Synthetic Data Generation in 2024: Techniques & Best Practices
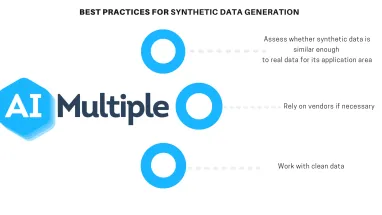
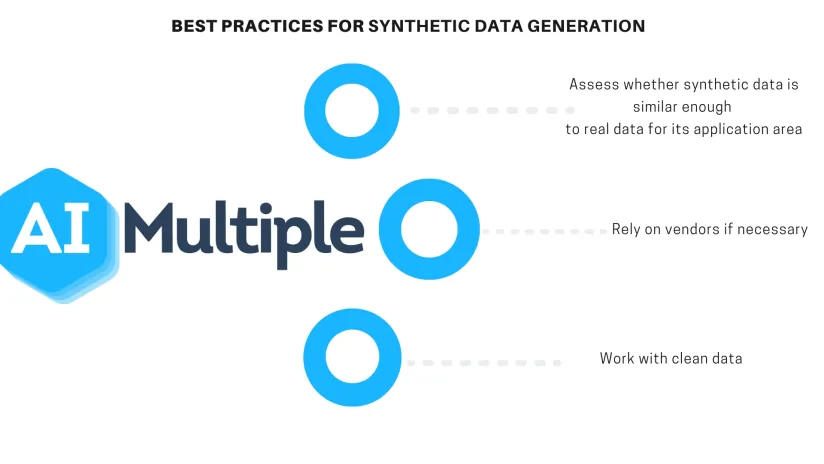
Synthetic data is artificial data generated to preserve privacy, testing systems, or create training data for machine learning algorithms. Synthetic data generation is critical since it is an important factor in the quality of synthetic data; for example, synthetic data that can be reverse-engineered to identify real data would not be useful in privacy enhancement.
As in most AI-related topics, deep learning comes up in synthetic data generation as well. So synthetic data created by deep learning algorithms is also being used to improve other deep learning algorithms. We explained other synthetic data generation techniques, as well as best practices:
What is synthetic data?
Synthetic data is artificial data that is created by using different algorithms that mirror the statistical properties of the original data but do not reveal any information regarding real people. For more information on synthetic data, feel free to check our comprehensive synthetic data article.
Why is synthetic data important for businesses?
Synthetic data is important for businesses for three reasons: privacy, product testing, and training machine learning algorithms. Industry leaders also started to discuss the importance of data-centric approaches to AI/ML model development, to which synthetic data can add significant value. For more detailed information, please check our ultimate guide to synthetic data.
When to use synthetic data
Businesses face a trade-off between data privacy and data utility when selecting a privacy-enhancing technology. Therefore they need to determine the priorities of their use case before investing. Synthetic data does not contain any personal information; it is sample data that has a distribution similar to the original data. Though the utility of synthetic data can be lower than real data in some cases, there are also cases where synthetic data is almost as valuable as real data. For instance, a team at Deloitte Consulting generated 80% of the training data for a machine-learning model by synthesizing data. The resulting model accuracy was similar to a model trained on real data.
Especially when companies need data to train machine learning algorithms and their training data is highly imbalanced (e.g. more than 99% of instances belong to one class), synthetic data generation can help build accurate machine learning models.
For more, feel free to check our comprehensive list of synthetic data use cases.
How do businesses generate synthetic data?
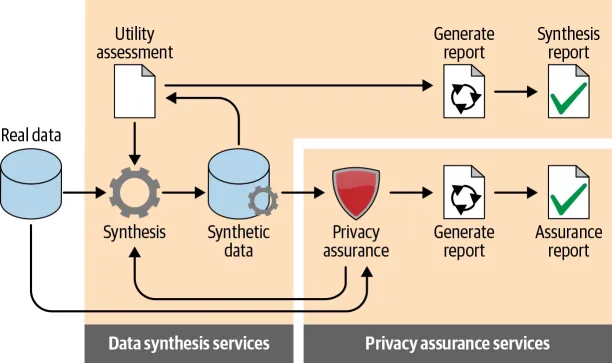
Businesses can prefer different methods such as decision trees, deep learning techniques, and iterative proportional fitting to execute the data synthesis process. They should choose the method according to synthetic data requirements and the level of data utility that is desired for the specific purpose of data generation.
