Radiology teams are under pressure from growing scan volumes, staff burnout, and the risk of diagnostic mistakes. These challenges are making it harder to deliver timely and accurate care.
AI is helping to ease the burden by accelerating image analysis, minimizing errors, and facilitating more informed decisions.
Explore the top 6 radiology AI use cases to help healthcare leaders understand where AI can make a practical difference and guide smart investment choices.
Top 6 use cases of radiology AI
1. Breast cancer detection
Breast cancer is the most common type of cancer in the world, as an estimated 310,720 women will receive a diagnosis of invasive breast cancer, with around 16% of cases occurring in women under the age of 50.1 Mammography is the primary method for breast cancer screening. However, 40% of cancers are missed by doctors while evaluating mammograms.2
To overcome this issue, computer vision technology is being introduced to the healthcare sector. AI-enabled computer vision and medical imaging systems can accurately analyze mammograms to detect cancers that are not visible to the human eye.
The machine learning models are trained on medical image data to provide a more accurate analysis of breast masses, breast density, and mass segmentation, as well as better cancer risk assessments.
However, current machine learning algorithms and models are not mature enough to detect all types of cancers. There are some cancers that are found by doctors and missed by the ML model.
Therefore, while implementing this technology in a healthcare facility, managers should not try to replace doctors but instead implement this technology to complement and assist doctors in enabling computer-aided detection of breast cancer.
See the video below for research by Google and Northwestern Medicine on detecting breast cancer:
For example, the NHS is set to begin the world’s largest trial of AI-assisted breast cancer screening, aiming to evaluate whether artificial intelligence can match radiologists in detecting signs of the disease.
Over the next few years, five AI systems will analyze approximately 462,000 of the 700,000 mammograms conducted in England, with the remaining 238,000 mammograms to be reviewed by two radiologists as per current practice.
If successful, the trial could replace the “second reader” system with AI, easing the burden on overstretched radiologists and speeding up diagnosis. The £11 million initiative, backed by the Department of Health and Social Care, is part of a broader push to modernize cancer care, including a forthcoming national cancer plan.3
Another example is iCAD, which utilizes machine learning algorithms to analyze mammograms, detecting early signs of breast cancer and assisting radiologists in making more accurate diagnoses. This advancement has contributed to better patient outcomes and a reduction in false-positive diagnoses.
iCAD’s Breast Health Solutions provides advanced software for digital breast tomosynthesis (DBT), breast density assessment, 2D mammography, and personalized risk evaluation.4
2. Tumor classification
Classifying tumors is an important part of tumor diagnosis and treatment since it helps doctors and surgeons decide which course of action to take. However, it is a time-consuming process.
AI-powered medical imaging tools can be used to analyze medical images, such as MRI and CT scans, to detect tumors and other abnormal cell structures accurately and quickly. These AI systems also have the ability to detect tumors even in complex brain imaging scenarios.
For example, researchers at Charité, Universitätsmedizin Berlin, have developed an AI model called crossNN that can accurately detect and classify over 170 types of cancer using the epigenetic fingerprint of tumors.
This non-invasive approach analyzes genetic material, such as cerebrospinal fluid, making it especially useful for cases where biopsies are too risky, like brain tumors.
The AI compares unknown tumor data with thousands of reference profiles using a neural network trained on over 8,000 samples, achieving up to 99% accuracy for brain tumors and 978% across all cancers.
Unlike traditional diagnostics that rely on tissue examination, crossNN uses DNA methylation patterns, which are unique to each tumor type. The technology is designed to be both highly reliable and explainable, which is essential for clinical use.5
Another study conducted in the UK found AI and advanced imaging effective in diagnosing brain tumors in children with non-invasive methods.6
See how it works:
3. Finding hidden fractures
Another application of AI in radiology is the detection of bone fractures that are difficult to visualize with the human eye. AI-powered medical imaging tools can help detect hidden bone fractures by reducing noise and providing more accurate representations of the radiological images.
Machine learning algorithms can be trained to recognize patterns in radiological images that may signify a fracture or other anomaly, and then alert medical professionals to investigate further. AI radiology tools are also capable of identifying subtle characteristics of a fracture that may be otherwise undetected by radiology professionals.
