Responsible AI: 4 Principles & Best Practices in 2024
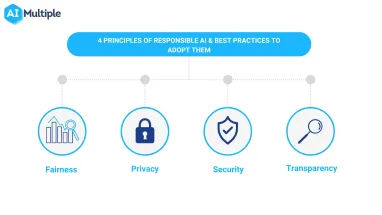
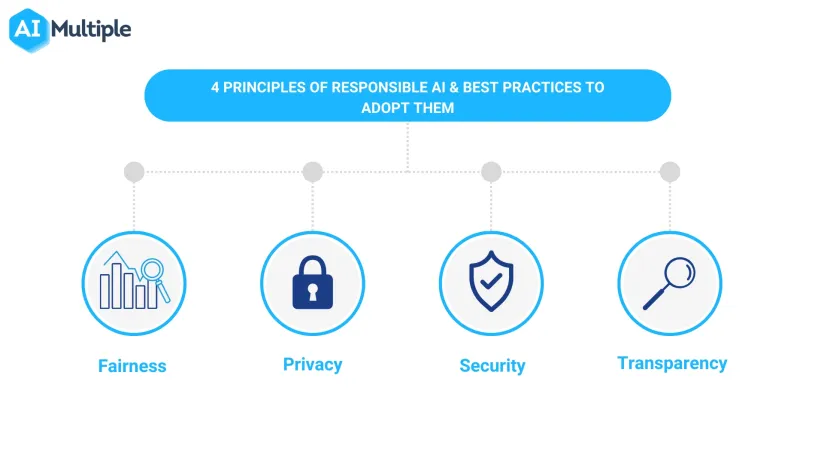
The use of artificial intelligence (AI) and machine learning (ML) is transforming industries, helping businesses solve real-world problems, and improving the daily lives of people around the world. It is expected that its usage will become more widespread in the near future: 90% of commercial apps will use AI by 2025, and the AI industry could be worth more than $15 trillion by 2030.
However, the use cases and possibilities of AI bring with them great responsibilities, and many organizations are struggling to address the risks associated with AI adoption. According to Accenture, about 65% of risk leaders believe they are not fully capable of assessing the risks of AI. 1
Developing and scaling AI applications with responsibility, trustworthiness, and ethical practices in mind is essential to build AI that works for everyone. In this research, we’ll explore four principles for responsible AI (RAI) design and recommend best practices to achieve them.
1. Fairness
AI tools are increasingly being used in various decision-making processes such as hiring, lending, and medical diagnosis. Biases introduced in these decision-making systems can have far-reaching effects on the public and contribute to discrimination against different groups of people.
Here are three examples of AI bias in real-world applications:
- In 2019, there were multiple claims (including from co-founder Steve Wozniak) that Apple’s credit card algorithm discriminates against women, offering different credit limits based on gender.
According to a recent report by Harvard Business School and Accenture, 27 million workers in the US are filtered out and unable to find a job due to automated and AI-based hiring systems. 2 These “hidden workers” include immigrants, refugees, and those with physical disabilities.
Researchers found 3 that some commercial facial recognition technologies, such as Amazon’s or Microsoft’s, had poor accuracy on dark-skinned women, but were more accurate on light-skinned men (Figure 1).
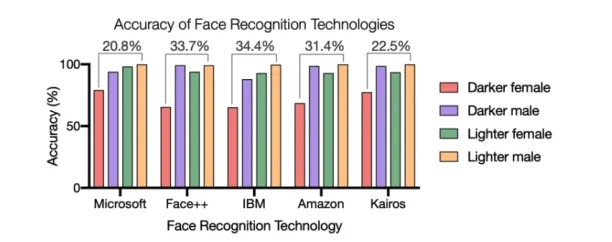
These biased decisions can result from project design or from datasets that reflect real-world biases. It is critical to eliminate these biases to create AI systems that are robust and inclusive to all.
Best practices to achieve fairness
- Examine the dataset for whether it is a fair representation of the population.
- Analyze the subpopulations of the dataset to determine if the model performs equally well across different groups.
- Design models with fairness in mind and consult with social scientists and other subject matter experts.
- Monitor the machine learning model continuously after deployment. Models drift over time, so biases can be introduced to the system after some time.
We have a comprehensive article on AI bias and how to fix it. Feel free to check. You can also read our article on AI ethics.
