Data labeling, the process of annotating raw data (such as images, text or audio), is essential for training ML models to perform tasks like classification and recognition. Here, we introduce top 20 data labeling tools.
The top data labeling tools:
Name of Tool | Data Type | Open or Closed Source |
---|---|---|
Label-studio | Audio, Image, Text, Time Series | Open |
Universal-data-tool | Audio, Image, Text, Time Series | Open |
awesome-data-labelling | Audio, Image, Text, Time Series | Open |
doccano | Text, Time Series | Open |
Scale | Audio | Closed |
Audio-annotator | Audio | Open |
Audino | Audio | Open |
SuperAnnotate | Image | Closed |
Labelbox | Image | Closed |
V7 | Image | Closed |
Dataloop | Image | Closed |
Telus International | Image | Closed |
supervise.ly | Image | Closed |
VoTT | Image | Open |
ImgLab | Image | Open |
CVAT | Image | Open |
LightTag | Text | Closed |
PDF Annotator | Text | Closed |
Drawboard | Text | Closed |
dataqa | Text | Open |
Ranking: From most to least comprehensive.
What is a data labeling tool?
A data labeling tool is software that can find raw data in image, text, and audio formats and help data analysts label data according to specific techniques such as bounding box, landmarking, polyline, named entity recognition, etc., to prepare high-quality data for ML model training. Each data type requires different features and labels.
Why are data labeling tools important?
Today’s businesses rely on AI/ML-driven decisions to make profits. Labeling data is one of the most important steps in training ML models. McKinsey argues that data labeling is the biggest challenge in building effective ML models. As mentioned earlier, businesses need a software program that specializes in labeling data.
To make successful predictions, ML models need high-quality data. The training process for ML models is no different from the growth of a child. Children learn the environment in which they live using labels assigned as categories by their parents: Cats, dogs, birds.
After receiving a certain amount of labeled data, children start to recognize birds without the help of their parents and make some successful predictions. Supervised ML models are trained in a similar manner.
For example, high-performance healthcare computer vision systems are dependent on high-quality medical data annotation. Due to the poor quality of the labeled data, if a medical vision system makes a wrong analysis of an MRI report, the consequences can be dire.
You can also check our data-driven list of medical data annotation tools to find the option that best suits your business needs.
What are the categories of data labeling tools?
We can categorize data labeling tools into two main groups:
- Price-based categorization: Firms can develop their own software program for data labeling. There are also software services offered by third parties. It is possible to divide such tools into two categories: Open source and Closed source. Open-source tools are free, while proprietary tools have fees. Nonetheless, both strategies offer a more cost-effective alternative compared to developing your own enterprise data labeling software.
- Function-based categorization: It is important to determine the type of ML model you want to train for your business purposes in order to select the right data labeling tool. For example, if you are training a chatbot to increase customer service efficiency, a data labeling tool specialized in image annotation would not be useful. Consequently, training computer vision, NLP, and audio-based ML models require different data labeling tools.
Price based categorization
In-house
It is possible to create an in-house software program to ensure the efficiency of the data labeling process. However, this is a costly and slow process. Creating your own software requires effort, a highly skilled engineering team, and time.
Obviously, these are rare sources that are only available to a limited number of companies. The advantage of in-house data labeling tools is that they provide greater data security because the data is never sent outside the organization. Thus, it might be the best strategy for a company if it has highly personalized data.
Open-source
Open source data labeling platforms allow companies to customize existing data tagging solutions without having to develop software from scratch. They are completely free, and since the code is available to anyone, it can be modified to meet the needs of the business.
Closed source
Closed source data labeling software is another cost-friendly option compared to in-house. The difference between closed and open-source software is that you need to purchase a key license to use the service.
Even though there is an annual cost for the closed source data labeling software, the team behind the tool will help you set it up and use it for your business. They are also responsible for any necessary updates. Therefore, less IT staff is needed in your company than with open-source software.
Function-based categorization
Data labeling for computer vision training
Image annotation is the process behind the training of computer vision models. Annotated image data powers ML applications like self-driving cars, ML-guided disease detection, autonomous vehicles, and so on. There are tools that specialize in image annotation.
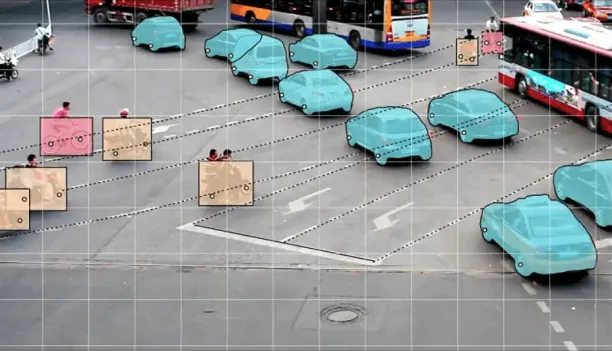
Data labeling for NLP training
Text annotation is the process behind training Natural Language Processing (NLP) models. NLP models help organizations derive the meanings behind text data and interpret it for their own benefit. There are tools that specialize in text annotation.
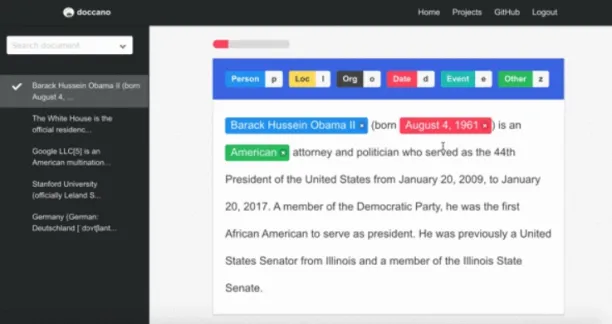
Data labeling for time series
Many ML models require proper annotation of time series data to function effectively. For example, sensors can be better trained if the conditions that force them to turn off are clearly annotated.
Data labeling for speech recognition
Audio annotation is the process underlying the training of speech reconstruction models. Speech recognition improves the customer service processes of companies. There are tools that specialize in annotating audio files.
You can check our sortable/filterable lists of data labeling/annotation/classification vendors and video annotation tools.
Data labeling tools vs. Data labeling service providers
Data labeling tools are software platforms or applications that enable businesses to manage and perform data labeling tasks in-house. These tools often come with features like task creation, workflow management, and automated annotations, allowing internal teams to label data such as images, text, and audio directly.
On the other hand, data labeling service providers offer outsourced solutions, where businesses partner with external companies to handle the entire data labeling process. These service providers use a combination of human labor and automated tools to label large datasets quickly and accurately. They typically offer expertise in specific data types and industries, making them an ideal choice for businesses looking for high-quality labeled data without investing in internal resources.
See the top data labeling service providers.
Further reading
To find vendors for data labeling, we can help:
Comments
Your email address will not be published. All fields are required.