We benchmarked 7 publicly available synthetic data generators sourced from 4 distinct providers, utilizing a holdout dataset comprising 70,000 samples, with 4 numerical and 7 categorical features, to evaluate their performance in replicating real-world data characteristics.
Synthesizer | Best For | |
---|---|---|
1. | ![]() YData |
Statistically most accurate |
2. | ![]() MOSTLY AI |
Easy to use |
3. | ![]() Synthetic Data Vault |
Open source applications |
4. | ![]() Gretel |
Custom data generation |
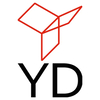
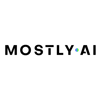
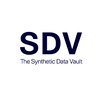
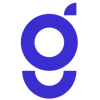
Below, you can see the benchmark results where we statistically compare the synthetic data generators.
You can find more detailed information about the metrics in the methodology.
Synthetic data generation is key in ensuring data quality, especially in maintaining privacy. By design, synthetic data mimics the statistical properties of real data without exposing sensitive information. However, if synthetic data can be reverse-engineered to reconstruct the original dataset, it undermines its fundamental purpose of safeguarding privacy.
Large Language Models (LLMs) are among the largest producers of synthetic data. Numerous benchmarks for state-of-the-art (SOTA) LLMs rely on these models to generate test cases for evaluating other LLMs. Moreover, LLMs themselves are often trained on synthetic data, leveraging the diversity and scale of artificial datasets to improve their performance.
As in most AI-related topics, deep learning is integral to synthetic data generation as well. Synthetic data created by deep learning algorithms is also being used to improve other deep learning algorithms. AIMultiple explains synthetic data generation techniques, as well as best practices.
Why is synthetic data important for businesses?
Synthetic data is important for businesses for three reasons:
- privacy,
- product testing,
- and training machine learning algorithms.
Industry leaders also started to discuss the importance of data-centric approaches to AI/ML model development, to which synthetic data can add significant value.
When is synthetic data used?
Businesses face a trade-off between data privacy and data utility when selecting a privacy-enhancing technology. Therefore, they need to determine the priorities of their use case before investing. Synthetic data does not contain any personal information; it is sample data with a distribution similar to the original data.
Though synthetic data can be less useful than real data in some cases, synthetic data can also be almost as valuable as real data. For example, a team at Deloitte Consulting generated 80% of the training data for a machine-learning model by synthesizing data. The resulting model accuracy was similar to that of a model trained on real data.
Synthetic data generation can help build accurate machine-learning models, especially when companies need data to train machine-learning algorithms and their training data is highly imbalanced (e.g., more than 99% of instances belong to one class).
See the list of synthetic data use cases.
How do businesses generate synthetic data?
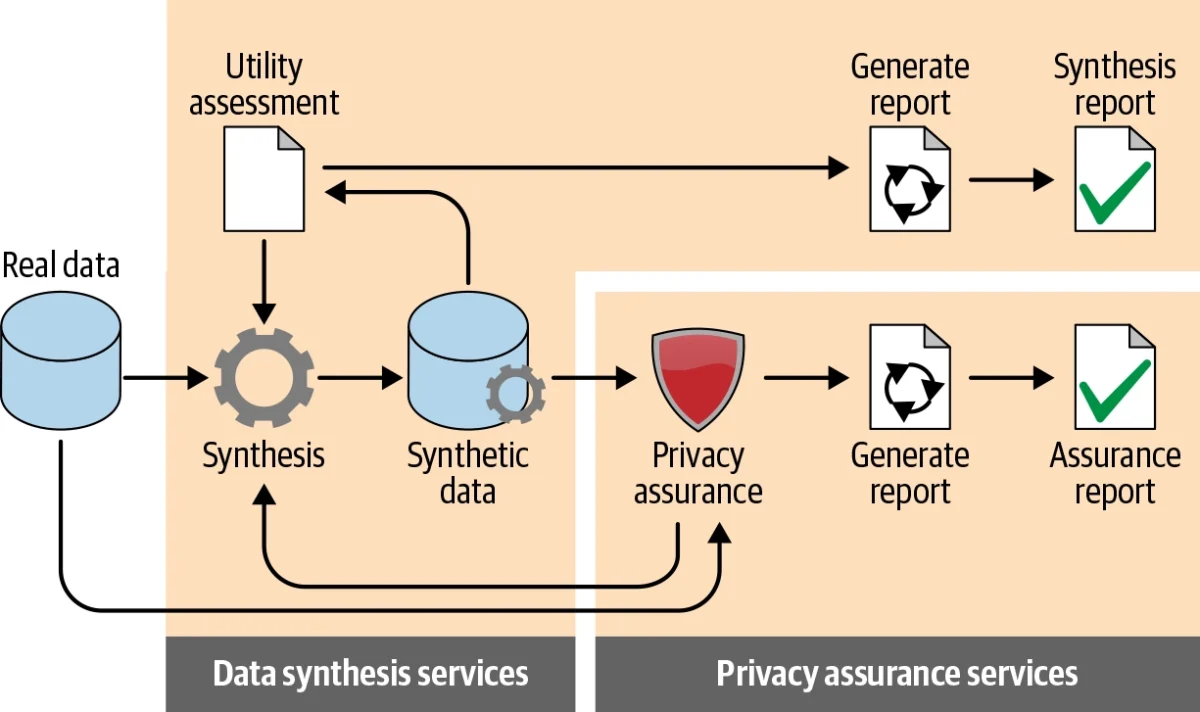
