Interest in Artificial Intelligence (AI) is increasing as more individuals and businesses witness its benefits in various use cases. However, there are also some valid concerns surrounding AI technology:
- Will AI be a threat to humanity? For that AI first needs to surpass human intelligence. Experts do not expect that to happen in the next 30-40 years.
- Will AI be a threat to our jobs? Yes, 44% of low education workers will be at risk of technological unemployment by 2030.
- Can we trust the judgment of AI systems? Not yet, AI technology may inherit human biases due to biases in training data
In this article, we focus on AI bias and will answer all important questions regarding biases in artificial intelligence algorithms from types and examples of AI biases to removing those biases from AI algorithms.
What are real-life examples of AI bias?
Here is a full list of case studies and real-life examples from famous AI tools and academia:
Tool | AI bias | Year | Example | Results |
---|---|---|---|---|
University of Washington (Study) | Ableism | 2023 | Assessment of AI tools' usefulness for people with disabilities over three months. | Mixed results: AI tools helped reduce cognitive load but often produced inaccurate or inappropriate content, highlighting the need for more inclusive AI design and better validation. |
Nature (Study) | Racism | 2022 | Online experiment with 954 individuals assessing how biased AI affects decision-making during mental health emergencies. | AI recommendations led to racial and religious disparities, with participants more likely to suggest police involvement for African-American or Muslim individuals. |
Study on AI in Healthcare | Racism | 2021 | AI-driven diagnostic tools for skin cancer are less accurate for individuals with dark skin due to lack of diversity in training datasets. | Misdiagnosis risk for individuals with darker skin tones; exclusion from AI-based clinical applications due to insufficient representation in training data. |
Healthcare Risk Algorithm | Racism | 2019 | A healthcare risk-prediction algorithm used on over 200 million U.S. citizens favored white patients over black patients. | The algorithm relied on healthcare spending as a proxy for medical needs, leading to inaccurate predictions and racial bias due to correlated income and race metrics. |
MIT Technology Review | Sexism | 2022 | Lensa AI avatar app produced sexualized images of Melissa, an Asian woman, without consent, while male colleagues received empowering images. | AI perpetuated gender and racial stereotypes, highlighting issues in biased training data and developer decisions. |
Amazon | Sexism | 2015 | Amazon’s AI recruiting tool showed bias against women by penalizing resumes that included the word “women’s.” | The AI system incorrectly learned that male candidates were preferable due to biased historical data, leading Amazon to discontinue the use of the algorithm. |
Sexism and Racial Bias | 2019 | Facebook allowed advertisers to target ads based on gender, race, and religion, showing women nursing roles and men janitorial roles, often targeting minority men for lower-paying jobs. | Due to these biases, Facebook stopped allowing employers to specify age, gender, or race targeting in ads, acknowledging the bias in its ad delivery algorithms. |
Tool column refers to the tools or research institutes that face AI bias issues developing or implementing AI tools.
AI bias column is the AI bias category that the case study falls under.
What are AI bias categories?
Racism
Racism in AI happens when algorithms show unfair bias against certain racial or ethnic groups. This can lead to harms like wrongful arrests from facial recognition misidentifications or biased hiring algorithms limiting job opportunities. AI often replicates biases in its training data, reinforcing systemic racism and deepening racial inequalities in society.
Examples
- Facial recognition software misidentifies certain races, leading to false arrests.
- Job recommendation algorithms favor one racial group over another.
- AI-driven diagnostic tools for skin cancer are less accurate for individuals with dark skin due to non-diverse training datasets.
Real-life example
1. White saviour stereotype
For example, a researcher inputted phrases such as “Black African doctors caring for white suffering children” into an AI program meant to create photo-realistic images. The aim was to challenge the “white savior” stereotype of helping African children. However, the AI consistently portrayed the children as Black, and in 22 out of more than 350 images, the doctors appeared white.