AI-enabled medical imaging systems can also detect hip fractures, which are usually hard to spot due to the soft tissues in the hip. However, such solutions can only enable computer-aided detection of anomalies and not work autonomously.
For example, Radiobotics’ AI-powered tool RBfracture has been approved for NHS use by NICE (National Institute for Health and Care Excellence) as one of four AI technologies supporting fracture detection on X-rays in urgent care.
RBfracture demonstrated improved diagnostic sensitivity, from 74% to 83%, without sacrificing specificity, thereby addressing challenges such as missed fractures, staff shortages, and clinician fatigue, particularly in smaller or rural centers.7
A systematic review highlights the effectiveness and promise of AI technologies in enhancing diagnostic accuracy and efficiency across different fracture types and imaging techniques.
Research indicates that advanced AI models, including convolutional neural networks (CNNs) such as InceptionNet, VGG16, and ResNet, frequently outperform human radiologists in terms of accuracy, sensitivity, and specificity. These models have been effectively utilized to identify and classify fractures in areas such as the proximal humerus, hip, chest radiographs, and dental structures.8
4. Detecting neurological abnormalities
AI can also be used to detect neurological disorders and illnesses, such as dementia, Alzheimer’s, Parkinson’s, etc.
For example, a recent study introduced an AI tool based on a convolutional neural network that can recognize speech patterns in Alzheimer’s patients’ conversations to make diagnoses with 95% accuracy. Since the language pattern of an Alzheimer’s patient changes, the AI model can detect that.9
Watch how this company uses AI to analyze patient data and make early diagnoses of Alzheimer’s and dementia. The application then refers the patient to a general physician to make further assessments and complete the diagnosis.
5. Automated lesion detection
Lesions are irregularities in radiological images that could indicate a problem. AI and machine learning algorithms are now being utilized to detect lesions more quickly and accurately.
With automated lesion detection powered by AI systems, such as deep learning networks, medical professionals can detect lesions from radiology scans earlier, leading to improved diagnostic accuracy and early intervention for patients in need of treatment options sooner rather than later.
For example, a recent study compared the detection of prostate cancer using conventional versus AI-assisted interpretation of multiparametric MRI (mpMRI) in 53 patients.
Two radiologists analyzed scans using the PI-RADS v2.1 framework, both with and without AI support, which included gland segmentation and lesion probability scoring. Pathology from biopsies served as the diagnostic reference. Results showed that AI significantly enhanced the detection of clinically significant prostate cancer (csPCa), improving diagnostic accuracy for both radiologists.10
6. Lung cancer screening
The use of radiology AI is becoming increasingly important for early lung cancer screening. AI-powered medical imaging tools can help to detect subtle signs and abnormalities that would otherwise go undetected by radiological methods alone.
This improved detection can lead to earlier diagnosis, which can improve survival rates among those with lung cancer. Studies show that such computer-aided detection systems can help reduce labor work in the following lung cancer diagnosis processes:11
- Low-dose computed tomography (LDCT)
- Chest radiographs (CXR)
- Pathology slide reading
- Nodule detection
A recent example from ECR 2025 is Median Technologies’ introduction of eyonis LCS, an AI-powered software that enhances early lung cancer detection by analyzing low-dose CT scans. Designed to assist radiologists in identifying and characterizing suspicious lung nodules, the tool aims to boost diagnostic accuracy and reduce false positives.
Tested in two major clinical trials (REALITY and RELIVE), eyonis™ LCS showed statistically significant improvements in detecting early-stage lung cancer. The software is now awaiting FDA 510(k) and CE mark approvals, expected by mid-2025, ahead of its planned commercial launch.
Beyond detection, eyonis™ LCS addresses radiologist variability and integrates into clinical workflows, offering a scalable solution for lung cancer screening programs across the U.S. and Europe.12
Another example, Rayscape’s Lung CT solution, is designed to assist radiologists by automatically identifying lung nodules ranging from 3 to 30 mm in diameter.
The system’s automated nodule detection capabilities offer high sensitivity and specificity, aiding in the early identification of potential lung cancers.
Additionally, Rayscape’s AI comparative tool can detect multiple nodular lesions and alert doctors to growing lung nodules, which may be indicative of malignancy.13
Figure 1: An example from qTrack lung nodule management platform on patient tracking.14
What is AI for Radiology?