Businesses can prefer different methods such as decision trees, deep learning techniques, and iterative proportional fitting to execute the data synthesis process. They should choose the method according to synthetic data requirements and the level of data utility that is desired for the specific purpose of data generation.
After data synthesis, they should assess the utility of synthetic data by comparing it with real data. The utility assessment process has two stages:
- General purpose comparisons: Comparing parameters such as distributions and correlation coefficients measured from the two datasets
- Workload-aware utility assessment: comparing the accuracy of outputs for the specific use case by performing analysis on synthetic data
What are the techniques of synthetic data generation?
Generating according to distribution
For cases where real data does not exist but the data analyst has a comprehensive understanding of how the dataset distribution would look, the analyst can generate a random sample of any distribution, such as Normal, Exponential, Chi-square, t, lognormal, and Uniform. In this technique, the utility of synthetic data varies depending on the analyst’s degree of knowledge about a specific data environment.
Fitting real data to a known distribution
If there is real data, then businesses can generate synthetic data by determining the best-fit distributions for given real data. If businesses want to fit real data into a known distribution and know the distribution parameters, businesses can use the Monte Carlo method to generate synthetic data. The method is a computational technique that uses random sampling and statistical modeling to solve problems that may be deterministic in principle but are too complex for direct analytical solutions. The steps are:
- Define the Problem: Specify the problem to be solved, often involving parameters with known or assumed distributions.
- Generate Random Inputs: Use random number generation to create inputs, often based on a probability distribution.
- Run the Model: Perform simulations using these inputs to evaluate the outcome of the system or process.
- Aggregate Results: Collect the results of all simulations and calculate statistical measures such as averages, variances, or probabilities.
The Monte Carlo method helps find the best fit for synthetic data, but it may not always meet business needs. Machine learning models like decision trees can model complex, non-classical distributions in such cases. This enables synthetic data generation to be highly correlated with original data. However, ML models risk overfitting, which can reduce their ability to generalize and predict future observations reliably.
Businesses can use hybrid synthetic data generation for cases where only some part of real data exists. In this case, analysts generate one part of the dataset from theoretical distributions and generate other parts based on real data.
Using deep learning
Deep generative models such as Variational Autoencoder (VAE) and Generative Adversarial Network (GAN) can generate synthetic data.
Variational Autoencoder
VAE is an unsupervised method in which the encoder compresses the original dataset into a more compact structure and transmits data to the decoder. Then, the decoder generates an output that represents the original dataset. The system is trained by optimizing the correlation between input and output data.
