Around 1.72 trillion1 photos are taken every year. Many are used to train digital solutions, such as self-driving systems powered by image recognition and computer vision (CV) technologies. However, all of these images would be useless for such digital solutions without image classification – a process that can identify and categorize images based on their visual content.
Image classification is one of the cornerstones of computer vision solutions. However, many executives are still not clear about its applications and best practices, limiting the effectiveness of image classification solutions.
To remedy that, we have curated this article to explore what image classification is and how business leaders can leverage it to develop sophisticated image recognition solutions.
What is image classification?
Image classification is a technique that involves analyzing the content of an image to attach tags or labels to it. This distributes the images into different classes. The purpose of this process is to digitally explain the contents of the image to a machine. Image classification is combined with image localization to enable an object detection system to find objects in images.
Single-label vs. multi-label classification
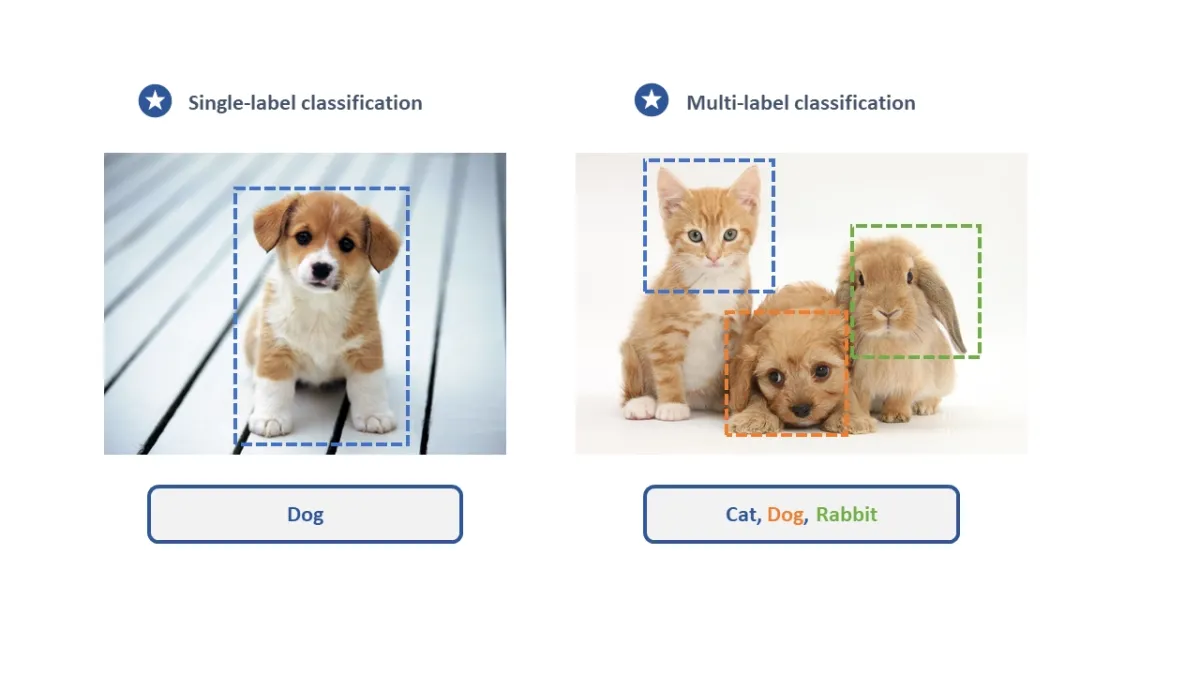
Single-label image classification is a traditional image classification problem where each image is associated with only one label or class. For instance, an image of a cat can be labeled as “cat” and nothing else. The task of the classifier is to predict the label for a given image.
On the other hand, multi-label image classification is an extension of the single-label image classification problem, where an image can have multiple labels or classes associated with it. For example, an image of a cat playing in a park can be labeled as “cat” and “park”. The task of the classifier, in this case, is to predict all the relevant labels or classes for a given image.
Multi-label image classification is a more complex problem than single-label image classification since it requires the classifier to identify multiple objects or features in an image and attach them with their corresponding labels.
Image classification can be done manually or with automated tools.
Manual image classification
Manual image classification is done by viewing each image and applying a label or category based on the data processor’s judgment of the contents of the image. Like many other manual tasks, manual image classification can also be tedious and prone to errors.
Automated image classification
Image classification can also be automated due to advancements in computer vision techniques and machine learning algorithms. This is achieved by feeding a large number of images attached with corresponding labels to the model.
