AI systems achieved remarkable milestones (e.g., exceeding human performance in image and speech recognition); however, AI progress is slowing down as scaling yields fewer benefits.1
Additionally, AI and ML models degrade over time unless they are regularly updated or retrained.2 This makes it critical to utilize all levers to improve AI models continually.
Explore key strategies, including data feeding, data and algorithm improvement, and AI scaling laws that will ensure your AI models stay relevant and practical.
Top 10 ways to improve your AI model
We explained methods to enhance your AI model in 4 different categories:
Method | Strategies | Description | Challenges |
---|---|---|---|
Feed More Data | 1. Data Collection (private, automated, crowdsourcing) 2. Synthetic Data | Adding more fresh, varied data to improve accuracy and generalization | Ensuring data quality, ethical concerns, and data accessibility |
Improve the Data | 1. Enrich Data 2. Improve Data Quality 3. Data Augmentation | Enhancing data through better labeling, augmentation, and diversity | Balancing quality with quantity, dealing with bias and noise |
Improve the Algorithm | 1. Improve Architecture 2. Feature Re-engineering | Upgrading model architecture and features for better efficiency | Complexity in re-engineering and resource demands |
Scaling Laws of AI | 1. Scaling Model Size 2. Scaling Data 3. Scaling Compute | Increasing model size, data, or compute to boost performance | Diminishing returns, compute bottlenecks, and high costs |
Feed more data
Adding new and fresh data is one of the most common and effective methods of improving the accuracy of your machine-learning model. Research has shown a positive correlation between dataset size and AI model accuracy.3
Therefore, expanding the dataset that is used for model retraining can be an effective way to improve AI/ML models. Make sure that the data changes according to the environment in which it is deployed. It is also essential to adhere to proper data collection quality assurance practices.
1. Data Collection
Data collection/harvesting can be used to expand your dataset and feed more data into the AI/ML model. In this process, fresh data is collected to re-train the model. This data can be harvested through the following methods:
- Private collection
- Automated data collection
- Custom crowdsourcing
To successfully collect data for AI, businesses need to look out for:
- Ethical and legal considerations in data collection must be respected to avoid any ethical issues.
- Bias in training data can lead to unwanted AI outcomes.
- Preprocessing raw data is essential to address quality issues and ensure data integrity for AI/ML training.
- Not all data is easily accessible due to restrictions related to sensitivity and privacy regulations.
Learn more about data collection methods.
It is also advised to work with an AI data service to obtain relevant datasets without the hassle of gathering data and to avoid any ethical and legal problems. Check out data collection services & companies and data crowdsourcing platforms to find the right data collection service for your AI project.
2. Synthetic data
Synthetic data refers to data that is artificially generated rather than derived from real-world events. Using algorithms, this data can be created to simulate various scenarios or conditions. It serves a range of purposes, such as testing new AI/ML models, validating model performance, and retraining existing models.
Ensuring that training data is representative and covers a wide range of real-world scenarios can mitigate risks such as diminishing returns caused by overfitting or poor generalization.
This can be especially challenging when real data is scarce or difficult to collect, which is where synthetic data becomes a powerful tool.
Synthetic data can be generated using algorithms to create realistic data for training and model validation, addressing gaps in datasets where real-world data is unavailable or too costly to acquire.
Learn more about how synthetic data can be generated.
Real-life example: More data for chatbots
A chatbot for IT support struggled with understanding and classifying user questions accurately. To improve its performance, 500 IT support queries were rewritten into multiple variations across seven languages.
This additional data helped the chatbot recognize different question formats, enhancing its ability to respond more effectively over time.4
Improve the data
Improving the existing data can also result in an improved AI/ML model.
Now that AI solutions are tackling more complex problems, better and more diverse data is required to develop them. For instance, research5 about a deep-learning model that helps object detection systems understand the interactions between two objects, concludes that the model is susceptible6 to dataset bias and requires a diverse dataset to produce results.
Improvements can be achieved through:
3. Enriching the data
Expanding the dataset is one way to improve AI. Another important way of enhancing AI/ML models is by enriching the data. This simply means that the new data that is collected to expand the dataset must be processed before being fed into the model.
This can also mean improving the annotation of the existing dataset. Since new and improved labeling techniques have been developed, they can be implemented on the existing or newly gathered dataset to improve model accuracy.
4. Improving data quality
Improving data quality is essential for advancing AI systems and enhancing the performance of AI models. While AI advancements often emphasize better algorithms and more computing power, high-quality training data remains crucial for optimal performance.
Adopting a data-centric approach helps accelerate AI progress by ensuring that the data used in training is not only abundant but also of high quality.
