89% of all companies across different sectors are switching to digital technologies and generative AI in fashion industry is not an exception. McKinsey reports that in 2021, fashion brands and companies invested approximately 1.7% of their income in emerging technologies. Moreover, they estimate the figure will rise between 3.0% and 3.5% by 2030.1
Blockchain technology, non-fungible tokens (NFTs), and AI technology are digital technologies that are implemented in the fashion industry. On the other hand, generative AI is relatively new; yet it started affecting many elements of the fashion industry.
Here, we’ll explore the use cases and real-world examples of how generative AI is being leveraged to innovate in the fashion industry.
Generative AI tools for image & design generation
Before diving into specific applications of generative AI in the fashion industry, it’s important to understand how the technology creates original images and content that form the foundation of a design.
Using generative algorithms, AI can create unique, interesting images that merge computer-generated styling with human creativity. The artwork created by generative AI in this way offers an entirely new approach to creating visual art. It can tap into generative elements and generate infinite variations of the same image.
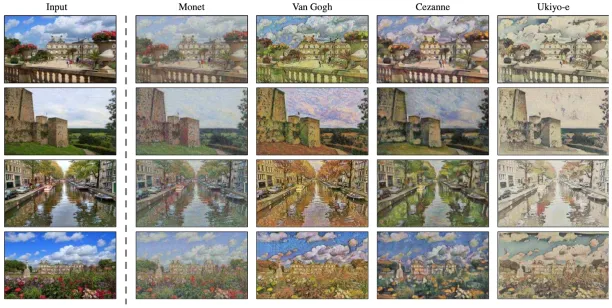
Figure 1. The cycleGAN algorithm is able to generate designs in the style of different artists and artistic genres, such as Monet, van Gogh, Cezanne and Ukiyo-e. (Source: ICCV 2017.)2
ComfyUI is a free, open-source, node-based tool built on diffusion models like Stable Diffusion, enabling users to design and iterate generative flows. In the fashion world, it’s increasingly leveraged to automate creative tasks such as virtual clothing try‑on, outfit swapping, and fashion‐idea generation:
Projects like FLUX AI utilize multi‑node ComfyUI setups to create polished, realistic outfit changes in seconds, matching industry standards.
Most AI-generated images are nearly impossible to differentiate from real ones. When participants in a study were unaware that generative AI technology had been used, they tended to perceive the images generated by GANs as more novel than the original images.3
Another famous generative AI tool, DALL-E, can create a wide range of images, including:
- Photorealistic images
- Abstract patterns
- Stylized illustrations.
It has been demonstrated to be capable of generating highly creative and novel images that go beyond what it was explicitly trained on. Some examples from its realistic and artistic generations:
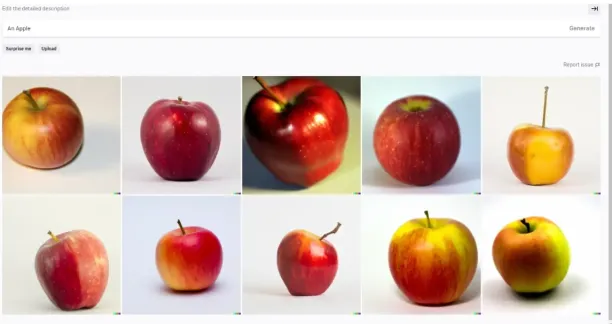
Figure 2. Entering “An Apple” will get a series of photorealistic apple images.
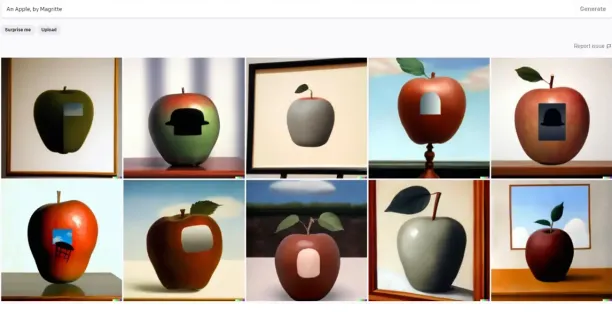
Figure 3. Adding modifier “by Magritte” dramatically changes the entire character of the prompt.
