Have you ever asked Siri to solve a math problem? Or asked about the weather from your Google assistant? Or have you used a sentence correction tool on your computer? If yes, then you have used natural language processing (NLP). NLP technology is increasingly being used to enable smart communication between people and their devices.
Data labeling is an integral part of training NLP models to mimic the human ability to understand and generate speech.
This article explores what data labeling is for natural language processing and how to approach it.
What is NLP data labeling?
Data labeling is to the process of using machine learning algorithms or other automated systems to assign labels or annotations to data (such as images, text, or audio) without human intervention or with minimal human involvement. The labeled data is then used to train the NLP models to make predictions or understand or generate speech.
How does it work?
Building a highly accurate NLP model requires a high volume of training data. This is because NLP models are based on machine learning (a subdomain of artificial intelligence (AI)) and since modern machine learning approaches like deep learning are data-hungry. To generate this large volume of training data, companies rely on:
- Machine learning models in cases where labeling can be automated
- Humans in cases where machine learning models do not have high confidence.
In this model, data that can not be auto-labeled with high confidence is sent by the ML model for human labeling. The data labeled by humans is then sent again to the ML model to retrain and improve it.
To learn more about how NLP data annotation works, check out this video:
You can also check our list of NLP services to find the option that best suits your project needs.
What are the types of data annotation in NLP?
Utterance annotation
In spoken language analysis, utterances are the smallest piece of speech. Anything that a user says that starts and ends with a pause are considered as an utterance.
For example:
“What is the time in Germany?”
“How is the weather in Paris?”
Since much of the language data is in long sentences, the data is split into individual utterances for training the ML model.
Intent annotation
This refers to what predictions are drawn by the ML from the said utterance by the user. For instance, in a chatbot, if the customer asks, “How much for the 2-inch tiles,” then the ML model will identify the intent as a “pricing query.”
There are also casual intents. For instance, a simple “yes” can also be “yeah, sure.” For more on intent classification, feel free to check our article on the topic.
Entity annotation
Entity annotation is one of the most important components of training an NLP training data set. It refers to identifying objects in an utterance. For example: “it is sunny in Milan” or “Bill Gates is the founder of Microsoft”. In these examples, Milan, Bill Gates, and Microsoft are all objects that need to be identified by the model. Check our article on named entity recognition for more on the topic.
What are the challenges in NLP data labeling?
Preparing data
Preparing data to train ML models is one of the most challenging tasks in creating NLP models. Since it requires high volumes of data of different types (audio, text, handwritten, etc.). However, it is much easier to gather and curate data today, thanks to advanced data mining.
Understanding homophones and synonyms
In text-to-speech annotation, some words have different meanings but similar pronunciations. It can be challenging to train the ML model on these words. Words with similar meanings might also be difficult to identify by the ML system.
Understanding emotions
Understanding the emotional condition of the speech can also be a challenging task. For instance, in a chatbot tool, it could be hard for the ML model to identify whether the speaker is angry, sad, or happy. Additionally, sarcasm or irony would also be difficult to recognize for the NLP model.
Industry-specific language
Vocabulary is different in every industry. Therefore, an NLP model trained with fashion brands’ data might not recognize terminologies used for the automotive sector.
To outsource or not to source
Outsourcing
Some companies can choose to outsource through crowd-sourced labeling services. With this option, you can have increased flexibility and quality of data labeling. However, outsourcing can be expensive and can also lead to data leaks.
In-house labeling
Some organizations hire internal labelers and tools for NLP data labeling. This can be a more secure option; however, it takes a dedicated workforce and tools to match the labeling capacity of a third-party service.
How to make the decision?
Answering the following question can give help you decide which option to choose:
- What level of expertise is required for your NLP labeling task?
- What level of data privacy do you require?
- What are the required labeling quality and capacity?
- Will NLP data labeling will be a core part of your business?
To make an objective decision on which option to choose, check out our sortable and filterable list of data annotation, labeling, and tagging services list, to compare and select the option that best suits your needs.
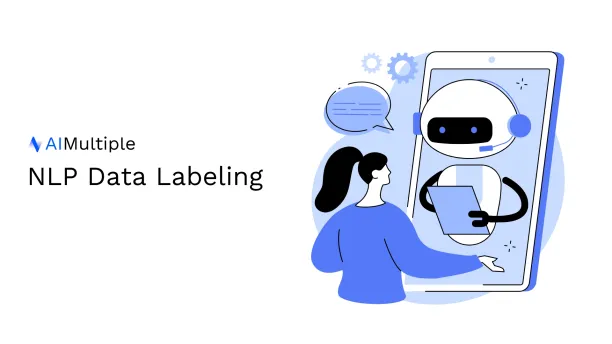
FAQ
How do I ensure the quality of labeled data?
Ensuring high-quality labeled data requires clear labeling guidelines, consistency, and regular quality checks. It’s important to have a well-defined process in place, such as cross-checking labels between multiple annotators, implementing review workflows, and using tools that provide feedback and validation. Additionally, using active learning to identify and label uncertain data can improve the accuracy and quality of the labeled dataset.
Comments
Your email address will not be published. All fields are required.