Artificial intelligence is transforming radiology by increasing the efficiency and accuracy of medical imaging across various clinical settings. AI-driven radiology solutions leverage deep learning algorithms, machine learning models, and AI technologies to automate image analysis, detect abnormalities, and support radiologists in making faster, more informed decisions.
Due to the complex nature of medical imaging, AI has the potential to significantly improve diagnostic accuracy, reduce errors, and optimize clinical workflows, leading to better patient outcomes. Check out healthcare AI use cases for more examples.
Key benefits of AI in radiology
Automated image analysis
AI algorithms rapidly process vast amounts of imaging data, aiding in the identification of abnormalities that might be difficult for the human brain to detect.
Enhanced diagnostic accuracy
AI models help reduce false positives, false negatives, and missed detections, ensuring critical findings are not overlooked.
Improved patient care
Faster and more precise diagnostics allow for urgent cases to be prioritized, improving overall patient outcomes.
Optimized drug discovery
Beyond imaging, AI contributes to drug discovery by analyzing radiological data to track disease progression and assess treatment efficacy. Check out similar use cases of generative AI in healthcare.
Why is AI important for radiology and healthcare?
Radiology already depends on advanced imaging technologies like X-rays, CT scans, MRI, and PET scans to diagnose and monitor diseases. However, the increasing volume of medical imaging data has placed significant pressure on radiologists.
Radiology AI solutions help manage existing workflows, enhancing the speed and precision of radiology reports and reducing physician burnout. These solutions are categorized based on task type, repetitive, quantitative, diagnostic, or explorative, across 119 software (See Figure 1):
- Repetitive tasks focus on high-volume, low-complexity processes like lung nodule detection or multiple sclerosis lesion tracking.
- Quantitative tasks involve measurements, such as emphysema volume in chest CTs or bone density analysis.
- Explorative algorithms enable radiologists to select an image region and find similar areas in other scans with associated diagnoses.
- Diagnostic tasks encompass most remaining algorithms, including pneumonia detection in chest X-rays, breast lesion classification, and liver tumor grading.
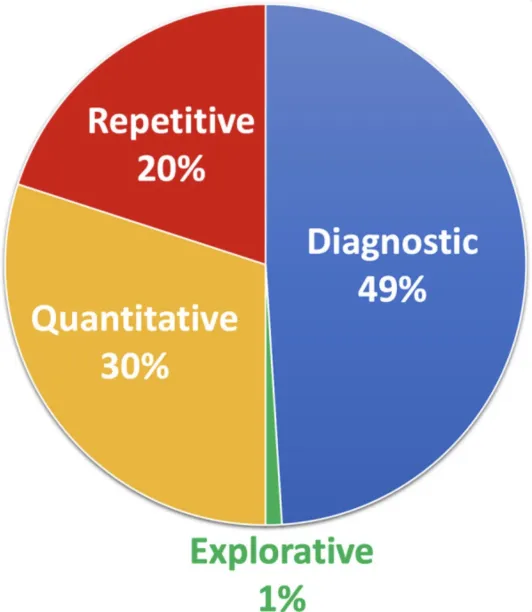
Figure 2: 4 areas of radiology AI offerings: Repetitive is 20%, diagnostic is 49%, quantitative is 30% and explorative is only 1 %.15
AI’s role: Complementing human expertise, not replacing it
Despite its capabilities, AI is not meant to replace radiologists or make independent medical decisions. Instead, AI-driven radiology should be seen as an assistive technology that enhances human performance by providing second opinions, cross-referencing medical cases, and reducing workload in clinical practice.
AI developers and AI vendors continue to refine AI models to ensure they function as reliable assistants rather than autonomous decision-makers.
The future of radiology AI
The future of AI in radiology is promising, with ongoing research and development leading to more sophisticated deep learning models and improved AI-assisted image acquisition techniques.
By refining AI algorithms, advancing AI technologies, and fostering collaboration between radiologists, professors, and AI researchers, the healthcare sector can harness the full potential of AI to revolutionize diagnosis, analysis, and patient care.