Here again, you can see how generative AI in fashion is capable of creating surprising and stylistic designs from a basic object.
5 use cases of generative AI in the fashion industry with example cases
1. Creative Designing for Fashion Designers
With its great ability to generate new images and content, generative AI can assist fashion designers in the creative design process by developing new ideas or helping to refine and optimize existing designs with the latest trends. This can be done through a variety of techniques, including:
- Generative design: Generative AI in fashion can create entirely new fashion designs based on specified constraints and parameters, such as the desired aesthetic, materials, and target market.
- Style transfer: Generative AI can be used to apply the style of one design to another, allowing designers to create variations on existing designs or combine elements from different sources.
Besides, you don’t need to be an exclusive fashion designer to create new designs. An ML engineer specializing in generative arts, Fathy Rashad, created his own generative cloth designer ClothingGAN by using StyleGan and GANSpace (see the figure below).4
Figure 4. Products generated by ClothingGAN.
2. Turning Sketches into Color Images
Generative AI benefits the fashion industry as it can also transform sketches into fully colored images. Generative AI allows designers and artists to experience their vision in real-time with minimal effort (see Figure 4). With this technology, they can save valuable time and resources while being able to experiment without difficulty.
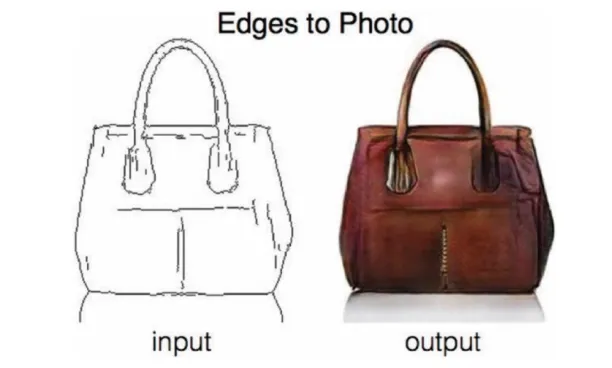
Figure 5. Image of a black-white sketch turned into photo-like color image by pix2pix.5
Additionally, generative AI can help limit human error, such as errors in color-matching and patterns. It can also enable fashion brands to become more creative, leveraging the ability to analyze numerous sketch-to-color combinations and generate multiple variations for review.
For example, Khroma is a tool that allows a trained algorithm to create genuine and personalized color palettes. Similarly, Colormind enables preparing creative color palettes based on preferred samples from movies, photographs, artworks, etc.
Implementing such tools can also help generative AI reduce the need for physical samples, saving time and resources.
3. Generating Representative Fashion Models
Using generative AI to create a diversity of fashion models can help fashion companies to serve a wide range of customers better and showcase their products in a more realistic and accurate way. A Cambridge University research shows that, when Dove’s advertising campaign featuring women of various skin tones and body types increased sales by 600% in two months.6
For being representative for all human body types, it can be used to create a diversity of fashion models in a virtual world in several ways:
- Virtual try-on: Generative AI can create virtual representations of fashion products that can be superimposed onto images of people, allowing customers to “try on” clothes virtually. These virtual models can be customized to represent a wide range of body types, colors, and sizes, allowing customers to see how the clothes would look on them specifically.
- 3D rendering: Generative AI can create 3D models of fashion products that can be rotated and viewed from different angles. These models can be customized to represent a wide range of body types, colors, and sizes, allowing designers to see how the clothes would look on different body models.
Japanese tech company DataGrid used GANS technology to create models that can change bodily. You can watch the video released by the company showing a multitude of generated models:
Lalaland is another tech startup that makes hyper realistic virtual fashion models driven by generative AI for use on e-commerce platforms. It works by creating model avatars, uploading the images of garments, styling the product, and then downloading output images.
