As healthcare systems face rising data volumes, workforce shortages, and increasing demands for personalized care, generative AI is emerging as a critical solution.
By generating insights from complex medical data, generative AI healthcare applications offer hospital administrators, clinicians, and researchers new ways to improve decision-making and patient outcomes.
Explore how generative AI is applied across healthcare delivery, administration, and population health management, along with the challenges and future directions shaping its adoption.
Area | Use Cases | Examples |
---|---|---|
Healthcare Delivery | Synthetic medical imaging Personalized treatment planning | - GANs for synthetic X-rays, - LLMs for drug discovery - Genomic analysis and customized rheumatoid arthritis treatments |
Healthcare Administration | Claim pricing Clinical guideline support Fraud detection Admin automation | - GPT-4 in EHRs - Nuance DAX Copilot to document patient visits with generative AI |
Population Health | Data synthesis Trend prediction Risk group segmentation | - Diagnostic Robotics predictive analytics to reduce ED visits, increase ROI, and personalize care strategies. |
Public Health Initiatives | Targeted campaigns Resource planning Preventive care Education | - AI-guided breast cancer screening - Simulated interventions and mobile health deployment planning |
Improving healthcare delivery
1. Create new medical images
Generative AI, especially models like Generative Adversarial Networks (GANs), can be trained to generate synthetic medical images that mimic real-world X-rays, MRIs, or CT scans. These images that are created with synthetic data, have several important applications in healthcare:
- Medical training & education: AI-generated images can be used to train healthcare professionals by creating diverse datasets of rare diseases, anomalies, or normal variants that may not always be present in real-world cases.
- Data augmentation for AI models: Training AI systems to diagnose medical conditions requires large datasets. Generative AI can produce synthetic but highly accurate images to augment limited datasets to improve the accuracy of diagnostic models without compromising privacy.
- Simulation and research: Synthetic images can help researchers simulate various scenarios (such as how a disease might progress) or test AI algorithms without waiting for new patient data. This process can support accelerating medical research.
- De-identification of data: By generating synthetic images that retain key clinical features but do not belong to any actual patient, healthcare systems can share these images without violating privacy laws like HIPAA, therefore facilitating broader research and collaboration.
Research has shown the effectiveness of synthetic images in medical imaging analysis. For example, a study in Nature Biomedical Engineering demonstrated that GAN-generated synthetic retinal images were just as effective as real images in training a deep learning model for diabetic retinopathy detection.1
Despite the advancement with synthetic medical data, there are still limitations such as privacy and ethical considerations. To overcome these challenges, a 2024 study, MAISI (Medical AI for Synthetic Imaging), utilized diffusion models to generate high-resolution synthetic 3D CT images.
The experimental findings demonstrate that MAISI can generate lifelike, anatomically precise images across various body regions and conditions, highlighting its strong potential to address challenges through the use of synthetic data (See the image below).
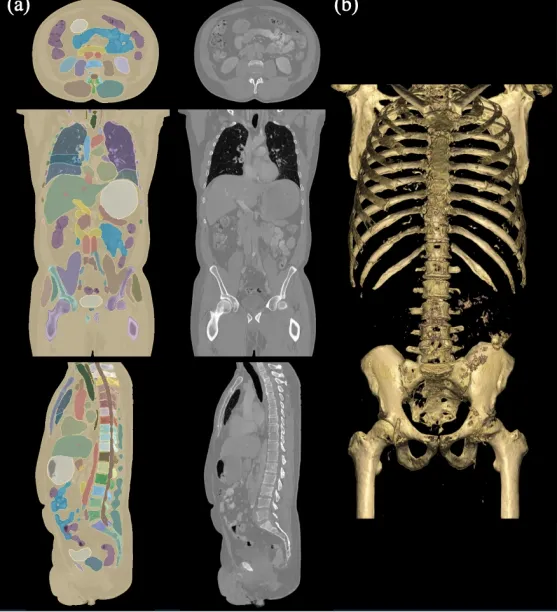
Figure 1: (a) A high-resolution CT scan generated by MAISI with its segmentation overlay, shown in axial, sagittal, and coronal views. (b) A 3D rendering focused on bone structures, highlighting the realism of the generated scan.2
Another study on creating new medical images with generative AI models focused on X-Diffusion, a novel approach that reconstructs full 3D MRI scans using just one or a few 2D slices, greatly accelerating scan times and reducing costs.
Unlike traditional methods that rely on complete 3D data or treat MRIs as separate 2D slices, X-Diffusion learns from entire 3D volumes during training (See the image below). It outperforms existing techniques in image quality and accuracy, preserves critical anatomical details, and even generalizes to new body regions it wasn’t trained on.