The collection and curation of high-quality data enable developers to build more efficient and effective AI models, which can then be leveraged to solve complex tasks across various industries. By focusing on data quality, businesses can make more accurate predictions, reduce bias, and enhance the capabilities of AI systems.
The quality of data can be significantly improved during the data collection phase. This process includes ensuring that data is representative of the real-world scenarios the model will encounter to eliminate bias, reduce noise, and make sure it is diverse enough to capture all relevant variables.
Additionally, maintaining consistency in data labeling and addressing gaps in the dataset can help reduce errors in the model’s learning process.
5. Leveraging data augmentation
Some people might confuse augmented data with synthetic data; however, both terms have some differences. Augmented data refers to the addition of information to an existing dataset, while synthetic data is generated artificially to stand in for real data. Augmented data is often used to improve the accuracy of predictions or models, while synthetic data is commonly used for testing and validation.
Check out to learn more about different techniques of data augmentation.
Real-life example: Speech recognition data improvement
Challenge: The speech recognition system for car infotainment struggled to understand diverse voice commands.
Solution: Thousands of voice recordings from different regions were collected, transcribed, and analyzed to improve recognition accuracy. This improvement in the voice dataset helped train the system to respond better to various commands and pronunciations.7
Improve the algorithm
Sometimes, the algorithm that was initially created for the model needs to be improved. This can be due to different reasons, including a change in the population on which the model is deployed.
Suppose a deployed AI/ML algorithm that evaluates the patient’s health risk and does not include the income level parameter is suddenly exposed to data of patients with lower income levels. In that case, it is unlikely to produce fair evaluations.
Therefore, upgrading the algorithm and adding new parameters to it can be an effective way to improve model performance. The algorithm can be improved in the following ways:
6. Improve the architecture
There are a few things that can be done in order to improve the architecture of an algorithm. One way is to take advantage of modern hardware features, such as SIMD instructions or GPUs.8
Additionally, data structures and algorithms can be improved through the use of cache-friendly data layouts and efficient algorithms. Finally, algorithm developers can exploit recent advances in machine learning and optimization techniques.
The Transformer is a deep learning architecture that changed the natural language processing (NLP) and other fields by enabling more efficient and effective modeling of sequence data. Introduced in the paper “Attention Is All You Need”9 , it relies heavily on a mechanism called self-attention, replacing recurrent and convolutional operations used in earlier models like RNNs and CNNs.
A Transformer consists of an Encoder and a Decoder, each built from multiple stacked layers:
The Encoder transforms input sequences into context-aware representations using multi-head self-attention to capture token relationships, feedforward networks for processing, and residual connections with layer normalization for stability.
The Decoder generates output sequences token by token, by incorporating masked multi-head self-attention to prevent future token access, cross-attention to integrate Encoder outputs, and similar feedforward and normalization mechanisms for efficient learning.
Real-life example: GPT4 with MoE
The Mixture of Experts (MoE) is a scalable architecture technique used to improve the performance and efficiency of large language models like GPT-4. It introduces a specialized way of structuring and activating parts of the model, allowing it to dynamically allocate computational resources based on the input, rather than using the entire model for every task.
- Sparse activation: In traditional dense models, all parameters contribute to every prediction. With MoE, only a few experts are active for any given input, therefore reducing computational costs. For example, if GPT-4 has 1,000 experts, the router may activate only 10 for a particular input.
- Dynamic routing: A learned routing mechanism decides which experts to activate based on the input. This allows the model to adaptively use its capacity for different types of tasks or contexts.
- Increased capacity with efficiency: MoE allows GPT-4 to scale up to trillions of parameters without proportionally increasing computational demands, as only a small fraction of the model is active during any single computation.
7. Feature re-engineering
Feature re-engineering of an algorithm is the process of improving the algorithm’s features in order to make it more efficient and effective. This can be done by modifying the algorithm’s structure or by tweaking its parameters.
Real-life example: Deepmind
Google DeepMind made significant improvements to its AI models by optimizing their architecture and re-engineering various components for better performance. For example, the Gemini model was built with a multimodal architecture, enabling it to handle tasks across text, audio, and images more effectively.
Additionally, PaLM 2 was enhanced by using a compute-optimal scaling approach and dataset improvements to enhance reasoning tasks. These architectural upgrades allowed for greater accuracy and adaptability.10
Scaling laws of AI
Scaling laws in AI machine learning applications describe how the performance of models improves as certain factors (such as model size, data, or compute) increase.
In deep learning, a key scaling law links the computational resources used for training (e.g., the number of compute hours or FLOPs—floating point operations) to the model’s performance.