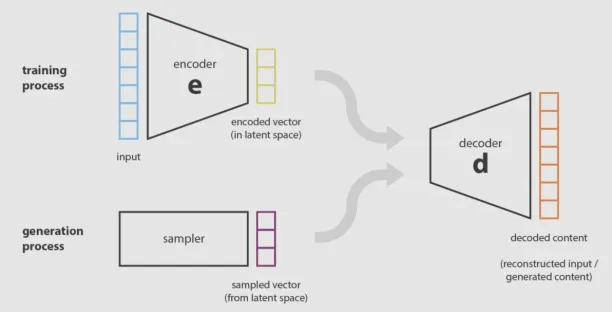
Generative adversarial network
In the GAN model, two networks, a generator and a discriminator, train the model iteratively. The generator takes random sample data and generates a synthetic dataset. The discriminator compares synthetically generated data with a real dataset based on conditions that were set before.
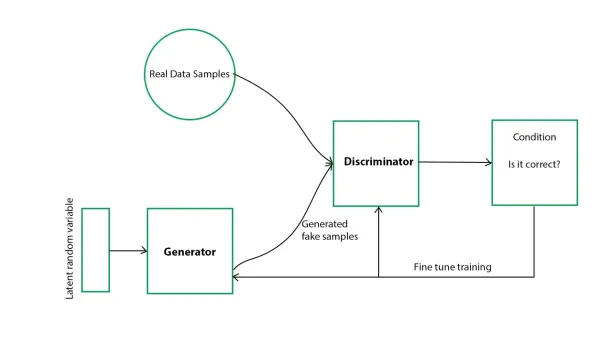
How do you generate synthetic data in Python?
There are several ways to generate synthetic data for simple tasks in Python, and with some effort, these methods can also be adapted for complex tasks.
1. Basic random data generation using NumPy
import numpy as np
# Generate 100 random integers between 0 and 10
random_integers = np.random.randint(0, 11, size=100)
# Generate 50 random floating-point numbers between 0 and 1
random_floats = np.random.rand(50)
2. Generating data with specific distributions using NumPy
import numpy as np
# Generate 100 values from a normal distribution (mean 0, standard deviation 1)
normal_data = np.random.normal(loc=0, scale=1, size=100)
# Generate 50 values from a uniform distribution between -1 and 1
uniform_data = np.random.uniform(low=-1, high=1, size=50)
3. Generating realistic data using faker library
from faker import Faker
fake = Faker() # You can also do Faker('fr_FR') for french or Faker('de_DE') for german
# Generate a fake name
name = fake.name()
# Generate a fake address
address = fake.address()
# Generate a fake email
email = fake.email()
# Generate a fake date
date = fake.date()
# Generate a fake credit card number
credit_card = fake.credit_card_number()
4. Generating data for classification and regression using scikit-learn
from sklearn.datasets import make_classification
from sklearn.datasets import make_regression
# Generate a synthetic classification dataset
X_classification, y_classification = make_classification(
n_samples=100,
n_features=5,
n_informative=3,
n_redundant=1,
random_state=42
)
# Generate a synthetic regression dataset
X_regression, y_regression = make_regression(
n_samples=100,
n_features=4,
noise=0.1,
random_state=42
)
What are the best practices for synthetic data generation?
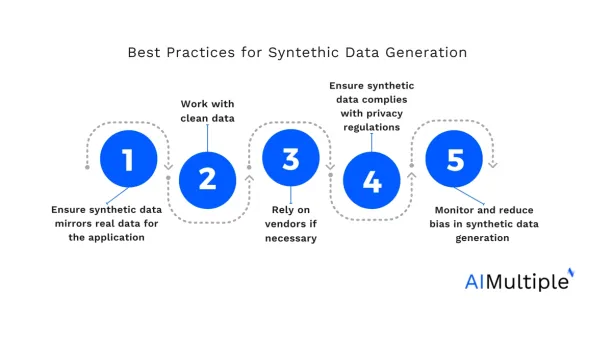
1-Ensure that synthetic data mirrors real data for the application
The utility of synthetic varies depending on the technique you use while generating it. You need to analyze their use case and decide if the generated synthetic data fits the specific use case.
