Foundation models like ChatGPT with many capabilities (e.g. translation, text generation) trained on public data have launched the generative AI wave.
As organizations increasingly adopt specialized AI, one of the most significant trends in AI is the shift toward integrating the latest AI model architectures, such as multimodal systems and sparse-expert networks, that offer domain-specific efficiency.
AIMultiple’s specialized AI framework splits specialized AI systems into 3 categories to facilitate comparing similar systems:
1. Horizontal AI
Horizontal AI refers to specialized AI systems that are focused on specific business functions (e.g. marketing, sales). They can be applicable across various industries. Unlike vertical AI, Horizontal AI models are more generalized and can be adapted for multiple use cases. The key characteristics of horizontal AI include:
- Cross-industry applications: Horizontal AI models are versatile and can be implemented in diverse industries.
- BU-specific applications: Some horizontal solutions are focused on a specific business unit such as marketing, customer service, or HR.
- General-purpose tools: These specialized AI systems are built to perform common tasks like natural language processing (NLP), computer vision, or predictive analytics, which are useful in multiple contexts.
Real-life examples for Horizontal AI
1. Chatbots and Virtual Assistants
AI-powered chatbots and virtual assistants help businesses automate customer support, answer frequently asked questions, and improve user engagement.
Zendesk Answer Bot is an example, used in customer service to provide instant responses to common inquiries and reduce the workload on human agents.
2. AI-Powered Cybersecurity
AI enhances cybersecurity by identifying threats, monitoring network traffic, and preventing attacks in real-time. Microsoft Sentinel AI delivers enterprise-level security analytics by leveraging machine learning to identify security incidents across multiple IT systems.
CrowdStrike Falcon is another cybersecurity AI application that protects endpoints from malware and unauthorized access through real-time AI-driven threat intelligence.
3. Business Intelligence and Data Analytics
Companies use AI-driven analytics tools to make data-driven decisions, optimize operations, and improve forecasting.
Tableau AI and Microsoft Power BI utilize AI to analyze large datasets, generate actionable insights, and deliver visual reports to businesses across various industries. Google Analytics applies AI to track user behavior on websites, helping digital marketers optimize their content and advertising strategies.
4. AI for Marketing and Sales
AI models are used in content recommendation, customer segmentation, and interaction analysis. Salesforce Einstein AI applies machine learning techniques to analyze business interactions and suggest relevant actions. Hootsuite AI examines engagement patterns on social media platforms. Peasy.ai and HubSpot AI process customer data to provide insights related to audience behavior.
5. AI for Automation and Process Optimization
AI-driven automation systems help automate repetitive tasks and streamline workflows. UiPath and Automation Anywhere provide AI-powered RPA solutions that automate data entry, invoice processing, and other business workflows.
6. Computer Vision Applications
AI-powered computer vision systems analyze and process images and videos for various applications. Google Vision AI detects objects, text, and faces in images, making it useful for security, retail, and healthcare applications. Amazon Rekognition is used for face recognition, identity verification, and video analysis.
2. Vertical AI
Vertical AI refers to AI systems specifically designed to solve problems within a particular industry or domain. These AI models are highly specialized, focusing on niche areas such as healthcare, finance, manufacturing, or legal services. The key characteristics of Vertical AI include:
- Industry-Specific Expertise: Vertical AI models are tailored to understand and address the unique challenges, regulations, and workflows of a particular industry.
- Customized Data: These models are trained on highly specific industry data, resulting in more accurate and relevant outcomes.
- Specialized Applications: Examples of Vertical AI include specialized AI systems used for medical diagnosis, financial risk assessment, supply chain optimization, and legal document analysis.
Real-life examples for Vertical AI
1. Healthcare AI
IBM Watson Health utilizes AI to analyze medical records, aid in disease diagnosis, and recommend treatment plans. It is tailored specifically for the healthcare industry, leveraging medical data, clinical trials, and research to provide insights to doctors and healthcare providers.
Watson has been used in oncology to help oncologists determine personalized treatment options for cancer patients, considering vast amounts of data that a human might not easily process1 .
2. Finance and Banking AI
AI is used in finance for risk assessment, fraud detection, and investment analysis. BloombergGPT is an AI model trained on financial data to support market analysis. Kensho (S&P Global) processes economic data and financial reports.
Investment firms use AlphaSense to track market trends, company performance, and emerging risks, helping them make informed decisions based on vast amounts of financial data2 .