2. Privacy
AI systems often use large datasets, and these datasets can contain sensitive information about individuals. This makes AI solutions susceptible to data breaches and attacks from malicious parties that want to obtain sensitive information:
- According to the Identity Theft Resource Center, there were 1862 data breaches in 2021, which is 23% higher than the previous all-time high in 2017.
Data breaches cause financial loss as well as reputational damage to businesses and can put individuals whose sensitive information is revealed at risk.
Best practices to ensure privacy
- Assess and classify data according to its sensitivity and monitor sensitive data.
- Develop a data access and usage policy within the organization. Implement the principle of least privilege
3. Security
The security of an AI system is critical to prevent attackers from interfering with the system and changing its intended behavior. The increasing use of AI in particularly critical areas of society can introduce vulnerabilities that can have a significant impact on public safety. Consider the following examples:
- Researchers have shown that they can get a self-driving car to drive in the opposite lane by placing small stickers on the road.
These adversarial attacks can involve:
- Data poisoning by injecting misleading data into training datasets.
- Model poisoning by accessing and manipulating the models.
among others to cause the AI model to act in unintended ways. As AI technology evolves, attackers will find new methods and new ways to defend AI systems will be developed.
Best practices to achieve security
- Assess whether an adversary would have an incentive to attack the system and the potential consequences of such an attack.
- Create a red team within your organization that will act as an adversary to test the system for identification and mitigation of vulnerabilities.
- Follow new developments in AI attacks and AI security. It is an ongoing area of research, so it is important to keep up with developments.
4. Transparency
Transparency, interpretability, or explainability of AI systems is a must in some industries such as healthcare and insurance in which businesses must comply with industry standards or government regulations. However, being able to interpret why AI models come up with specific outputs is important for all businesses and users to be able to understand and trust AI systems.
A transparent AI system can help businesses:
- Explain and defend business-critical decisions,
- Make “what-if” scenarios,
- Ensure that the models work as intended,
- Ensure accountability in case of unintended results.
Best practices to ensure transparency
- Use a small set of inputs that is necessary for the desired performance of the model. This can make it easier to accurately pinpoint where the correlation or the causation between variables comes from.
- Give explainable AI methods priority over models that are hard to interpret (i.e., black box models).
- Discuss the required level of interpretability with domain experts and stakeholders.
Feel free to check our data-driven lists of AI consultants and data science consultants for more on AI consultants. You can also check TensorFlow’s Responsible AI Toolkit ecosystem, which can help businesses adopt responsible AI practices.
If you have other questions about responsible AI principles and how to adopt them in your AI initiatives, we can help:
External Links
- 1. Culp, Steve. (2021). “In a world of risk, pace comes from preparation.” Accenture. Revisited January 20, 2023.
- 2. Fuller,Joseph B.; Raman, Manjari; Sage-Gavin, Eva; Hines, Kristen (2021). “HIDDEN WORKERS: UNTAPPED TALENT.” HBR. Revisited January 20, 2023.
- 3. Buolamwini, J., & Gebru, T. (2018, January). “Gender shades: Intersectional accuracy disparities in commercial gender classification.” In Conference on fairness, accountability and transparency (pp. 77-91). PMLR.

Cem has been the principal analyst at AIMultiple since 2017. AIMultiple informs hundreds of thousands of businesses (as per similarWeb) including 60% of Fortune 500 every month.
Cem's work has been cited by leading global publications including Business Insider, Forbes, Washington Post, global firms like Deloitte, HPE, NGOs like World Economic Forum and supranational organizations like European Commission. You can see more reputable companies and media that referenced AIMultiple.
Throughout his career, Cem served as a tech consultant, tech buyer and tech entrepreneur. He advised businesses on their enterprise software, automation, cloud, AI / ML and other technology related decisions at McKinsey & Company and Altman Solon for more than a decade. He also published a McKinsey report on digitalization.
He led technology strategy and procurement of a telco while reporting to the CEO. He has also led commercial growth of deep tech company Hypatos that reached a 7 digit annual recurring revenue and a 9 digit valuation from 0 within 2 years. Cem's work in Hypatos was covered by leading technology publications like TechCrunch and Business Insider.
Cem regularly speaks at international technology conferences. He graduated from Bogazici University as a computer engineer and holds an MBA from Columbia Business School.
To stay up-to-date on B2B tech & accelerate your enterprise:
Follow on
Comments
Your email address will not be published. All fields are required.