Based on this training data, the model can learn to recognize and categorize objects within new images and automatically classify them. However, such tools are implemented through a supervised classification approach where a human classifier is in the loop. Automated data labeling tools usually include image classification features.
Business applications of image classification
1. Autonomous driving systems
Image classification is an essential part of autonomous driving systems. It is used to detect and classify objects in the surroundings of the vehicle, such as other cars, pedestrians, road signs, traffic lights, etc. The autonomous driving system uses this information to guide the vehicle.
2. Manufacturing
In the manufacturing sector, image classification is used to implement computer vision-enabled automated solutions in the following ways:
2.1. Quality control and defect detection
Image classification algorithms can be used to ensure quality control of finished products or parts on a manufacturing line. For example, in vehicle manufacturing, image classification algorithms can inspect car parts for cracks, chips, or other imperfections.
2.2. Sorting and classification
Image classification algorithms can also be used to sort and categorize products based on their characteristics. For instance, in the food and beverage sector, image classification models can scan and sort fruits and vegetables based on their size, shape, and other attributes.
3. Defense
In the defense sector, image classification is commonly used in areas like target identification, surveillance, and threat assessment. The models are made to automatically recognize and categorize objects in pictures and videos taken by satellites or unmanned aerial vehicles (UAVs).
4. Retail/e-commerce
Time and clarity are important to online shoppers, and if an online platform falls short in those areas, it leads to higher cart abandonment rates (See figure 1). Image classification can help in reducing this rate. Image classification can be used to automate product data categorization and tagging on online stores and e-commerce websites.
This can improve the customers’ shopping experience by allowing them to quickly search and find products based on relevant categories.
Figure 1. Reasons why people abandon their online shopping carts
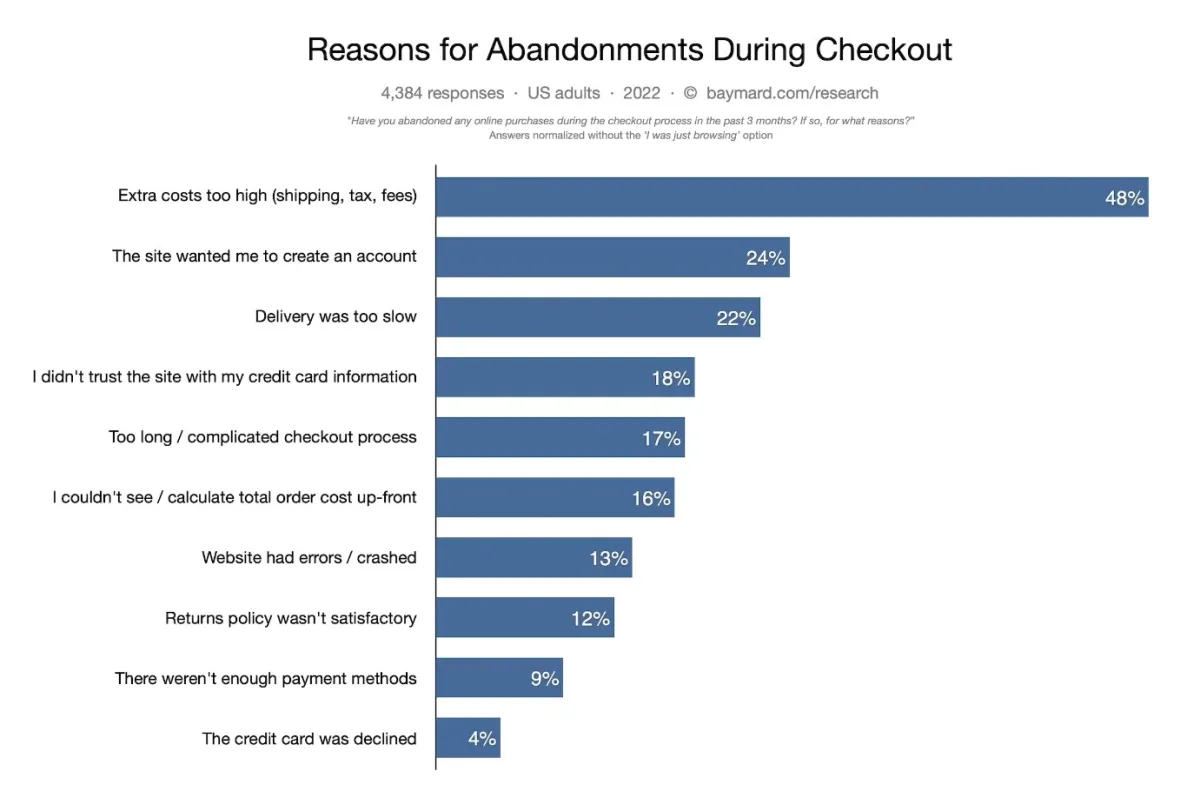
5. Healthcare
Image classification is used to leverage computer vision technology in the healthcare and radiology sectors. Medical images like X-rays, MRIs, and CT scans can be automatically analyzed using image classification. This enables medical personnel to diagnose and treat patients more accurately and efficiently. Medical image annotation tools usually include image classification features.
