Healthcare Analytics Adoption Model: In-Depth Guide in 2024
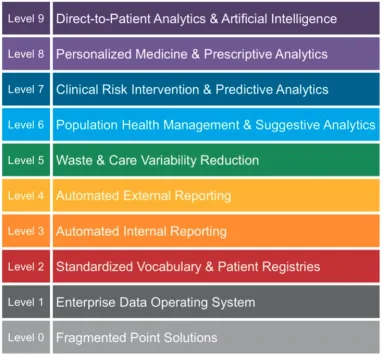
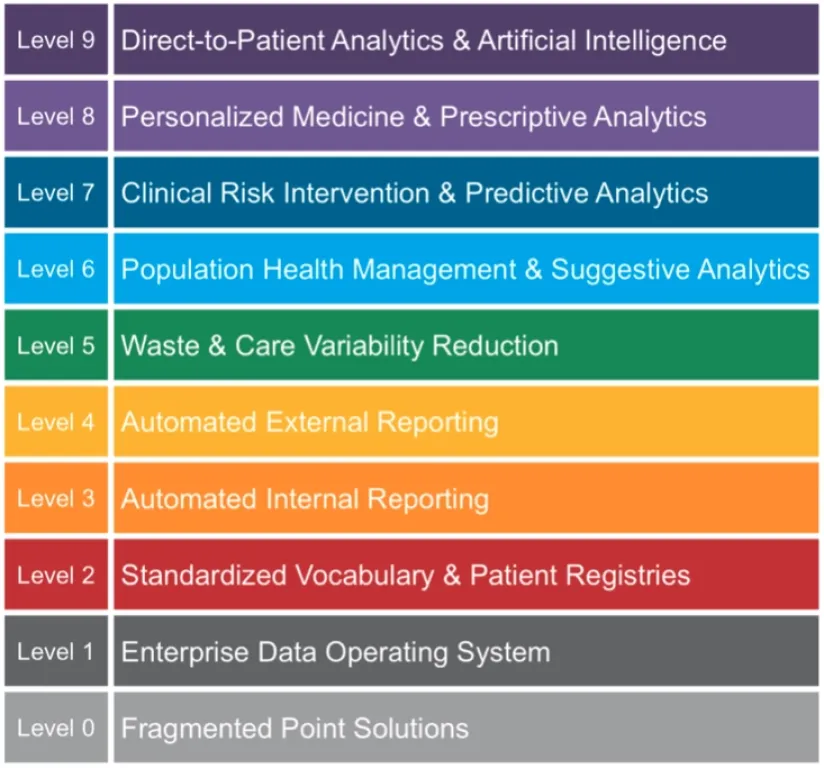
Analytics tools are used heavily today in healthcare organizations in financial, operational, and clinical functions. Healthcare adoption models are used as a guide to measure and advance predictive and prescriptive analytics capabilities of an organization. In 2002 the first analytics adoption model was released as a 5-step framework focusing on data management. However, the latest version was developed by Health Catalyst and Healthcare Information and Management Systems Society (HIMSS) and released in 2019 with more steps leveraging predictive and prescriptive analytics. HIMSS’ adoption model is specific to US healthcare providers (e.g. in areas such as external reporting), however it can be used as a general guide for non-US healthcare providers as well.
Benefits of leveraging the healthcare analytics adoption model
Using the healthcare analytics adoption model allows health organizations to:
- Evaluate vendor products
- Measure the organization’s success in adopting analytics
- Measure the healthcare industry’s adoption of analytics
- Progress in data-driven decision making
Stages of adoption
There are 3 main adoption stages of analytics strategy in the healthcare industry, which are data management, business intelligence, and analytics.
Data management
Data management is the basic step in healthcare analytics because it enables the higher stages of analytics, such as:
- Extracting insights from up-to-date datasets
- Integration of the data into the enterprise data warehouse
- Mining of clinical data (e.g. EMR and EHR)
- Predicting outcomes of diagnoses, diseases, and treatment plans.