After data synthesis, they should assess the utility of synthetic data by comparing it with real data. The utility assessment process has two stages:
- General purpose comparisons: Comparing parameters such as distributions and correlation coefficients measured from the two datasets
- Workload-aware utility assessment: comparing the accuracy of outputs for the specific use case by performing analysis on synthetic data
What are the techniques of synthetic data generation?
Generating according to distribution
For cases where real data does not exist but data analyst has a comprehensive understanding of how dataset distribution would look like, the analyst can generate a random sample of any distribution such as Normal, Exponential, Chi-square, t, lognormal and Uniform. In this technique, the utility of synthetic data varies depending on the analyst’s degree of knowledge about a specific data environment.
Fitting real data to a known distribution
If there is real data, then businesses can generate synthetic data by determining the best-fit distributions for given real data. If businesses want to fit real-data into a known distribution and they know the distribution parameters, businesses can use Monte Carlo method to generate synthetic data.
Though the Monte Carlo method can help businesses find the best fit available, the best fit may not have good enough utility for a business’ synthetic data needs. For those cases, businesses can consider using machine learning models to fit the distributions. Machine learning models such as decision trees allow businesses to model non-classical distributions that can be multi-modal and do not contain common characteristics of known distributions. With this machine learning fitted distribution, businesses can generate synthetic data that is highly correlated with original data. However, machine learning models have a risk of overfitting that fail to fit new data or predict future observations reliably.
For cases where only some part of real data exists, businesses can also use hybrid synthetic data generation. In this case, analysts generate one part of the dataset from theoretical distributions and generate other parts based on real data.
Using deep learning
Deep generative models such as Variational Autoencoder(VAE) and Generative Adversarial Network (GAN) can generate synthetic data.
Variational Autoencoder
VAE is an unsupervised method where the encoder compresses the original dataset into a more compact structure and transmits data to the decoder. Then, the decoder generates an output, which is a representation of the original dataset. The system is trained by optimizing the correlation between input and output data.
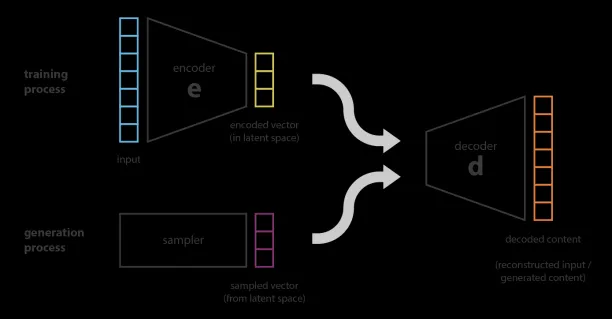
Generative adversarial network
In the GAN model, two networks, a generator, and discriminator, train the model iteratively. The generator takes random sample data and generates a synthetic dataset. Discriminator compares synthetically generated data with a real dataset based on conditions that are set before.
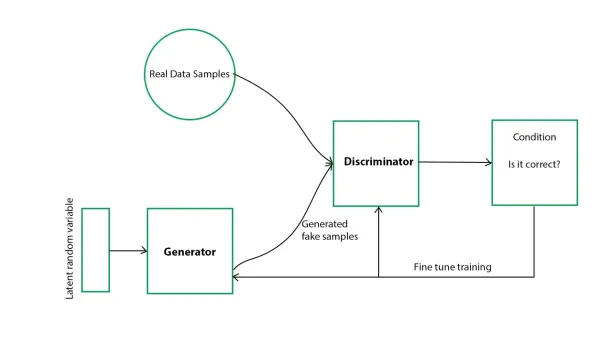
How to generate synthetic data in Python?
Python is one of the most popular languages, especially for data science. There are three libraries that data scientists can use to generate synthetic data:
- Scikit-learn is one of the most widely used Python libraries for machine learning tasks, and it can also be used to generate synthetic data. One can generate data that can be used for regression, classification, or clustering tasks.
- SymPy is another library that helps users to generate synthetic data. Users can specify the symbolic expressions for the data they want to create, which helps users create synthetic data according to their needs.
- Pydbgen: Categorical data can also be generated using Python’s Pydbgen library. Users can generate random names, international phone numbers, email addresses, etc., easily using the library.
What are the best practices?