3. Cybersecurity AI
AI-driven security models help detect threats, prevent cyberattacks, and monitor networks. Microsoft Sentinel AI provides automated threat detection and response. CrowdStrike Falcon monitors and protects endpoints against cyber threats. FireEye Helix uses AI for vulnerability assessment and security event analysis.
4. Legal AI
AI supports legal professionals by automating research, contract analysis, and litigation prediction. ROSS Intelligence processes legal texts to assist with case law research. Lex Machina analyzes past court cases to identify trends and predict outcomes. Casetext automates contract review and legal document analysis. These AI models help legal professionals improve efficiency in research and case preparation.
5. AI in Transportation and Logistics
AI models improve route planning, supply chain management, and autonomous systems. Tesla’s Autopilot is a specialized AI model designed for autonomous driving. It processes data from cameras, radar, and ultrasonic sensors to navigate roads, avoid obstacles, and assist with driving tasks.
Tesla vehicles equipped with Autopilot can perform tasks such as lane keeping, adaptive cruise control, and automated parking, making driving safer and more efficient3 .
6. AI in Agriculture
AI in agriculture helps with precision farming, pest detection, and yield prediction. Blue River Technology, a subsidiary of John Deere, uses AI for precision agriculture. Its See & Spray technology identifies crops and weeds in real-time and applies herbicides only where necessary.
Farmers using Blue River’s AI-driven equipment can significantly reduce the amount of chemicals used, lowering costs and environmental impact while maintaining crop yields4 .
7. Pharma AI
DeepMind’s AlphaFold is an AI model developed by DeepMind to predict protein structures. This model is highly specialized within the field of molecular biology and has revolutionized research in this area.
AlphaFold has been used to predict the structures of proteins that are difficult to study experimentally, accelerating drug discovery and understanding of diseases.
3. Common AI
Common AI refers to artificial intelligence models and systems that are widely used across different domains and everyday applications. These AI systems perform tasks such as text generation, image processing, voice recognition, and recommendation systems. Unlike vertical AI, which is industry-specific, standard AI solutions are designed for broad usage across multiple fields.
Real-life examples for Common AI
Most LLMs fall under this bucket.
GPT-4 by OpenAI
- Use Case: GPT-4 is a general-purpose language model that can be used across various industries for tasks like content generation, customer support, coding assistance, data analysis, and more.
- Real-Life Application: Companies across different sectors, from tech firms to media outlets, use GPT-4 for generating blog posts, automating customer service responses, drafting emails, and even creating code snippets.
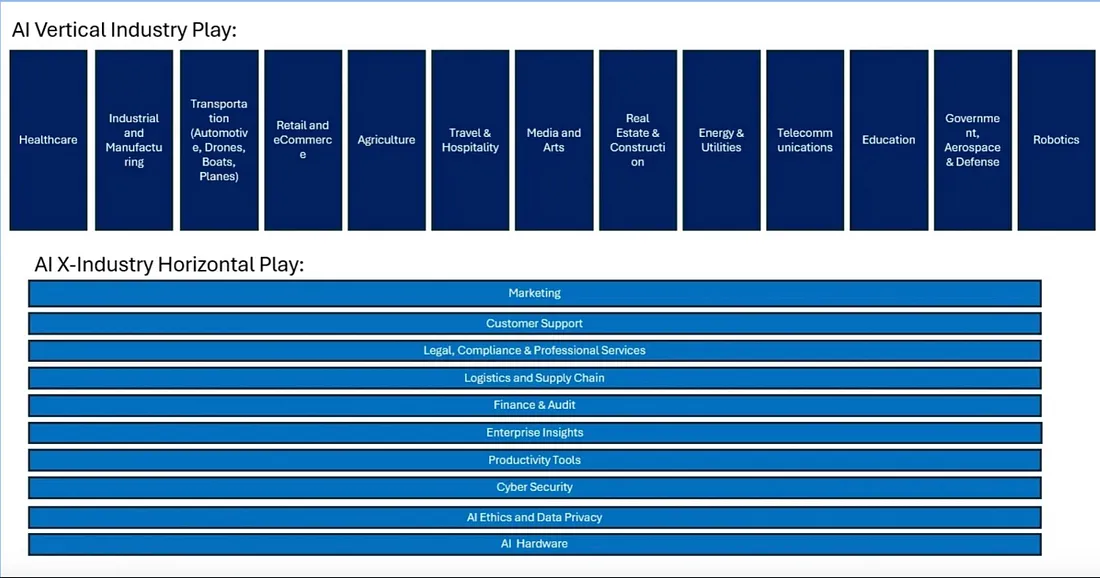
Google Cloud AI
- Use Case: Google Cloud AI offers tools and APIs for natural language processing, vision, translation, and more. These tools are applicable across numerous industries such as retail, healthcare, finance, and manufacturing.