This development is expected to make high-resolution MRI imaging faster, more affordable, and more widely accessible.
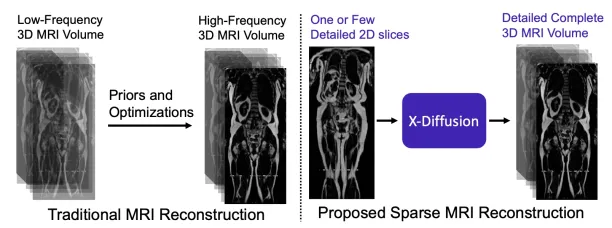
Figure 2: A comparison between traditional MRI reconstruction vs X-Diffusion.3
2. Generate personalized treatment plans
Generative AI models can analyze a patient’s comprehensive medical history, genetic profile, lifestyle factors, and even real-time health data (e.g., from wearables such as smartwatches) to create personalized treatment plans. Here’s how this works:
- Deep patient analysis: AI systems trained on large-scale medical records can identify patterns in a patient’s history, such as past diagnoses, responses to treatments, or genetic predispositions. Generative AI tools can then generate a treatment plan tailored to the patient’s unique circumstances.
- Integrating real-time data: AI can pull in real-time health data from sources like wearable devices, lab tests, and continuous monitoring systems. Based on this data, the system can adjust or generate new treatment recommendations to ensure the patient is always on the most effective course of treatment.
- Predictive treatment: By generating models that simulate how a patient might respond to various treatments based on historical and clinical data, generative AI tools can suggest options that maximize the likelihood of success. For example, if a patient has a specific genetic mutation associated with a poor response to a drug, these tools could suggest alternatives beforehand.
- Automating complex decision-making: Complex conditions such as cancer often require multi-modal treatments involving surgery, chemotherapy, and targeted therapies. Generative AI tools can assist by creating treatment schedules, predicting side effects, and coordinating multi-disciplinary care tailored to the patient’s evolving condition.
- Personalized medication: AI can recommend personalized dosages or medication types based on the patient’s metabolic profile, reducing the risk of adverse drug reactions or inefficient treatments.
Real-life examples:
In 2024, Mayo Clinic partnered with Cerebras Systems to develop AI models that analyze genomic data from over 100,000 patients. These models aim to predict individual responses to treatments, such as assessing the efficacy of methotrexate in rheumatoid arthritis patients, enabling more tailored therapeutic strategies.4
Google Cloud and Ginkgo Bioworks have collaborated to launch a new protein large language model (LLM) and a supporting API, designed to transform the drug discovery process.
Built on Google Cloud’s Vertex AI platform, these tools utilize Ginkgo’s biological data to help researchers analyze protein structures and interactions. This approach allows pharmaceutical and biotech companies to accelerate the identification of therapeutic targets while improving the development of new medicines.5
Supporting healthcare administration
Generative AI tools play a crucial role in managing various administrative functions within healthcare. These technologies can be applied across multiple areas to enhance efficiency, accuracy, and overall healthcare delivery.
3. Claim pricing
Generative AI technologies can support claim pricing in the insurance and healthcare sectors by automating the review of complex contracts.
Traditionally, determining the appropriate pricing for claims requires detailed analysis of various contractual terms, conditions, and coverage details, which can be prone to manual errors and inefficiencies. AI models can quickly and accurately process these documents, cross-reference relevant policy information, and calculate claim prices.
This process leads to faster and more accurate claim settlements while minimizing disputes and improving both the operational efficiency and the customer experience in healthcare delivery.
4. Clinical guidelines navigation
Generative AI in healthcare enables providers to compare patient data with clinical guidelines, thereby enhancing diagnostic accuracy and improving healthcare outcomes. These AI tools support clinical decision-making by leveraging natural language processing to analyze electronic health records (EHRs).
Real-life example:
Microsoft’s Sequential Diagnosis Benchmark (SD Bench) uses 304 complex cases from the New England Journal of Medicine to test how AI and physicians navigate diagnostic challenges, ask questions, order tests, and manage costs.
Central to this is the Microsoft AI Diagnostic Orchestrator (MAI-DxO), which coordinates multiple large language models (like GPT, Llama, Claude, Gemini, Grok, and DeepSeek) as a virtual team of doctors to deliver accurate, cost-aware diagnoses.
MAI-DxO, paired with OpenAI’s o3, achieved over 85% accuracy, far surpassing the physicians’ 20% average, while reducing unnecessary testing costs.