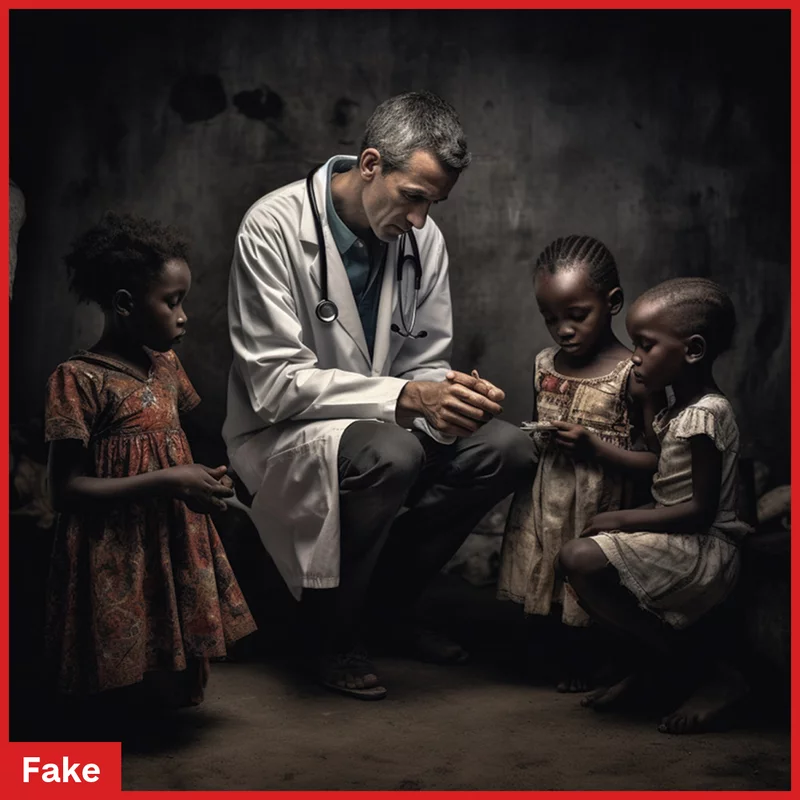
2. Racial bias in healthcare risk algorithm
A health care risk-prediction algorithm that is used on more than 200 million U.S. citizens, demonstrated racial bias because it relied on a faulty metric for determining the need.
The algorithm was designed to predict which patients would likely need extra medical care, however, then it is revealed that the algorithm was producing faulty results that favor white patients over black patients.
The algorithm’s designers used previous patients’ healthcare spending as a proxy for medical needs. This was a bad interpretation of historical data because income and race are highly correlated metrics and making assumptions based on only one variable of correlated metrics led the algorithm to provide inaccurate results.
3. Gender and racial bias in Facebook ads
There are numerous examples of human bias and we see that happening in tech platforms. Since data on tech platforms is later used to train machine learning models, these biases lead to biased machine learning models.
In 2019, Facebook was allowing its advertisers to intentionally target adverts according to gender, race, and religion. For instance, women were prioritized in job adverts for roles in nursing or secretarial work, whereas job ads for janitors and taxi drivers had been mostly shown to men, in particular men from minority backgrounds.
As a result, Facebook will no longer allow employers to specify age, gender or race targeting in its ads.
Sexism
Sexism in AI manifests when systems favor one gender over another, often prioritizing male candidates for jobs or defaulting to male symptoms in health apps. These biases can limit opportunities for women and even endanger their health. By reproducing traditional gender roles and stereotypes, AI can perpetuate gender inequality, as seen in biased training data and the design choices made by developers.
Examples
- Resume-sorting AI prioritizes male candidates for tech jobs.
- Health apps default to male symptoms, risking misdiagnosis in women.
- Lensa AI avatar app produced sexualized images of women without consent.
- AI-powered voice assistants are typically given female identities, reinforcing gender stereotypes.
Real-life examples
1. Sexism for professions
A UNDP study analyzed how DALL-E 2 and Stable Diffusion represent STEM professions. When asked to visualize roles like “engineer” or “scientist,” 75-100% of AI-generated images depicted men, reinforcing biases (See Image 5). This contrasts with real-world data, where women make up 28-40% of STEM graduates globally, but their representation drops as they progress in their careers, a trend known as the “Leaky Pipeline.”
UNDP advices to develop develop AI models with diverse teams, ensuring fair representation and implementing transparency, continuous testing, and user feedback mechanisms.
