Audio Annotation in 2024: What is it & why is it important?
In 2020, the market capitalization of AI/ML was $22.59 billion and is expected to reach $125 billion, growing at about 40% per year. Supervised and human-in-the-loop ML models make successful predictions if they have high-quality labeled/annotated data, as both models learn reality via the categorization of humans.
A subset of data annotation – audio annotation – is a critical technique for building well-performing natural language processing (NLP) models that offer many benefits to organizations, such as analyzing text, speeding up customer responses, recognizing human emotions, etc. In this article, we take a deep dive into audio annotation to understand its importance for businesses.
What is audio annotation?
Audio annotation is a subset of data annotation that involves classifying components of audio that come from people, animals, the environment, instruments, and so on. For the annotation process, engineers use data formats such as MP3, FLAC, AAC, etc. Audio annotation, like all other types of annotation (such as image and text annotation), requires manual work and software specialized in the annotation process. In the case of audio annotation, data scientists specify the labels or “tags” by using software and pass the audio-specific information to the NLP model being trained.
Why is audio annotation important now?
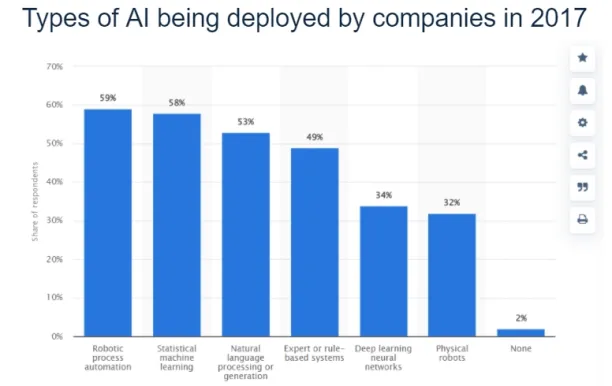
Audio annotation is crucial for the development of virtual assistants, chatbots, voice recognition security systems, etc. As the figure above shows, NLP is the third most common form of AI used by enterprises. In 2017, 53% of companies used some form of NLP. Consequently, it is a huge market in terms of value. The NLP market generated over $12 billion in revenue in 2020, and it is predicted that the market will grow at a compound annual growth rate (CAGR) of about 25% from 2021 to 2025, reaching over $43 billion in revenue. Consequently, audio labeling is an important task today.
In addition, customers are increasingly demanding digitized and fast customer service, as the following figure shows. Consequently, chatbots are becoming an integral part of customer service and the success of chatbots is directly related to the quality of audio annotation.
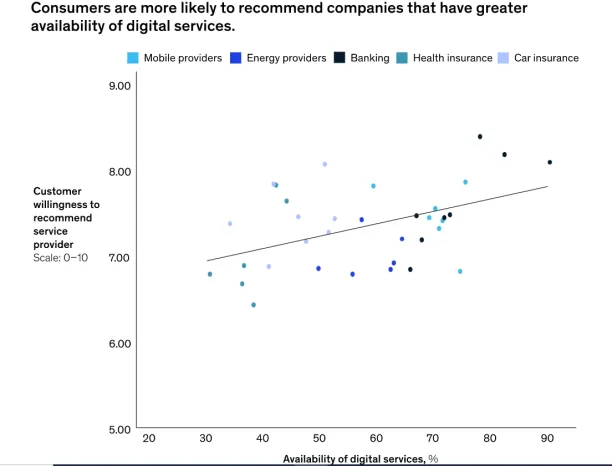
What are the types of audio annotation?
There are five main techniques of audio annotation:
- Speech to Text Transcription: Transcription of speech into text is an important part of the development of NLP models. This technique involves converting recorded speech into text, marking both words and sounds that the person pronounces. In this technique, it is also important to use correct punctuation.
- Audio Classification: Thanks to this technique, machines can distinguish voice and sound characteristics. This type of audio labeling is important for the development of virtual assistants, as the AI model recognizes who is performing the voice command.
- Natural Language Utterance: Natural language utterance is about annotating human speech to classify minute details such as semantics, dialects, context, intonation, and so on. Therefore, natural language utterance is an important part of training virtual assistants and chatbots.
- Speech Labeling: Data annotators separate the required sounds from a given recording and label them with keywords. This technique helps in the development of chatbots that handle a specific, repetitive task.
- Music Classification: Data annotators can mark genres or instruments in this kind of audio annotation. Music classification is very useful for organizing music libraries and improving user recommendations.
For a deeper understanding of audio data collection, feel free to download our data collection whitepaper:
How to annotate audio data?
Audio annotation software
Companies need software that specializes in audio annotation. It is possible to use third-party providers that offer open-source and closed-source audio annotation tools. Open-source audio annotation tools are free, and since the code is available to everyone, it can be customized to meet your organization’s needs. Closed-source tools, on the other hand, have a team available to help you set up and use the software for your business. However, there is a fee for this service.
An alternative to outsourcing could be to develop your own audio annotation software. However, this is a costly and slow process. The main advantage is that in-house tools offer greater data security. Nevertheless, developing your own software is only possible for a small proportion of firms that have sources and similar experience to accomplish such a challenging task.
In-housing vs outsourcing vs crowdsourcing
In-housing, outsourcing, and crowdsourcing are ways to perform the manual work of audio annotation. These methods come with varying costs, output quality, and data security. Therefore, it is an important strategic decision for organizations which method to use.
Of course, the optimal strategy depends on the organization’s capabilities, sources, and needs. However, the following table might help you choose the optimal strategy. For more information, see our article on data labeling outsourcing.
Outsource | In-house | Crowdsource | |
---|---|---|---|
Time required | Average | High | Low |
Price | Average | Expensive | Cheap |
Quality of labeling | High | High | Low |
Security | Average | High | Low |
Don’t forget to check our sortable/filterable list of data labeling/annotation/classification vendors list.
You might also want to read our articles on image and text annotation. If you are looking for a vendor for audio annotation, please contact us:

Cem has been the principal analyst at AIMultiple since 2017. AIMultiple informs hundreds of thousands of businesses (as per similarWeb) including 60% of Fortune 500 every month.
Cem's work has been cited by leading global publications including Business Insider, Forbes, Washington Post, global firms like Deloitte, HPE, NGOs like World Economic Forum and supranational organizations like European Commission. You can see more reputable companies and media that referenced AIMultiple.
Throughout his career, Cem served as a tech consultant, tech buyer and tech entrepreneur. He advised businesses on their enterprise software, automation, cloud, AI / ML and other technology related decisions at McKinsey & Company and Altman Solon for more than a decade. He also published a McKinsey report on digitalization.
He led technology strategy and procurement of a telco while reporting to the CEO. He has also led commercial growth of deep tech company Hypatos that reached a 7 digit annual recurring revenue and a 9 digit valuation from 0 within 2 years. Cem's work in Hypatos was covered by leading technology publications like TechCrunch and Business Insider.
Cem regularly speaks at international technology conferences. He graduated from Bogazici University as a computer engineer and holds an MBA from Columbia Business School.
To stay up-to-date on B2B tech & accelerate your enterprise:
Follow on
Comments
Your email address will not be published. All fields are required.