Synthetic data is widely used across various domains, including machine learning, deep learning, generative AI (GenAI), large language models, and data analytics. According to Gartner, by 2030, synthetic data use will outweigh real data in AI models.1
For some business leaders and executives, synthetic data may still be a foreign concept because of its differences from real data. We explain synthetic and real data, their differences, benefits, and challenges.
What is synthetic data? How is it created?
Synthetic data is data that has been artificially created by computer algorithms, as opposed to real data that has been collected from natural events.
Although there are other ways to generate synthetic data, AI-generated synthetic data is produced by AI that is trained on complex real-world data, by the power of deep learning algorithms. The merit in using generative AI is that it is capable of automatically detecting patterns, structures, correlations, etc. within real data, and then learning how to generate brand new data with the same patterns. You can see the structural similarity in Figure 1 below.
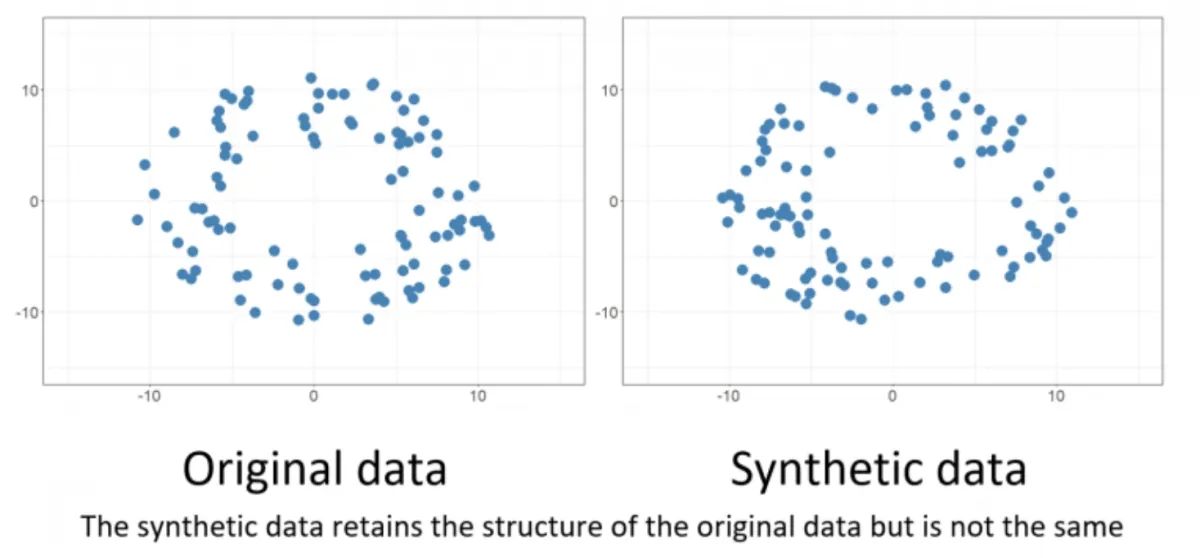
One popular method is to generate data using a computer algorithm that mimics the behavior of real-world data. This approach can be used to create synthetic data sets that are similar to real data sets in terms of their distribution and variability. Another common method for creating synthetic data is to use a random number generator to create data that is uniform and has no correlation.
For more on what is synthetic data and its benefits, you can check our article.
The benefits of synthetic data over real data
Here is a brief comparison between synthetic data vs real data. To get more detailed information, please see the section after the table.