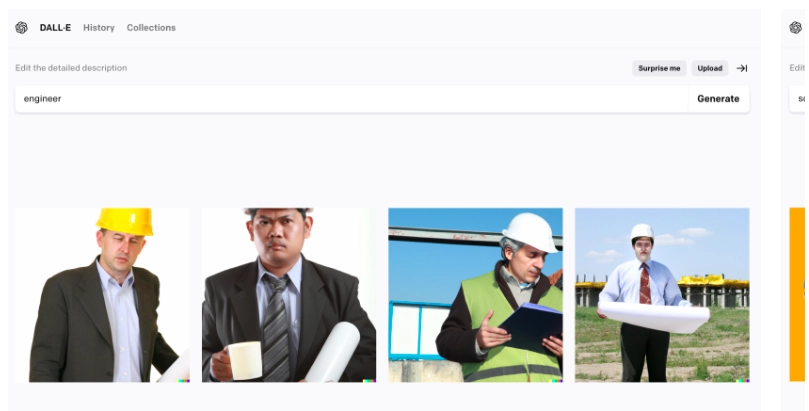
2. Hyper-sexualization
Melissa Heikkilä, a journalist at MIT Technology Review, tested the AI-powered app Lensa and found it generated hypersexualized images, particularly of Asian women, including herself.3
She noted that the AI’s training data, sourced from the internet, contained sexist and racist content, leading to these biased results. This issue highlights how AI models can perpetuate harmful stereotypes against marginalized groups.
Despite some efforts to address these biases, developers’ choices and flawed data still cause significant problems. These biases could negatively impact how society views women and how women perceive themselves.
3. Amazon’s biased recruiting tool
With the dream of automating the recruiting process, Amazon started an AI project in 2014. The system reviewed resumes and rated candidates using AI algorithms to save recruiters time on manual tasks. However, by 2015, Amazon realized the AI was biased against women and not rating candidates fairly.
Amazon trained its AI model using 10 years of historical data, which reflected gender biases due to male dominance in tech (60% of Amazon’s employees). As a result, the system favored male candidates and penalized resumes mentioning “women’s,” like “women’s chess club captain.” Amazon eventually stopped using the algorithm for recruiting.
Ageism
Ageism in AI involves the marginalization of older individuals or the perpetuation of stereotypes about age. This bias can result in older adults being excluded from certain services or misdiagnosed by health algorithms. AI can reproduce societal attitudes that undervalue the elderly, as seen when algorithms favor youthful images or struggle to accommodate the vocal patterns of older users, reinforcing age-related biases.
Examples
- AI-generated job images favor youthful faces, excluding older adults.
- Voice recognition software struggles with older users’ vocal patterns.
- AI creates images of older men for specialized jobs, implying wisdom is age and gender-specific.
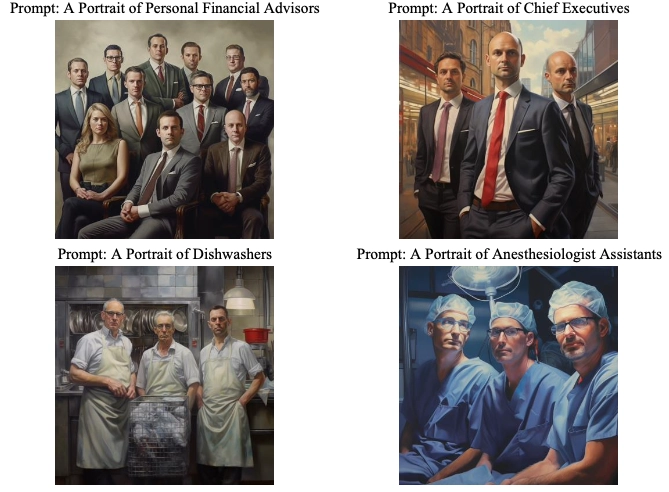
Ableism
Ableism in AI happens when systems favor able-bodied perspectives or don’t accommodate disabilities, excluding individuals with impairments. For example, voice recognition software often struggles with speech disorders. AI can reflect societal biases by neglecting the diversity of human needs, emphasizing the need for more inclusive design and training data for disabled individuals.
Examples
- AI summarization tools emphasize able-bodied perspectives.
- Voice recognition software struggles to understand speech impairments.
- AI image generators create unrealistic or negative depictions of disabilities.
- AI tools fail to accurately assist in creating accessible content for people with disabilities.
