Top 4 Use Cases of Generative AI in Banking [2024]
Gartner identified generative AI as a top technology trend in the last years for the banking and investment industry.1 Generative AI’s contributions to areas such as data privacy, fraud detection, risk management can be critical to financial services companies.
This article explains the top 4 use cases of generative AI in banking, with some real-life examples.
Fraud Detection
Detecting anomalous and fraudulent transactions is one of the applications of generative AI in the banking industry. In a study, researchers constructed a training set of fraudulent transactions, and then utilized it in a Generative Adversarial Network (GAN) -a generative model based on deep neural networks- to produce synthetic fraudulent transactions.2 The synthetic data was then compared to the genuine data to see whether the GAN was capable of developing sensitivity to identify anomalous transactions. Finally, it is seen that using a GAN-enhanced training set to detect such transactions outperforms that of the unprocessed original data set.
As a result of this study, it appeared that training GANs for the purpose of fraud detection produced successful outcomes because of developing sensitivity after being trained to identify underrepresented transactions. This is an especially important application for financial services providers that deal with enormous number of transactions.
Case Study for Fraud Detection via Generative AI in Banking
Swedbank used GANs to detect fraudulent transactions.3 GANs are trained to learn legal and illegal transactions in order to detect the fraudulent ones by creating graphs that reveal their patterns.
However, employing GANs for fraud detection has the potential to generate inaccurate results (see Figure 1), necessitating additional improvement.
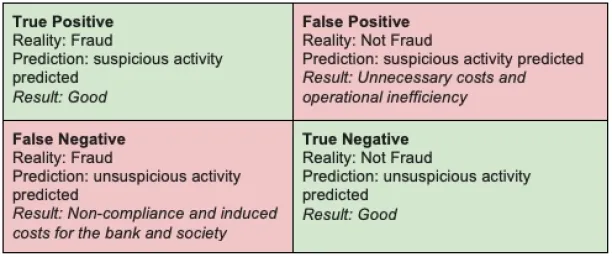
(Figure 1. Source: “Challenges in modeling fraud as a binary classification problem”)
Data Privacy
The use of synthetic data has the potential to overcome the challenges that the banking industry is facing, particularly in the context of data privacy. Synthetic data can be used to create shareable data in place of customer data that cannot be shared due to privacy concerns and data protection laws. Further, synthetic customer data are ideal for training ML models to assist banks determine whether a customer is eligible for a credit or mortgage loan, and how much can be offered.
GANs are capable of producing synthetic data (see Figure 2) and thus appropriate for the needs of the banking industry. Synthetic data generation can be achieved by different versions of GAN such as Conditional GAN, WGAN, Deep Regret Analytic GAN, or TimeGAN.
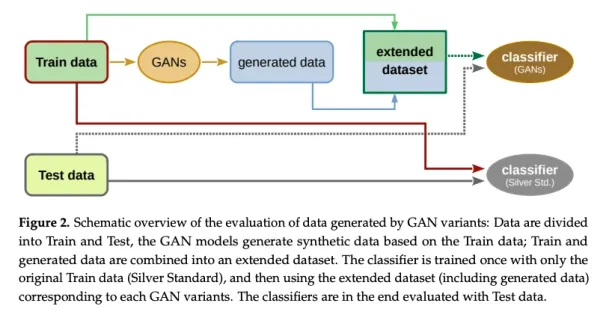
(Figure 2. Source: “GAN-Based Approaches for Generating Structured Data in the Medical Domain”)
Risk Management
Establishing a risk management plan is essential for banks to maintain an appropriate level of risk exposure, identify possible risk areas, and take action to preserve profitability. Banks may suffer losses if liquidity, credit, operational, and other risks are not appropriately handled.
Besides certain software systems for risk minimization, the use of generative AI is one possible solution for minimizing such losses resulting from the lack of adequate risk management.
With GANs, it is possible to calculate value-at-risk estimations that show the potential amount of loss in a particular period of time or to create economic scenarios that are useful for predicting the future of financial markets.4 5 GANs facilitates comprehending the possibilities of volatility by generating novel and assumption-free scenarios (See Figure 3) based on historical data patterns.