4. Marketing & Trend Analysis for Fashion Brands
AI-powered generative models allow companies to speed up and improve their trend forecasting and marketing analytics capabilities. As a result, companies stay ahead of trends while meeting the customers’ future needs more effectively.
It can help trend analysis by:
- Bringing together a variety of techniques, such as machine learning and probabilistic programming. These techniques allow for powerful generative models that consider the customer desires in the fashion business.
- Generating deeply personalized options for specific consumer desires that go beyond what traditional analytics and customer demand algorithms can do.
It also improves marketing capabilities by:
- Using a combination of data analysis, natural language processing, and machine learning, brands can craft product ranges that feel uniquely tailored to the preferences and needs of their target audience.
- Designing emails, website pages, captions, and ads that are tailored to a specific person’s interests and preferences in order to engage them
- Plotting creative and authentic marketing and ad content that are likely to storm search results
5. Protecting Data Privacy of Consumers
The fashion industry can utilize generative AI to improve consumer data privacy. The generative AI algorithms allow fashion companies to generate new designs while keeping customer data private. With synthetic datasets that generative AI produces, companies are able to create unique patterns and automated data analytics while protecting customers’ details, such as:
- Contact information
- Banking information
- Purchase history
- Preferences
- More from third parties
It safeguards individuals’ financial security and provides organizations with valuable insights into their target market without invading people’s privacy. Thus, generative AI offers a secure way for fashion brands to revolutionize their business strategy.
Challenges of generative AI for the fashion industry
The biggest challenge of generative AI for creative sectors, such as the fashion industry, can be the ambiguities around the copyright of AI-generated work. Using generative AI in the fashion industry can lead to some problems such as:
- Disclaiming the uniqueness, originality, or copyright eligibility of the generated designs or other fashion materials
- Ownership problems about whether the fashion designer or the programmer of the AI deserves the authorship rights of the generated work
- Misuse of such tools for unethical marketing strategies
- Risk of diminishing human creativity in the fashion industry and leading to unemployment
For more on the challenges of generative AI, you can check our articles on the copyright and ethical concerns around generative AI.
FAQ
How is generative AI used in fashion design?
Generative AI in fashion helps designers create unique fashion pieces by analyzing trends, generating patterns, and suggesting new styles based on customer preferences.
Can generative AI in fashion improve the online shopping experience?
Yes, generative AI enhances online shopping by offering virtual try-ons, AI-driven size recommendations, and personalized styling advice. It allows customers to visualize how outfits will look on their body using augmented reality (AR) and helps brands reduce return rates.
Further reading
If you are interested in the generative AI creations, applications, and tools in general, you should also check these articles:
- AI Text Generation: Top 12 Use Cases & 2 Case Studies
- Top 70+ Generative AI Applications / Use Cases
- Generative AI in Retail: 7 Use Cases, Examples & Benefits
- Generative AI in Manufacturing Industry: 5 Use Cases & 5 Benefits
External Links
- 1. State of Fashion Technology Report 2022 | McKinsey. McKinsey & Company
- 2. “Unpaired image-to-image translation using cycle-consistent adversarial networks.” ICCV 2017, Zhu, J.Y., Park, T., Isola, P., Efros, A.A.
- 3. Artificial intelligence in the fashion industry | by Research Features | Medium. Medium
- 4. “How to Build an AI Fashion Designer | by Fathy Rashad.” Towards Data Science. Accessed 25 December 2022.
- 5. “Generative Models as Fashion Designers.” Artificial Intelligence for Fashion, 125–139. Luce, L. (2018). doi:10.1007/978-1-4842-3931-5_8 .
- 6. Generative Deep Learning in Fashion: The Pinnacles, Perils And Why We’ll Be Synthesising Supermodels - The Interline. The Interline
Comments
Your email address will not be published. All fields are required.