Generative Design: Definition, Use Cases & Challenges in 2024
Design professionals across various sectors constantly seek tools to optimize creativity and innovation, aiming to predict and cater to evolving market demands. Traditional design methods, though effective, are often time-consuming and bound by human limitations, potentially missing the vast array of possibilities in complex design scenarios.
Generative AI, merging the worlds of design and artificial intelligence (AI), offers an ingenious solution. By algorithmically generating countless design variations and optimizing based on set parameters, it unlocks previously unimagined design potentials.
In this article, we will explain the importance of generative AI use cases and challenges for generative design.
What is generative design?
Generative design is an advanced process in which generative design software, often powered by artificial intelligence, produces multiple design alternatives based on specific design parameters provided by users. Instead of focusing on a single solution as seen in traditional methods, the generative design process uses computer aided design (CAD) tools to explore a vast design space to identify a multitude of viable design solutions that satisfy the given design intent.
According to McKinsey, in various industries, generative algorithms have cut the cost of components by 6-20%, decreased their weight by 10-50%, and shortened the development timeline by 30-50%.1 You can see the effectiveness of generative design in the figure below.
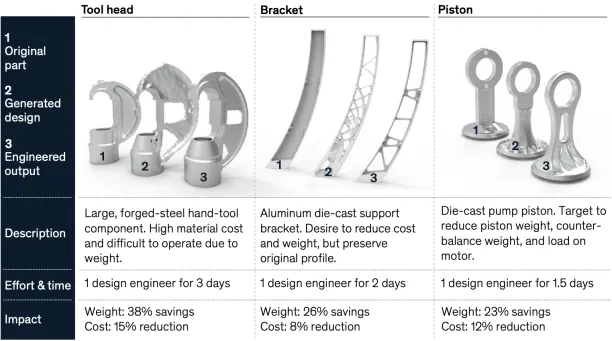
Source: McKinsey & Company
Key individual components of generative design are:
Design parameters
These are the guiding principles, such as geometric dimensions or material constraints, that frame the scope of the design. They play a crucial role in shaping the solutions that the software proposes.
Design and topology optimization
This is a mathematical method that modifies the material layout within a given design space. In the context of generative design, topology optimization refines designs, ensuring they meet performance criteria while using the least amount of material.
Design alternatives
Generative design software produces numerous potential solutions. Each of these alternatives is optimized for the design parameters and intent provided.
Further development
Once an initial set of designs is generated, there’s often a process of iterative refinement. Designs can be modified based on feedback, additional constraints, or new insights.
Additive manufacturing
Many of the design solutions generated may be complex or organic in shape. Additive manufacturing, or 3D printing, is often used to produce these intricate designs, as it allows for the creation of structures that would be difficult or impossible using traditional manufacturing methods.
Parametric design
Often integrated into the generative design process, parametric design allows for design solutions to be easily modified based on changes to input parameters. This means that if one parameter changes, the design can automatically adjust to accommodate.
Why is generative AI important for generative design?
Generative AI technology, often rooted in techniques such as Generative Adversarial Networks (GANs) and other machine learning models, is playing an increasingly significant role in the realm of generative design.
Here are some benefits of generative AI to generative design:
Complexity and scalability
Traditional generative design methods are algorithmically driven and often rely on deterministic processes. However, the design space can be vast and multi-dimensional. Generative AI can handle this complexity and scale, exploring numerous possibilities in a shorter time.
Data-driven insights
Generative AI can be trained on large datasets, drawing insights and patterns that might not be apparent to human designers. This can lead to the generation of design solutions that are informed by vast amounts of historical data, industry best practices, and even aesthetic trends.
Adaptive learning
Unlike static algorithms, generative AI models can learn and adapt. As more simulations and evaluations are done, the AI can refine its design approach, leading to better, more optimized solutions over time.
Novelty and creativity
Generative AI can produce unexpected and novel design outcomes that might not emerge from traditional algorithms. This can lead to groundbreaking and innovative designs, pushing the boundaries of what’s possible.
Customization and personalization
Generative AI can be tailored to produce design variations catering to specific user preferences or unique constraints. This is especially valuable in industries where personalization is a significant trend.
Efficiency and speed
Given the computational power of modern AI models, generative AI can significantly accelerate the design process and development time, especially when compared to manual or more traditional computer-aided methods.
What are the generative AI use cases in generative design?
Generative AI, with its capability to produce or generate new content from given data, can find various use cases in the realm of generative design across different industries. Here are some notable applications:
1- Architecture and urban planning
- Building design: Generative AI can be used to propose architectural forms and layouts based on environmental factors, site constraints, and aesthetic guidelines.
- Master planning: Algorithms can generate urban layouts, optimizing for traffic flow, pedestrian access, sunlight exposure, and more.
2- Product design and manufacturing
- Optimized components: AI can design parts that are lightweight yet durable, taking into account various constraints like materials, manufacturing processes, and performance requirements.
- Custom products: Generative AI can craft designs tailored to individual customer specifications or preferences, allowing for mass customization.