Challenges and limitations of AI in radiology
While AI-driven radiology offers significant advancements in medical imaging, diagnostic accuracy, and clinical workflows, its adoption comes with several challenges and limitations. Key concerns include bias in AI models, regulatory hurdles, and high implementation costs, all of which impact the technology’s reliability, accessibility, and scalability in clinical settings.
Bias in AI models: Limited and non-diverse training data
Many AI algorithms are trained on datasets predominantly sourced from Western populations, leading to potential biases in detecting and diagnosing diseases across diverse ethnicities and demographics.
This lack of representation may result in disparities in patient care, where AI systems perform well in certain populations but demonstrate reduced diagnostic accuracy for others.
To address this issue, AI developers and AI vendors must prioritize training deep learning models on more inclusive, globally representative datasets to ensure equitable AI results.
Regulatory challenges
The integration of AI-driven radiology into clinical practice can be slowed by strict regulatory approvals, such as FDA clearance, CE marking, and other global compliance standards.
Regulatory agencies require advanced testing, validation, and review to ensure AI solutions meet safety and efficacy standards. These delays in approval processes can hinder the widespread deployment of AI technologies, limiting their immediate impact on radiology and patient outcomes.
By working with policymakers, healthcare organizations can manage regulatory pathways to ensure efficient testing and validation without compromising security and patient care.
High implementation costs and resource requirements
Deploying AI models in clinical workflows requires substantial financial investment, including hardware upgrades, software integration, and staff training.
Many healthcare institutions, especially those with limited budgets, find it challenging to justify the cost of AI adoption compared to traditional radiology systems. Additionally, maintaining AI-powered solutions involves continuous data updates, security measures, and algorithm refinements, further increasing operational expenses.
Lower implementation barriers through cost-effective AI deployment models and AI vendor partnerships that provide scalable, budget-friendly solutions can help overcome this challenge for clinical settings.
Key considerations for AI adoption in radiology
While investing in AI-powered solutions to improve your radiology facility, the following points can be considered:
- Consider the adequacy of existing radiology processes and how incorporating AI can help improve them. This involves mapping out how such solutions can be integrated into the clinical workflow.
- Medical training data sets are difficult to find. Therefore, it is important to consider if enough digital data is available to implement the system and which ones could benefit from the use of AI/ML algorithms.
- Identify experts or consultants in the radiology field who can provide valuable insight into the best ways to implement AI-powered radiology systems.
- Ensure that your radiology facility is ready to invest in the hardware and technical expertise needed for AI-powered medical imaging.
- Since AI-powered radiology solutions can not operate autonomously, ensure that the staff in your radiology facility is trained and equipped to leverage such tools.
- Legal and ethical issues can be a barrier while implementing AI-powered solutions in the healthcare sector. Therefore, we recommend going through the country-specific regulations regarding using AI-powered solutions in a radiology facility (such as data collection regulations), to avoid lawsuits related to legal and ethical issues.
External Links
- 1. Breast Cancer Facts and Statistics 2025. Breastcancer.org
- 2. https://www.genengnews.com/news/artificial-intelligence-may-potentially-improve-breast-cancer-screening/
- 3. NHS to launch world’s biggest trial of AI breast cancer diagnosis | Breast cancer | The Guardian. The Guardian
- 4. AI Mammography for Patients | iCAD. iCAD
- 5. Tumor diagnostics: AI model detects more than 170 types of cancer. Medical Xpress
- 6. https://www.itnonline.com/content/child-brain-tumors-can-be-classified-advanced-imaging-and-ai
- 7. RBfracture approved for NHS use by NICE. Radiobotics
- 8. https://pmc.ncbi.nlm.nih.gov/articles/PMC11394268/
- 9. TechTarget Enterprise Technology News | Health IT.
- 10. Impact of artificial intelligence assisted lesion detection on radiologists' interpretation at multiparametric prostate MRI - Clinical Imaging.
- 11. https://pmc.ncbi.nlm.nih.gov/articles/PMC8946647/
- 12. ECR 2025: AI-powered Lung Cancer Screening - MedicalExpo e-Magazine. MedicalExpo e-Magazine
- 13. Rayscape | Radiology AI.
- 14. qLC - AI for early detection of Lung Cancer.
- 15. The State of Radiology AI: Considerations for Purchase Decisions and Current Market Offerings - PMC .
Comments
Your email address will not be published. All fields are required.