8. Scaling model size
Increasing the number of parameters in a model means making it larger, typically by adding more layers or making existing layers more complex. Larger models can:
- Capture more complex patterns: With more parameters, the model can represent more intricate relationships in the data.
- Handle larger datasets: Bigger models have greater capacity to process and learn from large-scale data.
However, the relationship between model size and performance may exhibit diminishing returns. A 10x increase in model size does not necessarily lead to a 10x improvement in performance.
Larger models also require exponentially more compute and memory resources which can make them costly and harder to train. Beyond a certain point, increasing model size might produce negligible gains, particularly if the dataset or compute resources are insufficient.
9. Scaling data
The availability and size of the dataset used to train a model significantly affect its performance:
- Larger datasets improve generalization: With more diverse and comprehensive data, the model learns a wider range of patterns and is less likely to overfit.
- Better understanding of rare events: Large datasets help the model learn rare and diverse patterns which would make it better at handling unusual cases.
However, scaling data also has limits:
- Leveling-off gains: After a certain point, adding more data provides diminishing returns in performance because the model has already learned most of the useful patterns.
- Quality over quantity: Poor-quality or noisy data may not improve performance, even in large volumes.
- Compute bottleneck: Larger datasets demand more compute power and training time, which can be prohibitive.
10. Scaling compute
Scaling compute involves increasing the computational power available during training or inference, typically through:
- More powerful hardware: GPUs, TPUs, or specialized AI chips.
- Distributed systems: Training across multiple machines in parallel to handle large workloads.
- Longer training durations: Allowing the model to optimize its weights over more iterations.
The relationship between compute and model performance is foundational:
- More compute enables larger models: Scaling compute allows for training models with more parameters.
- Extended training: With sufficient compute, models can train on larger datasets for longer periods, which would lead to better optimization.
However, scaling compute also has challenges:
- Diminishing returns: While performance improves with more compute, the rate of improvement slows as the resources increase.
- Cost and energy demands: Training advanced models like GPT-4 requires extensive financial and environmental resources.
Despite these challenges, scaling compute has been instrumental in driving AI machine learning improvements.
In the inference stage, the performance of an AI model, particularly for tasks requiring maths or multi-step reasoning, can improve by allocating more compute time. This is often achieved through strategies like increased computation per query or iterative refinement. Here’s how it works:
What happens during inference?
Inference is the stage where a pre-trained model is used to generate predictions or perform tasks based on new inputs. Unlike training, inference doesn’t update the model’s weights but relies on its learned capabilities to solve specific problems.
Why more compute time helps?
When performing tasks like mathematical calculations or multi-step reasoning, the model benefits from more time and resources per query because:
- Iterative refinement: For tasks requiring multiple logical steps, the model can break the problem into smaller parts, solve each part, and iteratively refine its solution. Allocating more compute allows the model to process these steps more thoroughly.
- Increased precision: In mathematical tasks, longer inference time allows for deeper exploration of patterns or trial-and-error mechanisms to approximate correct solutions.
- Better contextual understanding: In tasks like multi-step reasoning, a model with more compute time can evaluate the context repeatedly, to ensure that intermediate steps align with the broader problem.
Real-life example:
OpenAI’s GPT models are capable of handling multi-step reasoning tasks, and their performance improves when they are allocated more compute time for processing.
OpenAI’s GPT models perform better on multi-step reasoning tasks and longer prompts when given more compute time during inference. This enhances their ability to:
- Analyze and understand detailed context.
- Perform step-by-step reasoning.
- Refine and verify intermediate solutions for greater accuracy.
Recommendations on how to approach AI/ML model improvement
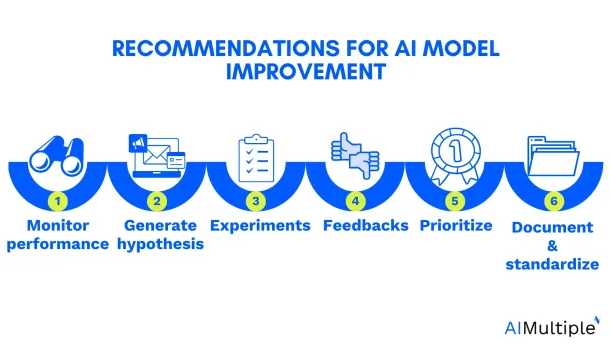
Improving an AI/ML model requires a strategic approach to identify areas to implement effective solutions. By combining performance monitoring with hypothesis-driven decision-making, AI/ML models can be refined and optimized for better outcomes:
Monitor performance
You can only improve something by knowing its areas for improvement. This can be done by monitoring the features of the AI/ML model. However, if all the model features can not be monitored, only a selected number of key features can be observed to study variations in their output that can impact the model’s performance.