For instance, pneumonia is a lung disease that is difficult to detect early by traditional diagnosis methods. An image classification algorithm trained on a dataset of chest X-ray images can be used to predict and accurately detect2 the presence of pneumonia in new X-rays.
X-ray recognition and classification through deep learning:
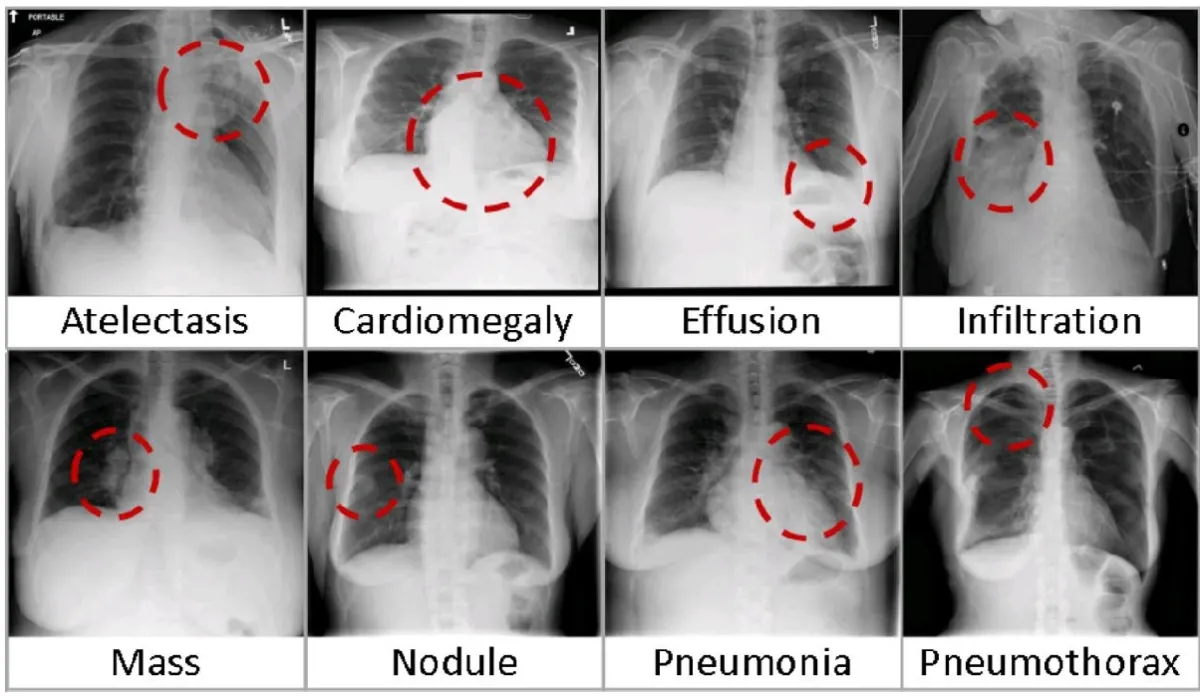
6. Security
Image classification can also be used to leverage computer vision in the security sector in the following ways:
- Surveillance: It can help in automatically identifying and categorizing objects in surveillance footage. This can help security personnel detect and respond to potential threats more quickly.
- Facial recognition: It can also help authorities identify people of interest in crowds. Image classification can help the security sector leverage computer vision technology in areas such as banks, airports, and other crowded places.