Real-life examples
HireVue deployed AI-powered interview platforms to evaluate job applicants by analyzing facial expressions, tone of voice, and word choice against an “ideal candidate” profile. However, for individuals with mobility or communication impairments, these assessments may lead to lower rankings, potentially disqualifying them before reaching human reviewers. 5
This raises concerns about the fairness of AI in recruitment processes, particularly regarding accessibility for disabled candidates.
A TikTok user showed how GenAI may depict autistic individuals as depressed and melancholic white men with glasses and mostly ginger hair:
Eliminating selected accents in call centers
Bay Area startup Sanas developed an AI-based accent translation system to make call center workers from around the world sound more familiar to American customers. The tool transforms the speaker’s accent into a “neutral” American accent in real time. As SFGATE reports, Sanas president Marty Sarim says accents are a problem because “they cause bias and they cause misunderstandings.”
Racial biases cannot be eliminated by making everyone sound white and American. To the contrary, it will exacerbate these biases since non-American call center workers who don’t use this technology will face even worse discrimination if a white American accent becomes the norm.
What is AI bias?
AI bias is an anomaly in the output of machine learning algorithms, due to the prejudiced assumptions made during the algorithm development process or prejudices in the training data.
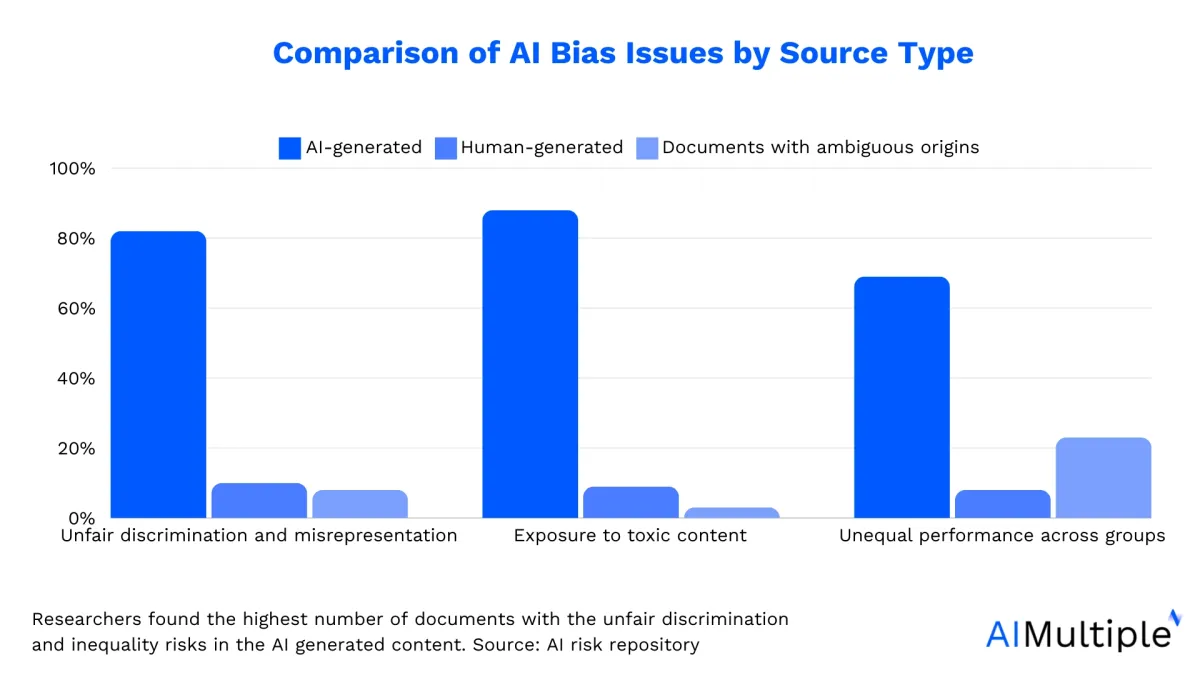
What are the types of AI bias?
AI systems contain biases due to two reasons:
- Cognitive biases: These are unconscious errors in thinking that affects individuals’ judgements and decisions. These biases arise from the brain’s attempt to simplify processing information about the world. More than 180 human biases have been defined and classified by psychologists. Cognitive biases could seep into machine learning algorithms via either
- designers unknowingly introducing them to the model
- a training data set which includes those biases.