Hypothesis generation
Prior to selecting the right method, we recommend performing hypothesis generation. This is a pre-decisional process that structures the decision process and narrows down the options.
This process involves gaining domain knowledge, studying the problem the AI/ML model is facing, and narrowing down readily available options that can tackle the identified issues.
Iterative improvement and experimentation
AI/ML model improvement is an ongoing process. After forming hypotheses and selecting potential solutions, experimentation and iteration are key to refining the model.
A/B Testing: Test different models or changes on subsets of data to compare results. This helps identify which improvements are most effective.
Model retraining: Regularly retrain the model with new data, feature updates, or algorithm adjustments to ensure it stays relevant and adapts to changing conditions.
Automated monitoring and feedback loops: Use automated systems to provide continuous AI feedback, enabling quick adjustments and rapid iteration on improvements.
Incorporate feedback from stakeholders
An often overlooked part of the model improvement process is gathering input from end-users or stakeholders. AI feedback collected from business teams, domain experts, or end users offers valuable context to refine predictions and address real-world blind spots.
Integrating this feedback loop helps ensure the model adapts continuously and remains aligned with operational needs..
This feedback loop ensures the model remains aligned with real-world needs and expectations.
Prioritize the most impactful changes
Not all improvements will have the same level of impact. It is essential to prioritize changes that directly address the most critical performance issues.
For example, improving data quality or addressing a significant bias in the model might have more substantial effects than minor adjustments to the algorithm’s hyperparameters.
Document and standardize the improvement process
For continuous improvements, document the methods, experiments, and results.
Standardizing this process allows for future enhancements to follow a proven, structured approach, ensuring that improvements can be measured, compared, and tracked over time.
Current state of AI improvement
The computational power available for training AI models has exponentially increased. The vast availability of data enables AI systems to learn from extensive examples, therefore enhancing their accuracy and performance.
Contrary to the belief that technological advancements are unpredictable, AI progress follows a foreseeable trajectory driven by the systematic enhancement of compute, data, and algorithms.
Despite the massive advancements in AI machine learning applications, recent developments indicate that progress has started to lose momentum.
One example is that OpenAI’s next major language model, code-named “Orion,” reportedly offers smaller performance gains over GPT-4, with limited improvements in areas like programming. A key factor behind this stagnation is the limited availability of high-quality training data, which is a challenge impacting the entire AI industry.
In response, OpenAI has formed a foundations team to address these limitations by exploring strategies such as using synthetic AI-generated data and enhancing models through post-training improvements.11
FAQs
How has AI technology improved over the years?
The evolution of artificial intelligence has led to remarkable progress in natural language processing (NLP). Today’s AI systems can understand, interpret, and generate human language with unprecedented accuracy. This significant leap is evident in sophisticated chatbots, language translation services, and voice-activated assistants.
How can I improve the accuracy of my AI model?
To enhance your AI model’s accuracy, consider collecting more high-quality and diverse training data. Additionally, fine-tune your model’s hyperparameters, experiment with different algorithms, and apply techniques like cross-validation to optimize performance.
What are effective methods for preventing overfitting in AI models?
Prevent AI overfitting by using regularization techniques, implementing dropout layers in neural networks, and employing early stopping during training. Increasing your dataset size and ensuring data diversity can also help your model generalize better to new inputs.
Further reading
- 4 Steps and Best Practices to Effectively Train AI
- AI chips: A guide to cost-efficient AI training & inference in 2022
External Links
- 1. OpenAI and others seek new path to smarter AI as current methods hit limitations | Reuters. Reuters
- 2. Temporal quality degradation in AI models | Scientific Reports. Nature Publishing Group UK
- 3. Professional Services - Telstra Enterprise.
- 4. Training Data for Chatbots - Case Study by clickworker.
- 5. [2111.09297] Learning to Compose Visual Relations.
- 6. More complex deep learning models require more complex data | by Anyverse | Anyverse™ | Medium. Anyverse™
- 7. Training data for machine learning of speech recognition systems.
- 8. Single instruction, multiple data - Wikipedia. Contributors to Wikimedia projects
- 9. https://arxiv.org/pdf/1706.03762
- 10. 2023: A Year of Groundbreaking Advances in AI and Computing - Google DeepMind.
- 11. OpenAI's new "Orion" model reportedly shows small gains over GPT-4. THE-DECODER.com
Comments
Your email address will not be published. All fields are required.