The data management stage consists of 3 levels:
Level 0: Finding solutions for fragmentation
At this point, the available data is not co-located in a data warehouse nor is it architecturally integrated with one another. This typically causes:
- Overlapping content
- Difficulty in reporting
Level 1: Data warehousing
The Enterprise Data Warehouse (EDW) collects and stores raw data from all the different sources, enabling the next level.
Level 2: Standardization
The goal is to clean, validate, normalize, and standardize the EDW data. The data covers patient identity, physician identity, procedure codes, diagnosis codes, facility codes, department codes and others.
Business intelligence
The aim of implementing business intelligence in healthcare is to extract insight from the EDW data to:
- Improve patient care and safety
- Reduce costs and improve revenue by:
- Identifying highly profitable services
- Monitoring cashflow
- Optimizing pricing
- Streamlining claims and payment process
Business intelligence consists of 2 levels:
Level 3: Automating internal reporting
Level 4: Automating external reporting
External reports include reports to:
- Hospital Value-Based Purchasing (VBP)
- Joint Commission
- National Registry of Myocardial Infarction (NRMI)
- Physician Quality Reporting System (PQRS)
Analytics and AI
Applying analytics, AI, and machine learning algorithms on data as large as healthcare data enables identification of patterns and correlations, and provides sufficient insights for improving the delivery of healthcare. The analytics stage consists of 4 levels:
Level 5: Reducing waste and variability
This step is focused on measuring the organization’s compliance to medical regulations and best practices, minimizing waste, and reducing variation. Research suggests that 30% of healthcare expenses in the US is considered wasteful and caused by variations mostly reflected in overuse and underuse of drugs and medical procedures. Analytics from a standardized EDW, which associates clinical with cost data, enable improvements in individual patient care, as well as reduction in risks and costs.
Level 6: Suggestive analytics
For this purpose, the population health data of an organization should include bedside devices, home monitors, external pharmacy data, and detailed service-based costing. Implementing analytics on this data allows specialty departments (e.g. surgery or ER) to make data-driven decisions about resource allocation such as surgical suite slots.
Level 7: Predictive analytics
Predictive analytics is critical in all patient-care stages including diagnosis, prognosis, and treatment. It enables:
- early detection of in-patient deterioration
- identification of at-risk out-patients
- decreasing hospital readmissions
- reduction of medical equipment downtime
Level 8: Prescriptive analytics
Prescriptive analytics enables personalized medicine. It enables:
- Genome sequencing
- Genomic data visualization and manipulation
- Interventional support modeling
Level 9: Leveraging artificial intelligence
AI-enabled analytics are provided directly to patients to support a collaboration between the physician and the patient. Treatment and health maintenance protocols utilize AI-based digital twins– “Patients Like This” and “Patients Like Me” pattern recognition.
You can also our list of AI and healthcare services:
For more on healthcare analytics
To learn more, feel free to read our articles:
To learn more about AI, feel free to read our whitepaper on the topic
And if you feel like you can benefit from investing in a healthcare analytics solution, let us guide you through the process

Cem has been the principal analyst at AIMultiple since 2017. AIMultiple informs hundreds of thousands of businesses (as per similarWeb) including 60% of Fortune 500 every month.
Cem's work has been cited by leading global publications including Business Insider, Forbes, Washington Post, global firms like Deloitte, HPE, NGOs like World Economic Forum and supranational organizations like European Commission. You can see more reputable companies and media that referenced AIMultiple.
Throughout his career, Cem served as a tech consultant, tech buyer and tech entrepreneur. He advised businesses on their enterprise software, automation, cloud, AI / ML and other technology related decisions at McKinsey & Company and Altman Solon for more than a decade. He also published a McKinsey report on digitalization.
He led technology strategy and procurement of a telco while reporting to the CEO. He has also led commercial growth of deep tech company Hypatos that reached a 7 digit annual recurring revenue and a 9 digit valuation from 0 within 2 years. Cem's work in Hypatos was covered by leading technology publications like TechCrunch and Business Insider.
Cem regularly speaks at international technology conferences. He graduated from Bogazici University as a computer engineer and holds an MBA from Columbia Business School.
To stay up-to-date on B2B tech & accelerate your enterprise:
Follow on
Comments
Your email address will not be published. All fields are required.