The system combines both breadth and depth of medical expertise, offering potential to lower healthcare waste and empower both clinicians and patients.6
Figure 3: The graph illustrating multiagent orchestration in SDBench shows how the Gatekeeper, Diagnostic, and Judge agents collaborate to handle diagnostic questions and evaluate final diagnoses against NEJM case records.7
Real-life example:
In 2024, Epic integrated GPT-4 into its Electronic Health Records (EHR) system through a partnership with Microsoft. This integration assists clinicians by providing AI-generated responses to patient messages and suggesting relevant clinical guidelines, thereby enhancing decision-making and patient communication.8
5. Fraud detection
AI models with machine learning technology can identify patterns in claims and patient data to improve fraud detection. These tools analyze medical images, discharge summaries, and lab results to support the healthcare sector’s ability to mitigate fraud while addressing data privacy concerns.
6. Medical record analysis
Generative AI models in healthcare settings can extract insights from unstructured clinical documentation, such as EHRs and CT scans. This improves diagnostic accuracy and healthcare providers’ ability to make informed decisions.
The technology also holds promise in analyzing medical conditions and clinical trials by using training data to fine-tune models in a controlled environment.
7. Increase administrative efficiency
Generative AI tools can help reduce the administrative burden in the healthcare industry by automating tasks like clinical documentation and data collection.
AI in healthcare helps medical professionals focus on patient care, enabling efficient healthcare delivery and improving health outcomes.
Real-life examples:
Stanford Health Care has adopted Nuance’s DAX Copilot, an AI-powered tool by Microsoft, to automate clinical documentation during patient visits. This technology reduces administrative burdens, helping to address physician burnout while improving care access and documentation quality.
Using ambient and generative AI, DAX Copilot generates clinical notes from exam conversations, allowing doctors to focus more on patients. Early results show strong physician satisfaction and time savings.9
Oscar Health integrates generative AI, specifically o1-preview, across multiple administrative functions to improve efficiency and accuracy. It automates claim pricing by reviewing complex contracts, assists clinicians by comparing patient data to clinical guidelines, and enhances fraud detection by identifying anomalies in claims.
Moreover, Oscar AI extracts valuable insights from unstructured clinical data, such as EHRs, improving diagnostic precision.
By automating administrative tasks such as clinical documentation, Oscar AI reduces the burden on healthcare providers, allowing them to focus more on patient care and improving healthcare delivery.10
Population health management
Generative AI can significantly enhance population health management by providing deeper insights into demographic trends and enabling the design of tailored interventions:
8. Access to more detailed demographic information
By synthesizing data from multiple sources, such as electronic health records (EHRs), insurance claims, social determinants of health, and public health databases, AI provides a comprehensive view of population health dynamics.
In areas with sparse healthcare data (e.g., rural or underserved communities), generative AI can also generate realistic synthetic data to fill gaps, providing a more comprehensive picture of population health and informing intervention strategies.
9. Predicting health trends
AI models can forecast health trends across different demographic groups, predicting the likelihood of chronic diseases or outbreaks. This allows policymakers to anticipate healthcare needs and allocate resources effectively.
10. Segmentation and personalization
AI-generated detailed profiles of different population segments help identify high-risk groups or communities requiring specialized attention. This insight can assist in targeting interventions based on factors like age, ethnicity, income, or geography.
Real-life example:
Diagnostic Robotics’ AI-powered population health management platform enables value-based care by identifying patients at risk of preventable conditions. Predictive analytics allows pinpointing care gaps and enables proactive intervention.
It also integrates into health plans to enhance care management workflows, enable cost reductions, and improve ROI, achieving a 2.9x return. The platform supports improved health outcomes, such as a 25% reduction in emergency department event rates.11
Designing targeted public health initiatives
Generative AI can also help design more effective and targeted public health campaigns tailored to the specific needs of underserved or vulnerable communities:
11. Customized campaigns
AI can analyze demographic and cultural factors to create personalized public health messages for campaigns such as smoking cessation, vaccination, or disease prevention to ensure they resonate with different populations.
12. Optimizing resource allocation
By simulating various public health scenarios, AI helps policymakers evaluate the impact of different interventions while allowing for resource allocation where it is most needed, particularly in hard-to-reach areas.
13. Addressing health disparities and increasing healthcare access
AI can identify hidden health disparities by analyzing how social determinants of health (such as income, education, or housing) affect various populations. This insight can guide initiatives to reduce disparities and improve access to preventive care.
AI tools can also identify areas most in need of healthcare infrastructure, guiding the placement of clinics, telemedicine services, or mobile health units.