Figure 1. A chair designed by DALL-E with the prompt “design a realistic bohemian chair made from wood and loganberry cushion”
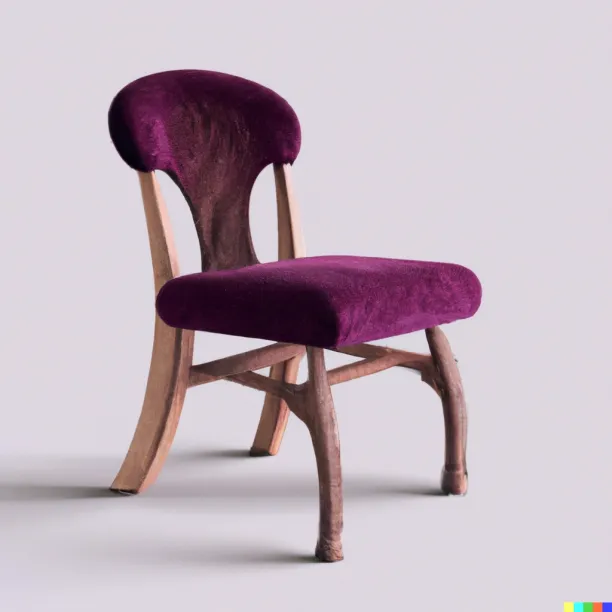
For more on such use cases, check our article on the use of generative AI in manufacturing.
3- Fashion and apparel
- Pattern generation: Algorithms can create new and unique patterns or textiles.
- Adaptive designs: AI can produce clothing designs based on specific criteria like fabric stretch, climate conditions, or even individual user measurements.
- Representative fashion models generation: Generative AI can also be used for designing various fashion models for all body types.
Japanese tech company DataGrid used GANS technology to create models that can change bodily. You can watch the video released by the company showing a multitude of generated models:
You can check our generative AI in fashion article to learn more.
4- Graphic design and art
- Visual content: Generative AI tools can create logos, banners, and other visual content based on specified themes or constraints.
- Art creation: There are AI systems that generate unique pieces of art, exploring styles, color palettes, and forms.
Figure 2. The cycleGAN algorithm is able to generate designs in the style of different artists and artistic genres, such as Monet, van Gogh, Cezanne and Ukiyo-e.2
5- Footwear design
AI algorithms can design shoes optimized for performance, comfort, and aesthetics, sometimes leading to structures or forms that might be unconventional yet functionally superior.
6- Automotive and aerospace
- Vehicle components: Generative AI can optimize designs for parts to be lightweight and aerodynamic while maintaining structural integrity.
- Cabin design: AI can help in designing vehicle or aircraft cabins to maximize space, comfort, and functionality.
7- Jewelry design
AI can be used to create intricate and novel jewelry designs, considering factors like material usage, aesthetics, and manufacturing feasibility.
8- Furniture design
Algorithms can generate furniture pieces that are ergonomic, functional, and aesthetically pleasing, taking into account material constraints and manufacturing processes.
9- Game development
- Environment generation: Generative AI can produce diverse and expansive virtual environments for video games.
- Character design: AI can assist in generating unique character designs based on specific criteria or themes.
10- Medical implants and prosthetics
AI can help design medical implants or prosthetic devices that are tailored to individual anatomies, ensuring better compatibility and performance.
What are the challenges of AI-generated design?
While the promise of generative AI in enhancing the generative design process is undeniable, there are challenges:
1- Over-reliance on technology: There’s a risk of sidelining the invaluable insights and creativity that human designers bring to the table in favor of the multitude of design alternatives generated by AI.
2- Interpretability: The reasoning behind AI-generated design solutions might not always be transparent. This makes it challenging to fully understand or justify certain design decisions compared to more traditional methods.
3- Data bias: The design solutions AI proposes are influenced by its training data. If this data is biased or lacks diversity, the AI-generated designs might inherit those biases, potentially compromising the design intent.
4- Integration challenges: Merging AI-powered generative design software with existing design and manufacturing workflows can be complex, requiring adaptation and training.
5- Ethical and IP concerns: AI-generated designs might inadvertently resemble existing designs, raising questions about originality and intellectual property rights. We have articles on the copyright problems and ethical problems of generative AI.
For more about these challenges, you can check our article on the risks of generative AI.
If you have questions or need help in finding vendors, we can help:
External Links
- 1. “How generative design could reshape the future of product development.” McKinsey. Accessed 28 August 2023.
- 2. Zhu, J.Y., Park, T., Isola, P., Efros, A.A.: Unpaired image-to-image translation using cycle-consistent adversarial networks. In ICCV 2017.

Cem has been the principal analyst at AIMultiple since 2017. AIMultiple informs hundreds of thousands of businesses (as per similarWeb) including 60% of Fortune 500 every month.
Cem's work has been cited by leading global publications including Business Insider, Forbes, Washington Post, global firms like Deloitte, HPE, NGOs like World Economic Forum and supranational organizations like European Commission. You can see more reputable companies and media that referenced AIMultiple.
Throughout his career, Cem served as a tech consultant, tech buyer and tech entrepreneur. He advised businesses on their enterprise software, automation, cloud, AI / ML and other technology related decisions at McKinsey & Company and Altman Solon for more than a decade. He also published a McKinsey report on digitalization.
He led technology strategy and procurement of a telco while reporting to the CEO. He has also led commercial growth of deep tech company Hypatos that reached a 7 digit annual recurring revenue and a 9 digit valuation from 0 within 2 years. Cem's work in Hypatos was covered by leading technology publications like TechCrunch and Business Insider.
Cem regularly speaks at international technology conferences. He graduated from Bogazici University as a computer engineer and holds an MBA from Columbia Business School.
To stay up-to-date on B2B tech & accelerate your enterprise:
Follow on
Comments
Your email address will not be published. All fields are required.