Example: Here is how an image classification system categorizes faces in an image:
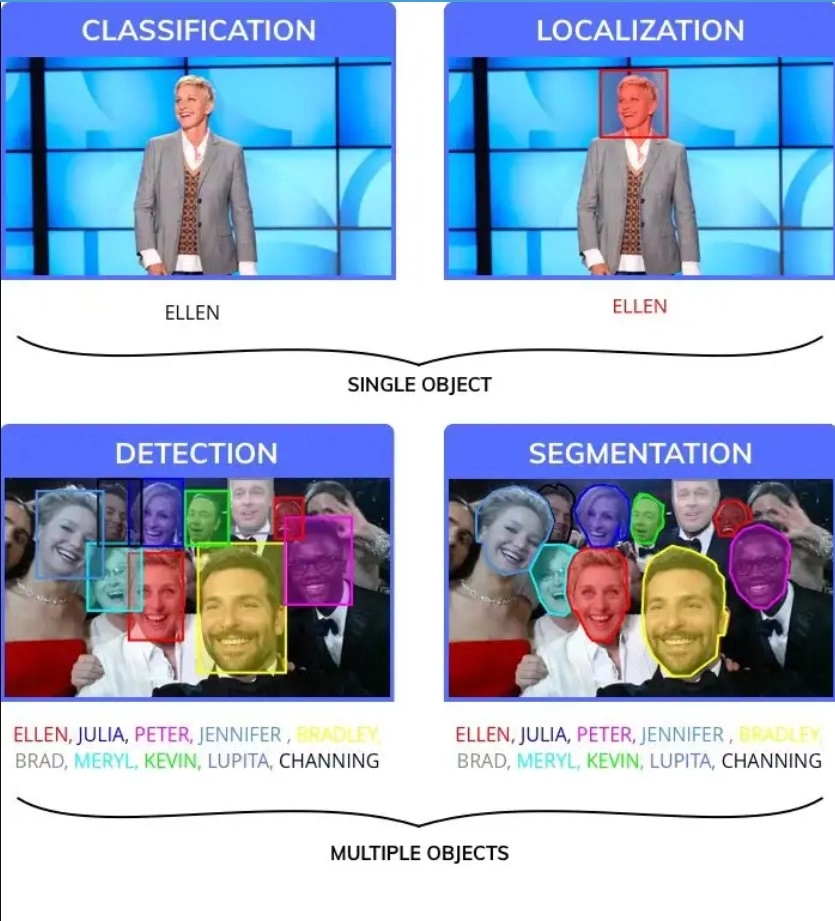
Then, a computer vision system uses the classified data to identify people in a surveillance system:
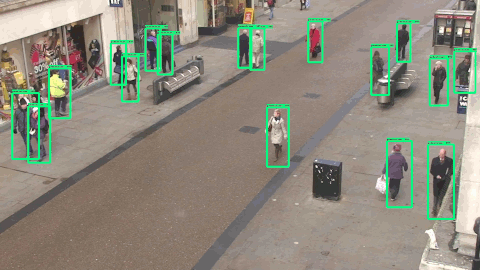
Best practices in developing an image classification tool
1. Data collection
Data collection is one of the most important steps in developing an automated image classifier model. Ensuring the quality and diversity level of the training data is crucial since they directly impact the performance of the model. The dataset should be large-scale and representative of the sample of images that cover the complete range of categories to be classified.
For instance, a company developing an image classification tool for fashion retail will require different variations of clothing images to be trained.
2. Data preprocessing
Preprocessing the training data can greatly improve the classification model’s performance. This includes:
- Resizing images
- Removing noise
- Augmenting it for more diversity
For instance, a healthcare organization pre-processes X-ray image data to ensure they are consistent in size and position. They might also apply filters to reduce noise and enhance the key features in the images. This will result in more accurate classifications by the automated tool.
3. Model selection
Image classification can be done using a wide variety of machine-learning algorithms. It is important to understand the problem and the pros and cons of each algorithm before choosing the best for the project.
For example, a surveillance company can select a convolutional neural network (CNN) as the model for their image classification tool since it is suitable for identifying objects in visual imagery.
4. Hyperparameter tuning
The performance of the model can be significantly impacted by tweaking its parameters. It’s important to tune the hyperparameters to find the best-performing model for the project.
For example, a retail company can improve the image classification model’s accuracy and reduce the amount of time required for training by tuning the:
- learning rate,
- batch size,
- And the number of epochs (Total number of iterations).
To learn more about the different methods and tools of hyperparameter Optimization, check out this article.
Further reading
- Image Annotation: Definition, Importance & Techniques
- 7 Data Transcription Services & Their Selection Criteria
References
- Broz, M. (2023). “Number of Photos (2023): Statistics, Facts, & Predictions.” Photutorial. Accessed: Feb 08, 2023.
- Mabrouk et al (2022). “Pneumonia Detection on Chest X-ray Images Using Ensemble of Deep Convolutional Neural Networks.” Applied Sciences, 12(13), 6448. Accessed: Feb 08, 2023.
Comments
Your email address will not be published. All fields are required.