Aspect | Synthetic Data | Real Data |
---|---|---|
Definition | Data generated to simulate real-world data patterns. | Actual data collected from real-world sources. |
Source | Created through algorithms, simulations, or pattern generation. | Collected directly from user interactions, transactions, or events. |
Data Privacy | High, as it contains no actual sensitive information. | Can contain sensitive, identifiable information. |
Accuracy | May approximate real data patterns but lacks true authenticity. | High, as it represents real events and interactions. |
Risk of Re-identification | Low, as it doesn’t include real user information. | High, depending on the presence of personally identifiable information (PII). |
Data Utility | Effective for testing, model training, and simulations. | Essential for production and insights requiring actual trends. |
Cost of Acquisition | Generally lower, as it can be generated programmatically. | Higher, often requiring data collection efforts and compliance. |
Bias and Representativeness | Potentially less biased if designed well, but depends on input patterns. | May contain biases reflective of actual populations and collection methods. |
Scalability | Easily scalable, as it can be generated in large quantities. | Limited by the availability and accessibility of real data. |
Use Cases | Ideal for prototyping, algorithm testing, and privacy-preserving analytics. | Essential for production, regulatory reporting, and detailed insights. |
1. Overcomes regulatory restrictions: The most important benefit of synthetic data over real data is that it avoids regulatory restrictions on real data. Synthetic data can replicate all important statistical properties of real data without exposing the latter, eliminating any concern about privacy regulations. This feature thus further enables:
- Privacy preservation: It is hard to sustain privacy in classic anonymization methods while preserving the usefulness of the real dataset. You have to choose either protecting the privacy of the people while diminishing the effectiveness of that data or getting usefulness while renouncing privacy. With synthetic data, the privacy/usefulness dilemma is resolved since there is no real data that you must protect against leaking.
- Resistance to reidentification: Real data removes certain information to satisfy anonymization. Yet, reidentifying the data source is still highly possible. As a study shows, sharing only 3 bank transaction information per customer, with the merchant and the date of the transactions, makes 80% of customers identifiable.3
- Aptitude for Innovation and Monetization: As there are no privacy concerns for synthetic data, it is possible to share these datasets with third parties for innovation research and to use them as a monetization tool.
2. Streamlines simulation: Synthetic data enables the creation of data to simulate conditions that have not yet been encountered. Where real data does not exist, synthetic data is the only solution. For instance, automotive firms may not gather real data for all possible situations to train smart cars.
3. Avoids statistical problems: Synthetic data can eliminate problems like item nonresponse and skip patterns. By setting rules and dependencies during generation, responses can be logically consistent and complete across all survey items.
4. Speeds up the process: Synthetic data can be generated much faster than real data can be collected, saving time and ensuring agility and competitiveness in the market.
5. Achieves higher consistency: Synthetic data can be more uniform and consistent than real data, which can be variable due to its natural origins. This uniformity makes synthetic data more suitable for performing accurate analyses on synthetic datasets.
6. Ensures easy manipulation: Synthetic data is easier to manipulate in controlled ways than real data, enabling precise testing and training of machine learning models. It can be generated at scale with specific traits or biases, helping improve model performance across various applications.
7. Increases cost-effectiveness: Synthetic data can be more cost-effective than real data. Of course, creating synthetic data is not free. The main cost of synthetic data is an upfront investment in building the simulation. However, real data enforce timely and financial costs every time a new data set is required or an existing one is revised.
8. Facilitates AI/ML training: Synthetic data is more enriching for teaching AI/ML models as it has no regulations restricting real data. Also, it has a higher capacity to create more data, feeding AI much more to learn. For more detail, check our article on the use of synthetic data to improve deep learning models.
Some challenges with using synthetic data against real data
Besides a variety of benefits, there are some challenges with using synthetic data.
- Biased or deceptive results: Synthetic data can be misleading, limited or discriminatory due to its lack of variability and correlation.
- Lack of accuracy: Another challenge with synthetic data is that it is often created using a computer algorithm, which may not always be accurate. As a result, synthetic data can occasionally produce inaccurate results.
- Time-consuming steps: Relatedly, synthetic data requires additional verification steps, such as comparing model results with human-annotated, real-world information. Such efforts take time to complete and prolong the projects.
- Losing outliers: Synthetic data may not cover some of the outliers present in the original dataset because it can only mimic but not replicate real data. However, outliers can be relevant for some research.
- Dependency on the real data: Synthetic data quality often depends on the real model and the dataset that have been developed for creating synthetic data. Without a desirable and qualitative real dataset, various synthetic datasets that are generated in huge amounts by using the original dataset will end up functioning ineffectively and sometimes even incorrectly.
- Consumer skepticism: As synthetic data use increases, businesses can face consumer skepticism, such as questioning the credibility of the data for reaching conclusions and making products. Consumers might demand assurance of the transparency of the data generation techniques and the privacy of their information.