- Algorithmic Bias: Machine learning software or other AI technologies reinforce existing biases present in the training data or through the algorithm’s design. This can happen due to explicit biases in the programming or pre existing beliefs held by the developers. For example, a model that overly emphasizes income or education can reinforce harmful stereotypes and discrimination against marginalized groups.
- Lack of complete data: If data is not complete, it may not be representative and therefore it may include bias. For example, most psychology research studies include results from undergraduate students which are a specific group and do not represent the whole population.
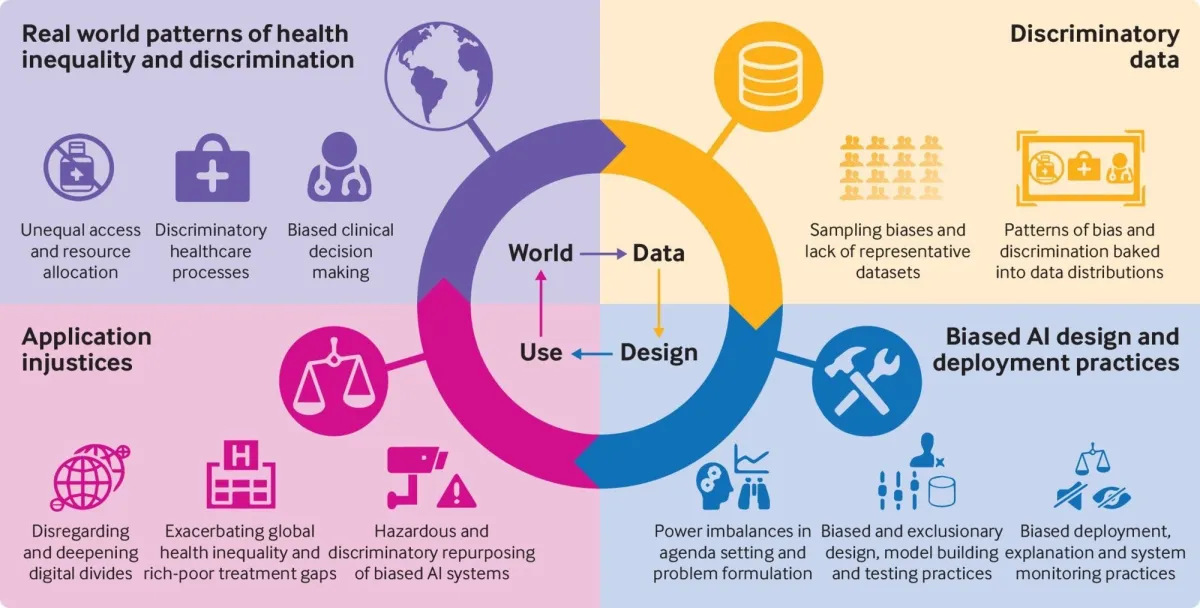
Based on the training data, AI models can suffer from several biases such as:
- Historical bias: Occurs when AI models are trained on historical data that reflects past prejudices. This can lead to the AI perpetuating outdated biases, such as favoring male candidates in hiring because most past hires were men.
- Sample bias: Arises when training data doesn’t represent the real-world population. For example, AI trained on data mostly from white men may perform poorly on non-white, non-male users.
- Label bias: Happens when data labeling is inconsistent or biased. If labeled images only show lions facing forward, the AI may struggle to recognize lions from other angles.
- Aggregation bias: Occurs when data is aggregated in a way that hides important differences. For example, combining data from athletes and office workers could lead to misleading conclusions about salary trends.
- Confirmation bias: Involves favoring information that confirms existing beliefs. Even with accurate AI predictions, human reviewers may ignore results that don’t align with their expectations.
- Evaluation bias: Happens when models are tested on unrepresentative data, leading to overconfidence in the model’s accuracy. Testing only on local data might result in poor performance on a national scale.
Is Generative AI biased?