- Work with clean data: Clean data is an essential requirement of synthetic data generation. If you don’t clean and prepare data before synthesis, you can have garbage-in, garbage-out situation. In the data preparation process, make sure you apply the following principles:
- Data cleaning: Eliminating inaccurate, improperly formatted, redundant, or lacking data from a dataset
- Data harmonization: Synthesizing data from several sources and providing customers with a comparable understanding of information from various research.
- Assess whether synthetic data is similar enough to real data for its application area: The utility of synthetic varies depending on the technique you use while generating it. You need to analyze their use case and decide if the generated synthetic data is a good fit the specific use case.
- Outsource support if necessary: Identify your organization’s synthetic data capabilities and outsource based on the capability gaps. The 2 important steps are data preparation and data synthesis. Both steps can be automated by suppliers.
What are synthetic data generation tools?
The synthetic data generation process is a two step process. You need to prepare data before synthesis. There are various vendors in the space for both steps.
If you want to learn leading data preparation tools, you can check our list about top 152 data quality software.
If you are looking for a synthetic data generator tool, feel free to check our sortable list of synthetic data generator vendors.
Synthetic data is not the only way to secure data; feel free to read our other security and privacy-related articles to minimize the impact of potential data breaches or improve your company’s compliance:
- Data Masking: Protect your enterprise’s sensitive data
- AI Security: Defend against AI-powered cyberattacks
Synthetic Data FAQ
In what fields is synthetic data commonly used?
A: Synthetic data is widely used in healthcare, finance, autonomous vehicles, gaming, cybersecurity, and any field where data privacy is crucial or real data is scarce or biased.
What are the limitations of synthetic data?
Limitations include potential inaccuracies if the synthetic data doesn’t accurately reflect real-world complexities, the risk of introducing bias, and the need for sophisticated algorithms and expertise to generate high-quality synthetic data.
How does synthetic data relate to data privacy regulations like GDPR?
Synthetic data can help comply with data privacy regulations like GDPR by ensuring that the data used for analysis or AI training doesn’t contain personally identifiable information. However, compliance also depends on the methodology used to generate the synthetic data.
Can synthetic data replace real data?
While synthetic data can supplement real data in many scenarios, especially where privacy or data scarcity is a concern, it’s not always a complete replacement. The decision to use synthetic versus real data depends on the specific use case, the quality of the synthetic data, and the criticality of accuracy.
If you have further questions, reach us:

Cem has been the principal analyst at AIMultiple since 2017. AIMultiple informs hundreds of thousands of businesses (as per similarWeb) including 60% of Fortune 500 every month.
Cem's work has been cited by leading global publications including Business Insider, Forbes, Washington Post, global firms like Deloitte, HPE, NGOs like World Economic Forum and supranational organizations like European Commission. You can see more reputable companies and media that referenced AIMultiple.
Throughout his career, Cem served as a tech consultant, tech buyer and tech entrepreneur. He advised businesses on their enterprise software, automation, cloud, AI / ML and other technology related decisions at McKinsey & Company and Altman Solon for more than a decade. He also published a McKinsey report on digitalization.
He led technology strategy and procurement of a telco while reporting to the CEO. He has also led commercial growth of deep tech company Hypatos that reached a 7 digit annual recurring revenue and a 9 digit valuation from 0 within 2 years. Cem's work in Hypatos was covered by leading technology publications like TechCrunch and Business Insider.
Cem regularly speaks at international technology conferences. He graduated from Bogazici University as a computer engineer and holds an MBA from Columbia Business School.
To stay up-to-date on B2B tech & accelerate your enterprise:
Follow onNext to Read
Synthetic Data for Healthcare: Benefits & Case Studies in 2024
Synthetic Data to Improve Deep Learning Models in 2024
Synthetic Data Statistics: Benefits, Vendors, Market Size [2024]
It is SimPy not SymPy – the two are very different..
Hi Jaiber, thank you for your comment, we also notice a lot of typos on the web.
However, we had mentioned above that SymPy can help generate synthetic data with symbolic expressions, I clarified the wording a bit more. That seems correct to me.
I believe you mean that SimPy discrete event simulation can be used to create synthetic data, too, right? If you have an example, happy to add, too.
How I can generate synthetic data given that I want the data on the tail to follow a specific distribution and data on the head of follows a different distribution?
You could combine distributions to create a single distribution which you can use for data generation.
Comments
Your email address will not be published. All fields are required.