Exploring Analytics & AI in 2024: A Detailed Primer
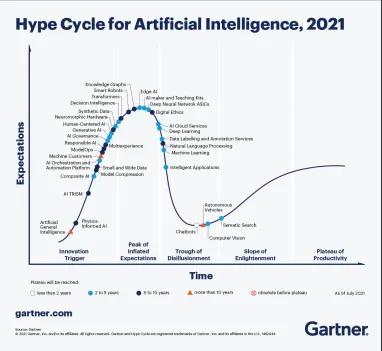
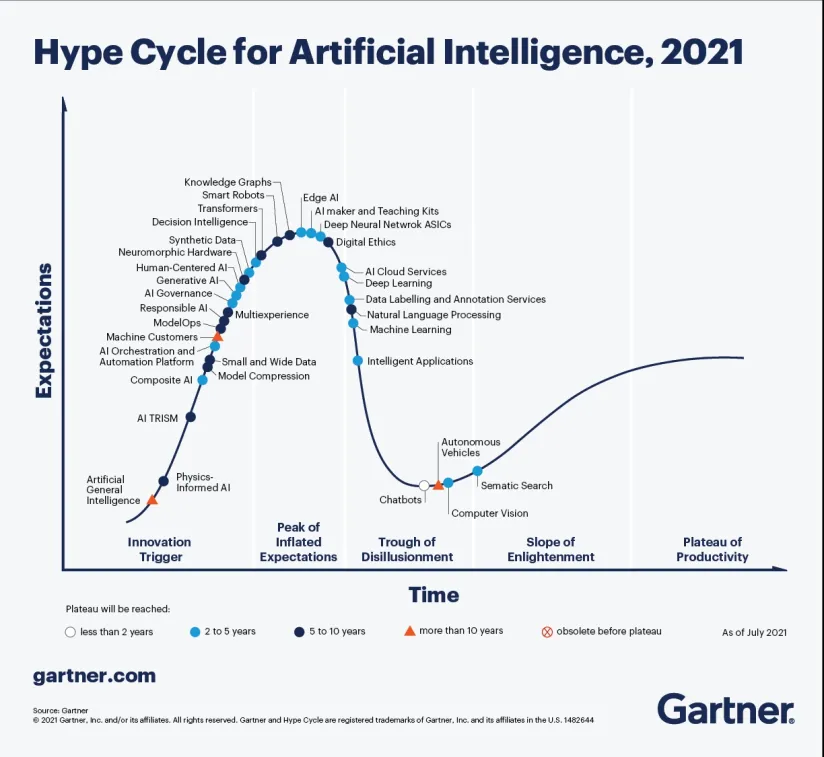
It is often said that data is the most valuable asset a business can have; the oil of a digital era. But data itself, while interesting, often leaves out a variety of important details – creating a need for analytics. And how we complete these analytics has evolved – and will continue to do so; particularly with the rapid proliferation of AI tools and technologies.
What is analytics? [Separate article]
What are the benefits of analytics?
Data analytics allows companies to institute data-driven decision making which allows companies to minimize bias and gut feel while making both important corporate decisions and making fast operational decisions. For example; with Data Analytics, a business can classify customers according to patterns found in data to understand their customers’ perspective.
Additionally, a successful analytics program can lead to:
- Increased productivity via instant access to the right data through better classification processes
- Improved speed, accuracy, and efficiency throughout analytics and data management practices
- Facilitating clear compliance with legal and similar requirements
- Cost saving by finding the best ways to achieve a wide range of tasks
To better understand data, analytics, AI, and how they all go together, we will answer the following questions:
Why is analytics important now?
The belief that data can serve as a guide is growing stronger in both business and government. Organizations want to act on the growing data sets they are gathering, which leads them to place analytics at the center of the decision-making process.