- Real-Life Application: Retailers use Google Cloud’s Vision AI to automate inventory management by recognizing products and tracking stock levels through images and videos.
- Microsoft Azure AI
- Use Case: Azure AI provides a range of services, including machine learning, cognitive services, and AI development tools that can be implemented across various industries.
- Real-Life Application: Businesses use Azure AI for tasks such as sentiment analysis in customer feedback, predictive maintenance in manufacturing, and fraud detection in banking.
Other specialized AI models
Above, we split AI models by their application areas in business. There are other ways to categorize AI models further. These specialized AI models that cater to more focused, cross-disciplinary needs or unique environments:
- Edge AI: AI models optimized to run on edge devices (like IoT sensors or smartphones) rather than centralized data centers. Edge AI is crucial for real-time processing in applications such as autonomous vehicles, smart cities, and industrial automation.
- Multimodal AI: Multimodal AI models, such as large multimodal models, can process and integrate data from multiple modalities such as text, images, audio, and video, providing more comprehensive and context-rich outputs.
- Generative AI: Generative AI models can create new content such as text, images, music, and even video by learning from existing data. These models include technologies like Generative Adversarial Networks (GANs) and variational autoencoders.
- Explainable AI (XAI): AI systems designed to provide transparent and understandable decision-making processes. This is increasingly important in sectors where AI decisions must be audited or justified, such as healthcare, finance, and law.
FAQ
How can you find vertical AI / horizontal AI solutions?
Vertical AI can be found in the form of vertical SaaS (vSaaS). Users can search industry analysts like AIMultiple to find new solutions. For example, AIMultiple’s AP AI list contains horizontal AI vendors in the accounts payable (AP) domain.
What is the future of specialized AI technologies?
Both established SaaS companies and AI startups are launching new specialized AI solutions. As these solutions mature, AIMultiple expects vendors to consolidate their offerings to help enterprises:
Minimize application switching for employees
vendor sprawl
However, given that specialized AI is an emerging field, we don’t expect this consolidation in the next few years.
How can you find vertical AI / horizontal AI solutions
For Vertical AI solutions, you can start by researching AI vendors or platforms that specialize in your industry. These solutions are often tailored to address the unique challenges and regulatory requirements of a particular sector, such as healthcare, finance, or manufacturing. Industry conferences, trade publications, and professional networks can also provide insights into leading Vertical AI providers. On the other hand, Horizontal AI solutions are more generalized and can be applied across multiple industries. These solutions are typically offered by major tech companies or AI platforms, such as cloud service providers (e.g., AWS, Google Cloud, Microsoft Azure), which provide AI tools for a wide range of applications, including natural language processing, predictive analytics, and computer vision. Exploring these platforms, reviewing case studies, and consulting with specialized AI consultants can help you identify the right Horizontal AI tools that can be adapted to your specific business needs. Additionally, attending AI-focused conferences or webinars can expose you to both Vertical and Horizontal AI solutions and how they are being used effectively across different domains.
Why is specialized AI relevant now?
From an inference (i.e. running a machine learning model to produce predictions) perspective, we have hit the limits of increasing the scale of dense transformer models. A state-of-the-art 8xGPU cluster can not serve a multi-trillion parameter dense transformer model at a fast enough speed to keep a human reader engaged.
This is why OpenAI relied on a Mixture of Experts architecture in GPT-4.5 . Such architectures enable building smaller expert machine learning models that act together to solve a diverse set of problems.
Since our capabilities in building larger deep learning models may grow slowly, we need to increase the specialization of these models to increase performance. There has been numerous examples of specialized data improving model performance.6
Notes & external links
All models mentioned in this article are narrow AI models and not generalized artificial intelligence models. Generalized AI is not likely to happen in this decade.
External Links
- 1. IBM Products.
- 2. AlphaSense | Market Intelligence and Search Platform. AlphaSense
- 3. Autopilot | Tesla.
- 4. Welcome - Welcome | Blue River Technology .
- 5. GPT-4 Architecture, Infrastructure, Training Dataset, Costs, Vision, MoE – SemiAnalysis. SemiAnalysis
- 6. Introducing BloombergGPT, Bloomberg’s 50-billion parameter large language model, purpose-built from scratch for finance | Press | Bloomberg LP.
Comments
Your email address will not be published. All fields are required.