put, the agent learns not only from the consequences of its actions but also from the human feedback. This human feedback can be corrective, pointing out where the agent has gone wrong, or affirmative, reinforcing the right decisions made by the agent.
Coffee-making analogy to simplify the concept of RLHF
Imagine teaching a robot to make a cup of coffee. Using traditional RL, the robot would experiment and figure out the process through trial and error, potentially leading to many suboptimal cups or even some disasters. With RLHF, a human can provide feedback, steering the robot away from mistakes and guiding it towards the correct sequence of actions, reducing the time and waste involved in the learning process. To visualize the concept, see the image below.
Figure 2: An RLHF model
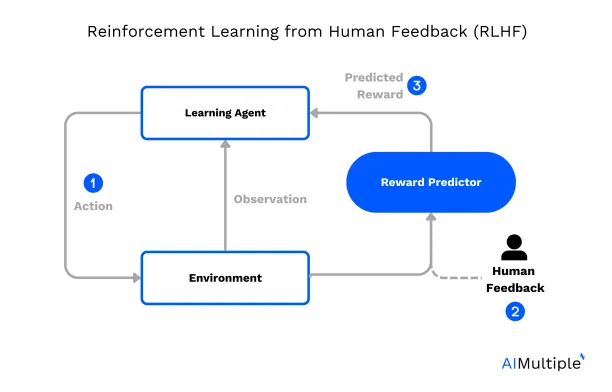
Use cases of RLHF
This section highlights some ways you can use the RLHF approach.
1. Natural language processing (NLP)
RLHF has proved to be effective in diverse NLP domains, including crafting more contextually appropriate email responses, text summarization, conversation agents, and more. For instance, RLHF enables language models to generate more verbose responses, reject inappropriate queries, and align model outputs with complex human values.
One of the most popular examples of RLHF-trained language models is OpenAI’s ChatGPT and its predecessor, InstructGPT.
2. Education, business, healthcare, and entertainment
RLHF finds applications in generating solutions for math problems, and coding, and has broad use cases across sectors like education, business, healthcare, and entertainment.efn_note]Liu, G.K.M., (2023). Transforming Human Interactions with AI via Reinforcement Learning with Human Feedback (RLHF). Accessed: 17/October/2023.[/efn_note]
3. Video game development
In the game-developing space, RLHF has been employed to develop bots with superior performance, often surpassing human players. For example, agents trained by OpenAI and DeepMind to play Atari games based on human preferences showcased strong performance across various tested environments.efn_note]Christiano, Paul F; Leike, Jan; Brown, Tom; Martic, Miljan; Legg, Shane; Amodei, Dario (2017). “Deep Reinforcement Learning from Human Preferences”. Advances in Neural Information Processing Systems. Curran Associates, Inc. 30. Accessed: 4/March/2023.[/efn_note]
4. Summarization tasks
RLHF has also been applied to train models for better text summarization, showcasing its potential in enhancing the quality of summarization AI models. This is done by using human feedback to guide the model’s learning process towards generating concise and informative summaries.
By iteratively adjusting the model based on human evaluations of generated summaries, RLHF facilitates a more human-aligned performance in summarization, ensuring the produced summaries are coherent, relevant, and adhere to a high standard of quality
5. Robotics
In a more interactive and whimsical application, RLHF can be envisioned to teach robots to behave like humans and assist in complex tasks through interactive and playful interactions.efn_note]Thomaz, A.L., Hoffman, G. and Breazeal, C., (2006). Reinforcement learning with human teachers: Understanding how people want to teach robots. In ROMAN 2006-The 15th IEEE International Symposium on Robot and Human Interactive Communication (pp. 352-357). Accessed: 17/October/2023.[/efn_note]
The benefits of RLHF
This section highlights some of the benefits of leveraging the RLHG approach.
1. Enhancing learning efficiency
One of the primary benefits of RLHF is its potential to boost learning efficiency. By including human feedback, RL algorithms can sidestep the need for exhaustive trial-and-error processes, speeding up the learning curve and achieving optimal results faster.
2. Addressing ambiguity and complexity
RLHF can also handle ambiguous or complex situations more effectively. In conventional RL, defining an effective reward function for complex tasks can be quite challenging. RLHF, with its ability to incorporate nuanced human feedback, can navigate such situations more competently.
3. Safe and ethical learning
Lastly, RLHF provides an avenue for safer and more ethical AI development. Human feedback can help prevent AI from learning harmful or undesirable behaviors. The inclusion of a human in the loop can help ensure the ethical and safe operation of AI systems, something of paramount importance in today’s world.
Challenges and recommendations for RLHF
While RLHF holds immense promise, it also comes with its own set of challenges. However, with every challenge comes an opportunity for innovation and growth.
1. Quality and consistency of human feedback
The efficacy of RLHF heavily relies on the quality and consistency of the human feedback provided. Inconsistent or erroneous feedback can derail the learning process.
Recommendation
This challenge can be mitigated by incorporating multiple feedback sources or by using sophisticated feedback rating systems that gauge the reliability of the feedback providers. Working with an RLHF platform with a large network of contributors can also help, since then the tasks will be divided into many micro-tasks, and the quality can be assured much easily.
2. Scalability
As AI systems handle increasingly complex tasks, the amount of feedback needed for effective learning can grow exponentially, making it difficult to scale.
Recommendation
One way to address this issue is by combining RLHF with traditional RL. Initial stages of learning can use human feedback, while more advanced stages rely on pre-learned knowledge and exploration, reducing the need for constant human input. Outsourcing to a third-party service provider can help manage scalability.
3. Over-reliance on human feedback
There’s a risk that the AI system might become overly reliant on human feedback, limiting its ability to explore and learn autonomously.
Recommendation
A potential solution is to implement a decaying reliance on human feedback. As the AI system improves and becomes more competent, the reliance on human feedback should gradually decrease, allowing the system to learn independently.
4. Manpower and costs
There are costs associated with RLHF. Such as:
- Recruiting experts to provide feedback
- The technology and infrastructure needed to implement RLHF
- Development of user-friendly interfaces for feedback provision
- Maintenance and updating of these systems
Recommendations
Working with an RLHF service provider can help streamline the process of using RLHF for training AI models.
Further reading
If you need help finding a vendor or have any questions, feel free to contact us:

Comments
Your email address will not be published. All fields are required.