Real-life example:
JP Morgan has applied synthetic data in finance to generate accurate financial models while safeguarding customer privacy. Their approach includes testing synthetic data to ensure that it reflects the real characteristics of their financial data sets. This is particularly important when using synthetic data to train fraud detection algorithms, where the synthetic data must behave like real transactions to identify fraudulent patterns. 4
2-Work with clean data
Clean data is an essential requirement of synthetic data generation. If you don’t clean and prepare data before synthesis, you can have a garbage-in, garbage-out situation. In the data preparation process, make sure you apply the following principles:
- Data cleaning: Eliminating inaccurate, improperly formatted, redundant, or lacking data from a dataset
- Data harmonization: Synthesizing data from several sources and providing customers with a comparable understanding of information from various research.
Real-life example:
The Institute for Informatics, Data Science, and Biostatistics (I2DB) at Washington University in St. Louis adopted the MDClone platform in 2018. Through a landmark study, they confirmed that synthetic data can yield the same analytical outcomes as real data while also preserving privacy. This initiative is part of the university’s broader strategy to enhance data-driven research by providing secure and innovative resources to the academic community.5
Before creating synthetic data, patient records are cleaned—this involves removing errors and duplicates and ensuring data consistency. By cleaning and harmonizing Electronic Health Records (EHRs) from different departments, MDClone ensures that synthetic versions of these records maintain the utility of real data for medical research while protecting patient privacy.
3- Rely on vendors if necessary
Identify your organization’s synthetic data capabilities and outsource based on capability gaps. The two important steps are data preparation and data synthesis. Suppliers can automate both steps.
4-Ensure that synthetic data complies with privacy regulations
When generating synthetic data, ensuring the privacy of individuals whose data is used to create the synthetic dataset is critical. Compliance with data privacy regulations, such as GDPR (General Data Protection Regulation) and HIPAA, is mandatory. Synthetic data should be completely detached from any real individual, and there should be no way to trace synthetic data back to the original records.
Real-world datasets often contain sensitive information, especially in the healthcare, finance, and telecommunications industries. If synthetic data is not generated with strong privacy measures, it could lead to legal repercussions and customer trust issues.
5-Bias Mitigation
Synthetic data generation should aim to avoid introducing or perpetuating biases present in real-world datasets. This involves monitoring for potential bias in attributes like race, gender, or socioeconomic status, possibly leading to discriminatory outcomes if left unchecked. Bias mitigation is crucial for ensuring fair and equitable models.
Biased data leads to biased models, which in turn can perpetuate social inequalities. For instance, an AI hiring tool trained on biased data may disproportionately reject candidates from certain demographic groups. Ensuring that synthetic data is balanced and unbiased helps develop fairer AI models.
Methodology
We conducted the benchmark to evaluate the performance of 7 publicly available synthetic data generators using a holdout dataset. The dataset consists of 70,000 samples and includes 4 numerical and 7 categorical features.
Data Preparation
The dataset was first homogenized, meaning the distributions of features were consistent across the dataset, eliminating the need for additional preprocessing, such as normalization or handling missing values. To prepare the data for training and evaluation, we performed the following steps:
- Shuffling: The dataset was randomly shuffled to ensure that the data points were distributed uniformly and to avoid any ordering bias.
- Splitting: The shuffled dataset was split roughly into two equal halves:
- Training Data: 35,000 samples were used to train the synthetic data generators.
- Holdout Data: 35,000 samples reserved for evaluation to assess the quality of the generated synthetic data against unseen real data.
Since the dataset was homogenized, the distributions of features in the training and holdout sets were similar, ensuring a fair comparison.
Training Synthetic Data Generators
We selected 7 synthetic data generators for this benchmark: YData, Mostly AI, Gretel, and 4 generators from Synthetic Data Vault. The training process for each generator was as follows:
- SDV and YData: We used the SDKs and models provided by SDV and YData, configured with their default settings to ensure consistency and reproducibility.
- Mostly AI and Gretel: These generators were trained using their platforms, with settings configured as recommended by the providers.
Each generator was trained exclusively on the training data (35,000 samples) to generate synthetic datasets that mimic the characteristics of the real data.
Evaluation
After training, each synthetic data generator produced a synthetic dataset. We evaluated the quality of these synthetic datasets by comparing them against both the training data (to assess how well the generators captured the training distribution) and the holdout data (to evaluate generalization to unseen data).