Despite these challenges, synthetic data remains an important tool for data analysis. When used correctly, synthetic data can provide valuable insights into the behavior of real-world data.
Which type of data should be used for which specific application?
As we discussed in the section on the benefits of synthetic data, there are various application areas where it can be used effectively—sometimes even exclusively—while real data may be impractical or unavailable.
When to Use Synthetic Data
Synthetic data is especially useful in scenarios where real data is inaccessible, sensitive, or constantly evolving. One such example is radioactive data sets. The term “radioactive” refers to data that is rapidly changing, difficult to version, and nearly impossible to maintain in its original form. This could be due to rapid dataset growth, frequent updates, or a highly dynamic nature. In such cases, synthetic data offers a manageable and scalable alternative.
When Real Data Is Preferred
Real data should be prioritized when the objective is to reproduce the exact distribution of real-world phenomena. This includes applications where statistical authenticity, detailed pattern recognition, or regulatory compliance is critical.
Moreover, for tasks that involve studying the correlation between variables, real data is often superior. Unlike synthetic datasets, which may lack complex inter-variable relationships, real-world data reflects natural dependencies and can offer more valid insights. Synthetic data may also be harder to interpret and less reflective of actual behavior patterns.
Choosing the Right Data for the Task
Ultimately, the decision between synthetic and real data hinges on the specific goals and constraints of your application.
- If accuracy and representativeness are paramount, real data is usually the better option.
- If speed, scalability, or data availability are key drivers—and especially when handling sensitive or non-existent data—synthetic data may be the optimal choice.
Synthetic data vs real data examples
Automotive & Transportation: Self-Driving Car Development
Synthetic & Real Data Use
Self-driving companies like Waymo and Tesla use synthetic data to simulate rare or extreme driving scenarios (e.g., accidents, severe weather) that are difficult to capture with real-world testing. Real-world data is also essential, with millions of miles driven on public roads to ensure the AI system is prepared for typical driving conditions.
Challenges
Synthetic data may not fully capture the complexity and unpredictability of real-world conditions, potentially leading to performance gaps when the AI encounters novel situations in the real world. This gap is one reason for performance issues seen in autonomous driving systems like Tesla’s Autopilot in unfamiliar environments or edge cases.
Collecting real-world driving data is costly, time-consuming, and poses safety risks. Furthermore, real-world data often lacks enough edge cases, such as rare but critical accident scenarios, making it difficult to train AI comprehensively. Privacy concerns also arise when recording data from real drivers and pedestrians.
Healthcare & Life Sciences: Clinical Trials and Patient Predictions
Synthetic & Real Data Use
Synthetic health data is used by companies like Synthea to create realistic patient records for AI model training, allowing researchers to build predictive models without compromising patient privacy. Real-world clinical data is crucial for validating AI predictions and ensuring the model’s accuracy with actual patient outcomes.
Challenges
Synthetic data might not include rare or edge-case health conditions, leading to models that miss crucial correlations or fail to recognize uncommon diseases.
Medical data is heavily regulated (HIPAA, GDPR), making it difficult to access and share for research. Data scarcity is another issue—certain diseases or conditions have limited available real-world records, which restricts AI model development. Additionally, biases in real-world data (e.g., underrepresentation of certain demographics) can lead to unfair AI predictions.
Finance & Banking: Fraud Detection
Synthetic & Real Data Use
Banks like HSBC and J.P. Morgan generate synthetic transaction data to simulate fraud scenarios and train models without exposing sensitive customer information. Real data is necessary to validate the models, especially when assessing the efficacy of fraud detection algorithms in detecting actual fraudulent activities.
Challenges
Synthetic data may not fully represent rare fraudulent events, making it harder to simulate sophisticated fraud techniques that occur infrequently in real life.
Real financial data contains sensitive personal and transaction information, making it difficult to use without violating privacy laws (e.g., GDPR, CCPA). Additionally, fraud datasets are often highly imbalanced, with very few fraud cases compared to legitimate transactions, making AI training less effective.