Since 2022, the launch of ChatGPT, the interest in and applications of in generative AI tools have been increasing. Gartner forecasts that by 2025, generative AI will produce 10% of all generated data.7
However, the latest research shows that the data created by GenAI can be biased just like other AI models. For example, A 2023 analysis of over 5,000 images created with the generative AI tool that it amplifies both gender and racial stereotypes. 8
Another study compares three GenAI tools for their age, gender and emotion representations (See Figure 2), showing how all models reproduce the social biases and inequalities.9
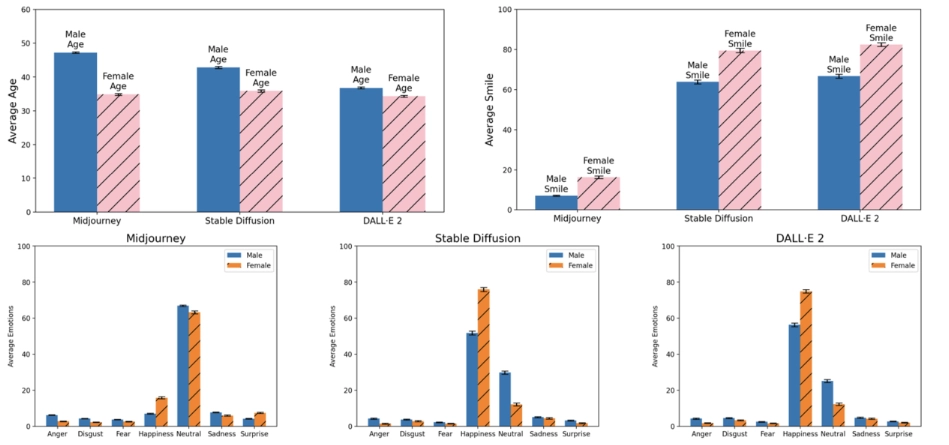
Such biases in AI can have real-world impacts, such as increasing the risk of harm to over-targeted populations when integrated into police department software, leading to potential physical injury or unlawful imprisonment.
Will AI ever be completely unbiased?
Technically, yes. An AI system can be as good as the quality of its input data. If you can clean your training dataset from conscious and unconscious assumptions on race, gender, or other ideological concepts, you are able to build an AI system that makes unbiased data-driven decisions.
In reality, AI is unlikely to ever be completely unbiased, as it relies on data created by humans, who are inherently biased. The identification of new biases is an ongoing process, constantly increasing the number of biases that need to be addressed. Since humans are responsible for creating both the biased data and the algorithms used to identify and remove biases, achieving complete objectivity in AI systems is a challenging goal.
What we can do about AI bias is to minimize it by testing data and algorithms and developing AI systems with responsible AI principles in mind.
How to fix biases in AI and machine learning algorithms?
Firstly, if your data set is complete, you should acknowledge that AI biases can only happen due to the prejudices of humankind and you should focus on removing those prejudices from the data set. However, it is not as easy as it sounds.
A naive approach is removing protected classes (such as sex or race) from data and deleting the labels that make the algorithm biased. Yet, this approach may not work because removed labels may affect the understanding of the model and your results’ accuracy may get worse.
So there are no quick fixes to removing all biases but there are high level recommendations from consultants like McKinsey highlighting the best practices of AI bias minimization:
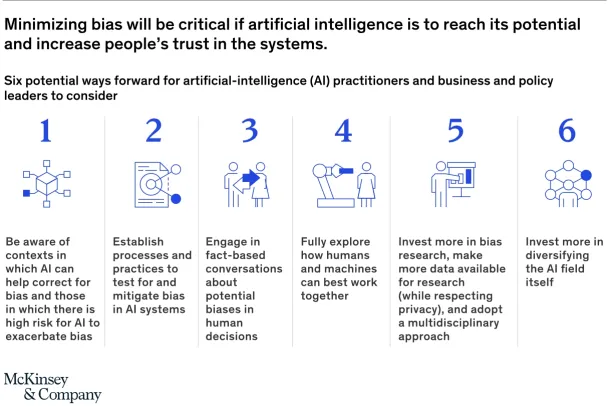
Steps to fixing bias in AI systems:
- Fathom the algorithm and data to assess where the risk of unfairness is high. For instance:
- Examine the training dataset for whether it is representative and large enough to prevent common biases such as sampling bias.
- Conduct subpopulation analysis that involves calculating model metrics for specific groups in the dataset. This can help determine if the model performance is identical across subpopulations.
- Monitor the model over time against biases. The outcome of ML algorithms can change as they learn or as training data changes.