The graph below from Dresner Consulting Services reveals how organizations are showing increased interest in big data. As seen on the graph, the percentage of organizations that use data analytics has increased from 16% to 59% between 2015 and 2018. So organizations need to understand that if they are not using analytics to take advantage of data-driven decision-making, their competitors probably are or will within a few years.
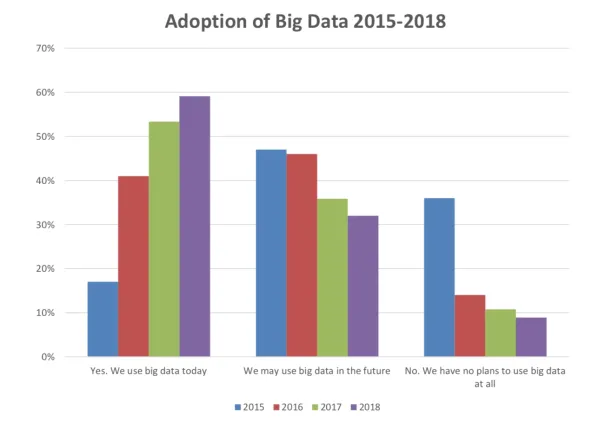
How did analytics evolve?
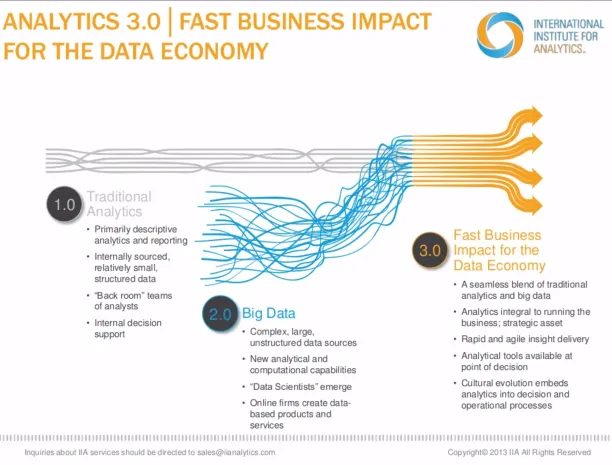
Analytics 0.1
Since the early days of computing, companies relied on computing systems such as IBM mainframes and later spreadsheet programs like Microsoft Excel to analyze data. However, these analyses were mostly performed ad-hoc and lacked the replicability and robustness of later approaches.
Analytics 1.0
The evolution of Data Analytics began in the mid-90s. Organizations started using Data Warehouse (DW) and Operational Data Store (ODS) technologies to collect and store business data. Business Intelligence (BI) applications were developed to help businesses get insights from structured data like sales data, customer interactions, and financial reports to obtain a competitive advantage. Due to the manual workload of data preparation at the time, the time remaining for analytics was comparatively low.
Analytics 2.0
In the early 2000s, the volume of data generated from individuals exploded as the internet became a mainstream product. This is called the era of Big Data because of the amount of data provided from phones and social media platforms. Big Data technologies and tools enlarged the set of capabilities that data analytics can do. Some of these capabilities are processing data and leveraging with code in open-source software like Hadoop.
Analytics 3.0
With further increases in data generated and increased expectations to make real-time decisions, decision-making needs to be pushed to edge devices. Analytics 3.0 combines the information generated at the edge with internal data of the organization using the latest machine learning approaches to predict potential outcomes.
Shankar Meganatha, Oracle Enterprise Cloud Architect, has a simple explanation for Analytics 3.0:
Every device, shipment, and consumer leaves a trail referred to as data exhaust. It’s just not feasible to keep moving very large amounts of raw data to centralized data stores – it is too big, changing too fast, and hyper distributed. Analytics 3.0 is essentially a combination of traditional business intelligence, big data and Internet of Things (IoT) distributed throughout the network.
What are the trends shaping analytics today?
Gartner’s hype cycle shared at the top of the article and our interviews highlighted these trends:
AI
AI is helping analytics get automated, more accessible, and more powerful. We have explored this in detail in our AI in analytics article.