Government & Public Sector: Census and Policy Analysis
Synthetic & Real Data Use
Governments like the U.S. Census Bureau and Statistics Netherlands use synthetic data for policy analysis and research, especially when real data is sensitive or difficult to access due to privacy concerns. However, actual census and survey data are crucial for accurate policy-making, especially when crafting targeted programs for specific demographics.
Challenges
Synthetic data may fail to capture complex relationships that exist in real data, such as the impact of local socio-economic conditions on demographic behaviors, leading to less accurate policy analysis.
Real census data is often incomplete due to low response rates, missing information, or biased sampling, which can result in inaccurate insights for policymakers. Additionally, sharing real demographic data poses privacy risks, requiring anonymization methods that can degrade data quality.
Retail & E-Commerce: Customer Behavior Modeling
Synthetic & Real Data Use
Retailers like Amazon and Walmart use synthetic data to simulate customer behaviors, preferences, and transaction histories for AI model training in areas like dynamic pricing, recommendations, and fraud detection. Real transactional data is necessary for validating AI models and understanding actual customer behaviors and market trends.
Challenges
Synthetic data may not always capture unanticipated changes in consumer behavior, such as emerging trends or external market disruptions, which may require real data for optimal model performance.
Real-world retail data is subject to privacy regulations (e.g., CCPA, GDPR) and consumer consent laws, limiting how it can be collected and used. Data silos between different retail platforms also make it difficult to gain a holistic view of customer behavior, reducing AI effectiveness.
Manufacturing & Industrial IoT: Predictive Maintenance
Synthetic & Real Data Use
Manufacturers like Siemens and General Electric (GE) use synthetic data generated from simulations of machine operations to predict failures and plan maintenance schedules. Real sensor data is needed to validate the predictive models and ensure the maintenance predictions align with actual machine performance.
Challenges
If the synthetic data oversimplifies the behavior of machines, the resulting AI models might not perform well under complex, real-world conditions.
Real-world machine failure data is scarce, as breakdowns are rare events. This makes it difficult to train AI models effectively. Additionally, collecting and storing large volumes of sensor data is expensive, and machine data varies significantly between different environments, making AI generalization harder.
AI/ML Model Training: Overcoming Data Scarcity and Privacy Concerns
Synthetic & Real Data Use
AI models across industries use synthetic data to overcome data scarcity, particularly in sectors like healthcare and finance, where data may be sensitive or difficult to access. Companies like Zebra Medical Vision and DeepMind utilize synthetic data for training AI models for medical imaging and health predictions. Real data is essential to ensure that AI models reflect accurate patterns and real-world performance.
Challenges
Over-reliance on synthetic data can lead to overfitting, where models perform well on synthetic data but fail in real-world applications due to differences in data distributions.
Real data collection is expensive and time-consuming, often requiring manual labeling (e.g., medical imaging datasets). Additionally, data bias can be a significant issue, as real datasets may not always represent all demographic or geographic variations, leading to biased AI predictions.
Is real data and human-generated data the same?
Human-generated data and real data are related but not interchangeable terms. Human-generated data refers specifically to information created or input by people, such as survey responses, social media posts, or written feedback. In contrast, real data encompasses any data collected from real-world events, which can be produced either by humans or by machines (e.g., IoT sensors, GPS logs, system logs).
While there is overlap, such as a user submitting a product review that reflects an actual experience, not all human-generated data is necessarily grounded in real events, and not all real data is human-generated. For example, fictional stories or hypothetical examples are human-generated but not “real” in the empirical sense. Conversely, machine-generated logs of real transactions or environmental readings are real data but not human-generated.
Key differences:
- Distinctions: Machine-generated system logs are real but not human-generated; fictional blog posts are human-generated but not real.
- Human-generated data: Created intentionally by people (e.g., text, images, survey answers).
- Real data: Reflects actual events or behaviors, collected passively or actively.
- Overlap: A review describing an actual purchase is both human-generated and real.
For more on synthetic data
If you want to gain more insight into synthetic data, its benefits, use cases, and tools, you can check our other articles on the topic:
- Synthetic Data Generation
- Top 20 Synthetic Data Use Cases & Applications
- Synthetic Data Tools Selection Guide & Top 7 Vendors
- Synthetic Data Applications to Enable Finance Innovation
Comments
Your email address will not be published. All fields are required.