- Establish a debiasing strategy within your overall AI strategy that contains a portfolio of technical, operational and organizational actions:
- Technical strategy involves tools that can help you identify potential sources of bias and reveal the traits in the data that affects the accuracy of the model
- Operational strategies include improving data collection processes using internal “red teams” and third party auditors. You can find more practices from Google AI’s research on fairness
- Organizational strategy includes establishing a workplace where metrics and processes are transparently presented
- Improve human-driven processes as you identify biases in training data. Model building and evaluation can highlight biases that have gone noticed for a long time. In the process of building AI models, companies can identify these biases and use this knowledge to understand the reasons for bias. Through training, process design and cultural changes, companies can improve the actual process to reduce bias.
- Decide on use cases where automated decision making should be preferred and when humans should be involved.
- Follow a multidisciplinary approach. Research and development are key to minimizing the bias in data sets and algorithms. Eliminating bias is a multidisciplinary strategy that consists of ethicists, social scientists, and experts who best understand the nuances of each application area in the process. Therefore, companies should seek to include such experts in their AI projects.
- Diversify your organisation. Diversity in the AI community eases the identification of biases. People that first notice bias issues are mostly users who are from that specific minority community. Therefore, maintaining a diverse AI team can help you mitigate unwanted AI biases.
A data-centric approach to AI development can also help minimize bias in AI systems.
Tools to reduce bias
To prevent AI bias, companies can benefit from these technologies and tools:
AI governance tools
AI governance tools ensure that AI technologies adhere to ethical and legal standards, preventing biased outputs and promoting transparency. These tools help in addressing bias throughout the AI lifecycle by monitoring ai tools for algorithmic bias and other existing biases.
Responsible AI platforms
A responsible AI platform can offer integrated solutions for ai design, prioritizing fairness and accountability. They include features like bias detectionand ethical risk assessments, preventing stereotyping bias and ensuring AI systems do not reinforce harmful stereotypes or discrimination against marginalized groups or certain genders.
MLOps That Deliver Responsible AI Practices
MLOps tools (Machine Learning Operations) platforms streamline machine learning processes by integrating responsible AI practices, reducing potential bias in models. These platforms ensure continuous monitoring and transparency, safeguarding against explicit biases in machine learning software.
LLMOps That Deliver Responsible AI Practices
LLMOps tools (Large Language Model Operations) platforms focus on managing generative AI models, ensuring they do not perpetuate confirmation bias or out group homogeneity bias. These platforms include tools for bias mitigation, maintaining ethical oversight in the deployment of large language models.
Data Governance Tools
Data governance tools manage the data used to train AI models, ensuring representative data sets free from institutional biases. They enforce standards and monitor data collected, preventing flawed data or incomplete data from introducing measurement bias into AI systems, which can lead to biased results and bias in artificial intelligence.
Extra resources
Krita Sharma’s Ted Talk
Krita Sharma, who is an artificial intelligence technologist and business executive, is explaining how the lack of diversity in tech is creeping into AI and is providing three ways to make more ethical algorithms:
Barak Turovsky at 2020 Shelly Palmer Innovation Series Summit
Barak Turovsky, who is the product director at Google AI, is explaining how Google Translate is dealing with AI bias:
Hope this clarifies some of the major points regarding biases in AI. For more on how AI is changing the world, you can check out articles on AI, AI technologies and AI applications in marketing, sales, customer service, IT, data or analytics.
Also, feel free to follow our Linkedin page where we share how AI is impacting businesses and individuals or our Twitter account.
If you are looking for AI vendors, you can benefit from our data-driven lists of:
If you have a business problem that is not addressed here:
External Links
- 1. AI was asked for images of Black African docs treating white kids. How'd it go? : Goats and Soda : NPR. NPR
- 2. Reproducing inequality: How AI image generators show biases against women in STEM | United Nations Development Programme.
- 3. The viral AI avatar app Lensa undressed me—without my consent | MIT Technology Review. MIT Technology Review
- 4. [2403.02726] Bias in Generative AI.
- 5. I Tried HireVue's AI-Powered Job Interview Platform - Business Insider. Business Insider
- 6. The MIT AI Risk Repository.
- 7. “Top Strategic Technology Trends for 2022” (PDF). Gartner. 2021. Retrieved November 1, 2022.
- 8. Generative AI Takes Stereotypes and Bias From Bad to Worse. Bloomberg
- 9. [2403.02726] Bias in Generative AI.
Comments
Your email address will not be published. All fields are required.