14. Tailoring preventive care programs
AI-driven analysis can design preventive care programs, such as screenings for chronic conditions, targeting at-risk populations, leading to earlier interventions and reduced long-term healthcare costs.
15. Enhancing health education and awareness
Generative AI can simulate different methods of delivering health education, helping to develop culturally sensitive strategies that are well-received by various communities.
Real-life example:
Google Health is conducting a study with Northwestern Medicine to assess the effectiveness of AI in breast cancer screening. The AI model flags high-risk mammograms for immediate radiologist review, potentially speeding up diagnosis. Women flagged by AI may receive same-day additional imaging, which is expected to shorten the typical waiting period.
This approach shows AI’s ability to match or exceed clinician accuracy in analyzing mammograms and create personalized treatment plans accordingly.12
How does generative AI work in healthcare?
Generative AI algorithms use deep learning techniques/machine learning models to learn from large amounts of data and generate new content similar to the input data.
Generative AI in healthcare works by using advanced AI models, such as large language models and foundation models, trained on extensive datasets from electronic health records (EHRs), medical images, and other clinical data.
These generative AI models analyze patient data, apply natural language processing to extract insights, and assist in clinical decision making.
They help improve diagnostic accuracy, streamline administrative tasks, and enhance healthcare delivery by automating clinical documentation and reducing administrative burden for medical professionals in healthcare settings.
They also support drug development and clinical trials by analyzing proprietary data.
For successful implementation, healthcare organizations must ensure that AI applications, data availability, and privacy laws are upheld to gain consumer trust and widespread implementation across the health care sector.
What are some potential challenges of generative AI healthcare?
While there are many potential benefits to using generative AI in healthcare, there are also some possible challenges and drawbacks. Some examples include:
- Privacy and security: Patient privacy is strictly regulated. The use of generative AI in healthcare also raises concerns about protecting patient privacy, sensitive medical data, and the potential for misuse or unauthorized access to healthcare data.
- Bias and discrimination: Generative AI algorithms can be prone to bias and discrimination, especially if they are trained on healthcare data that is not representative of the population they are intended to serve. This can result in unfair or inaccurate medical diagnoses or treatment plans for underprivileged groups, such as women or non-white races.
- Misuse and over-reliance: If generative AI algorithms are not used properly, they can lead to incorrect or harmful medical decisions. Additionally, there is a risk that healthcare providers may become overly reliant on these algorithms, losing the ability to make independent judgments.
- Ethical considerations: The use of generative AI in healthcare raises several ethical concerns, such as the potential impact on employment in the healthcare sector.
The future of generative AI and its impact on healthcare
The future of generative AI in healthcare is likely to be highly significant as the technology continues to advance and become more widely adopted. Some potential future developments include:
- More sophisticated algorithms: Machine learning algorithms are likely to become increasingly refined over time, with enhanced ability to analyze large amounts of healthcare data and identify patterns and trends. This will enable healthcare providers to make more accurate and personalized diagnoses and treatment plans.
- Broader applications: Generative AI is likely to be utilized in a wider range of healthcare settings and for a more comprehensive range of applications, including predictive modeling of disease outbreaks and drug discovery.
- Better integration with other technologies: Generative AI will likely be integrated with other technologies(e.g., medical imaging and wearable health devices) to provide more comprehensive and personalized patient care.
- Increased collaboration: Collaboration between healthcare providers, researchers, and technology companies to develop and implement generative AI algorithms in healthcare settings is expected to increase.
External Links
- 1. Generative AI for medical imaging analysis and applications | Published in Future Medicine AI.
- 2. https://arxiv.org/pdf/2409.11169
- 3. https://arxiv.org/pdf/2404.19604
- 4. JPM24: Mayo Clinic details AI plans, new partnership. Fierce Healthcare
- 5. Exclusive: Google Cloud And Ginkgo Bioworks Partner To Launch New Protein LLM and API.
- 6. The Path to Medical Superintelligence | Microsoft AI. Microsoft AI
- 7. https://arxiv.org/pdf/2506.22405
- 8. Epic to Integrate GPT-4 into Its EHR Through Expanded Microsoft Partnership - MedCity News.
- 9. DAX Copilot to Automate the Creation of Clinical Documentation, Reduce Physician Burnout, and Expand Access to Care Deployed Enterprise-Wide at Stanford Health Care - Mar 11, 2024.
- 10. Oscar Health’s early observations on OpenAI o1-preview — OscarAI.
- 11. Diagnostic Robotics | AI-Powered Population Health Management.
- 12. Using artificial intelligence in breast cancer screening. Google
Comments
Your email address will not be published. All fields are required.