Analytics Enablers
Natural language user interfaces (NLUI): All employees need to be able to at least access analytics. These interfaces enable employees to write their queries in natural language and access results,
Application specific analytics
Text analytics: Analyzing text requires the use of Natural Language Processing (NLP), a branch of AI. There are emerging specialized analytics tools for analyzing written communication.
What are the best practices for analytics?
Based on analytics vendors and our own experience and interviews, these are emerging best practices:
High quality data collection is an important first step
Most organizations still throw away a significant share of their data or keep data with significant quality issues. With data issues, analytics can be an exercise of garbage in, and garbage out.
Choose the Best Visualization Tools
To see the big picture in your data, it is good to use the most suitable visualization tools. Visualization is a great way to understand and present insights in the process of decision-making.
Identify your KPIs and regularly monitor them via dashboards
Identifying and tracking KPIs is critical for any business. To achieve this, it is good to keep in mind the end goal of the collected data. With that answer, you can add relevant parameters to your reports or dashboards, and remove the redundant ones. Well-prepared reports and dashboards, which are free from irrelevant metrics, ease organizations’ understanding of the current situation and improvement areas.
Check your Analysis Periodically
Results of your analysis will change as your company and market conditions evolve. A decision your organization makes today may not be the most optimal decision tomorrow. That’s why periodical analytical checks of the insights can be a life-saving action.
What are the analytics pitfalls to avoid?
We have heard about these analytics pitfalls as we spoke to practitioners:
- Organizations that operate on executives’ gut feel rather than data. The first step in setting up analytics in an organization should be to ensure that the whole company, from management to most junior employees, follows data-driven decision-making.
- Operating a small sample size of data, which is associated with low statistical power, prevents organizations from success. Make sure you have a large volume of data to get accurate insights.
- Data may give you all the information you need, but analysts should be careful while translating it into insight. Confusing causation with correlation may lead to misleading conclusions, be hesitant to make conclusions, and try to find out an answer to the question of why.
What are some modern analytics tools?
Some tools that can enable more powerful analytics include:
Name | Founded | Status | Number of Employees |
---|---|---|---|
Amazon Machine Learning | 1994 | Public | 10,001+ |
Ayasdi | 2008 | Private | 51-200 |
BigML | 2011 | Private | 11-50 |
H2O.ai H2O | 2012 | Private | 51-200 |
IBM Watson | 1911 | Public | 10,001+ |
Infosys | 1981 | Public | 10,001+ |
Microsoft Azure Machine Learning | 1975 | Public | 10,001+ |
Receptiviti | 2015 | Private | 11-50 |
SAS Analytics Suite | 1976 | Public | 10,001+ |

Cem has been the principal analyst at AIMultiple since 2017. AIMultiple informs hundreds of thousands of businesses (as per similarWeb) including 60% of Fortune 500 every month.
Cem's work has been cited by leading global publications including Business Insider, Forbes, Washington Post, global firms like Deloitte, HPE, NGOs like World Economic Forum and supranational organizations like European Commission. You can see more reputable companies and media that referenced AIMultiple.
Throughout his career, Cem served as a tech consultant, tech buyer and tech entrepreneur. He advised businesses on their enterprise software, automation, cloud, AI / ML and other technology related decisions at McKinsey & Company and Altman Solon for more than a decade. He also published a McKinsey report on digitalization.
He led technology strategy and procurement of a telco while reporting to the CEO. He has also led commercial growth of deep tech company Hypatos that reached a 7 digit annual recurring revenue and a 9 digit valuation from 0 within 2 years. Cem's work in Hypatos was covered by leading technology publications like TechCrunch and Business Insider.
Cem regularly speaks at international technology conferences. He graduated from Bogazici University as a computer engineer and holds an MBA from Columbia Business School.
To stay up-to-date on B2B tech & accelerate your enterprise:
Follow on
Comments
Your email address will not be published. All fields are required.