We evaluated the synthetic data quality using three metrics:
Correlation Distance (Δ)
Measures the absolute difference between correlation matrices of numerical features in real and synthetic datasets, assessing how well relationships (e.g., between house size and energy consumption) are preserved.
- Range: 0 (perfect) to 1 (worst).
- Purpose: Ensures structural relationships are maintained, vital for tasks like regression.
Kolmogorov-Smirnov Distance (K)
Measures the maximum difference between cumulative distribution functions (CDFs) of numerical features, evaluating how well their marginal distributions (e.g., distribution of customer ages) are captured.
- Range: 0 (identical) to 1 (completely different).
- Purpose: Ensures realistic numerical distributions, which are crucial for simulations or statistical analysis.
Total Variation Distance (TVD)
Measures the difference between probability distributions of categorical features, calculated as half the sum of absolute differences (e.g., distribution of customer regions).
- Range: 0 (identical) to 1 (completely different).
- Purpose: Assesses how well categorical distributions are captured, important for tasks like classification.
Model-based evaluation
Statistical metrics are useful for gaining initial insights into synthetic data quality. However, the true evaluation should be model-based, focusing on the practical utility of synthetic data for model training. It is crucial to measure how synthetic data benefits model performance.
In our evaluation, we assessed the quality of synthetic data generated using the default settings of the synthesizers and checked for overfitting on the original training data.
We also investigated whether augmenting training data with synthetic data could improve accuracy. However, our tests, involving incrementally adding synthetic data from 10% up to 100% of the training set size, showed that model accuracy consistently decreased even with the smallest addition.
This decline correlated with the volume of synthetic data added, indicating increased noise introduction. Notably, synthesizers producing higher-quality data (lower error rates in our benchmark) introduced less noise and resulted in smaller performance drops.
Customizing these generators for specific needs in practical applications could produce significantly better results. Furthermore, we have omitted the performance results of models trained on augmented data. This is because those models were also tested using default configurations without hyperparameter tuning. Presenting such results would not constitute a fair comparison, given that real-world deployment necessitates model optimization.
In model-based evaluations, particularly when using a partial augmentation approach blending synthetic with real data, careful consideration of the synthetic data volume is vital. Adding too much synthetic data can introduce noise and diminish the model’s ability to learn effectively from the real data, potentially leading to an inaccurate assessment of synthetic data’s true benefits.
Synthetic Data FAQ
What is synthetic data?
Synthetic data is artificial data created by using different algorithms that mirror the statistical properties of the original data but do not reveal any information regarding real-world events or people.
For example, data produced by computer simulations would qualify as synthetic data. This includes applications like music synthesizers, medical imaging, economic models, and flight simulators, where the outputs mimic real-world phenomena but are entirely generated through algorithms.
In what fields is synthetic data commonly used?
Synthetic data is widely used in healthcare, finance, autonomous vehicles, gaming, cybersecurity, and any field where data privacy is crucial or real data is scarce or biased.
What are the limitations of synthetic data?
Limitations include potential inaccuracies if the synthetic data doesn’t accurately reflect real-world complexities, the risk of introducing bias, and the need for sophisticated algorithms and expertise to generate high-quality synthetic data.
How does synthetic data relate to data privacy regulations like GDPR?
Synthetic data can help comply with data privacy regulations like GDPR by ensuring that the data used for analysis or AI training doesn’t contain personally identifiable information. However, compliance also depends on the methodology used to generate the synthetic data.
Can synthetic data replace real data?
While synthetic data can supplement real data in many scenarios, especially where privacy or data scarcity is a concern, it’s not always a complete replacement. The decision to use synthetic versus real data depends on the specific use case, the quality of the synthetic data, and the criticality of accuracy.
External Links
- 1. Data Anonymization | The Hashbrown Blog.
- 2. 生成式AI核心技术详解:从GANs到Transformers-腾讯云开发者社区-腾讯云.
- 3. Generative Adversarial Network. Basics of GAN | by DARSHAN DILIPBHAI PATEL | Medium. Medium
- 4. Synthetic Data for Real Insights.
- 5. MDClone | Informatics, Data Science & Biostatistics | Washington University in St. Louis.
Comments
Your email address will not be published. All fields are required.