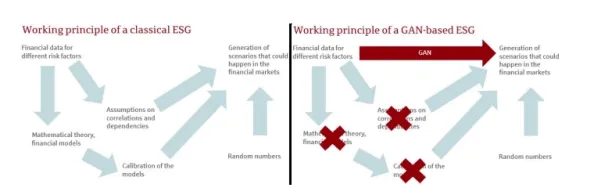
(Figure 3. Source: “Scenario generation for market risk models using generative neural networks”)
Generating Applicant-Friendly Denial Explanations
Loan decisions are one of the uses of AI in the banking sector. AI enables banks to determine whether a customer is qualified for credit lending by risk scoring, as well as make informed decisions about the maximum credit limit that a customer can have, and loan pricing based on the assessed risks.6
Nevertheless, not only decision makers, but also loan applicants require explanations of AI-based decision-making processes, such as the reason why their applications were denied. The reason for such a need is to ensure user trust as well as to increase customer awareness so that they can make more informed applications in the future.
In this context, a conditional GAN –a variant of GAN in which the generator and discriminator are conditioned through class labels– is useful to generate applicant-friendly denial explanations (See Figure 4). Organizing the causes of denial from simple to complex in a hierarchical manner, two-level conditioning is created for generating understandable explanations.
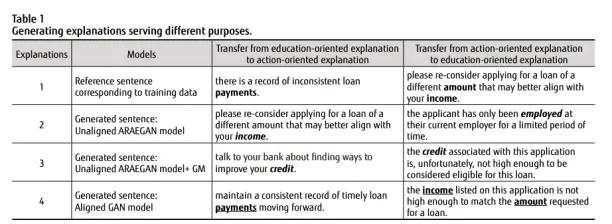
(Figure 4. Source: “Generating User-Friendly Explanations for Loan Denials Using Generative Adversarial Networks”)
For all GenAI applications in financial services, not just in banking, read our article on generative AI in financial services.
For more information on other financial technology capabilities of AI, feel free to read our articles:
KYC Automation: 5 Technologies That Increase Transparency
Anti Money Laundering Algorithms in 2022: Tackling AML with AI
If you have questions or need help in finding vendors, reach out:
External Links
- 1. “Gartner Identifies Three Technology Trends Gaining Traction in Banking and Investment Services in 2022” (Press release). Stamford, Connecticut: Gartner. May 24, 2022. Retrieved November 11, 2022.
- 2. “Fiore U.; De Santis A.; Perla F.; Zanetti P.; Palmieri F. (2019). “Using generative adversarial networks for improving classification effectiveness in credit card fraud detection”. Information Sciences. Volume 479: 448-455.
- 3. “Mamaghani M.; Ghorbani N.; Dowling J.; Bzhalava D.; Ramamoorthy P.; Bennett M. (March 26, 2022). “Detecting Financial Fraud Using GANs at Swedbank with Hopsworks and NVIDIA GPUs”. NVIDIA Developer. Retrieved November 11, 2022.
- 4. “Fiechtner L. (2019). “Risk Management with Generative Adversarial Networks”. [MSc Dissertion, University of Oxford]
- 5. “Flaig S.; Junike G. (2021). “Scenario generation for market risk models using generative neural networks”. Risks. Vol. 10(11): 1-28.
- 6. “AI-powered decision making for the bank of the future” (PDF). McKinsey & Company. March 2021. Retrieved November 11, 2022.

Cem has been the principal analyst at AIMultiple since 2017. AIMultiple informs hundreds of thousands of businesses (as per similarWeb) including 60% of Fortune 500 every month.
Cem's work has been cited by leading global publications including Business Insider, Forbes, Washington Post, global firms like Deloitte, HPE, NGOs like World Economic Forum and supranational organizations like European Commission. You can see more reputable companies and media that referenced AIMultiple.
Throughout his career, Cem served as a tech consultant, tech buyer and tech entrepreneur. He advised businesses on their enterprise software, automation, cloud, AI / ML and other technology related decisions at McKinsey & Company and Altman Solon for more than a decade. He also published a McKinsey report on digitalization.
He led technology strategy and procurement of a telco while reporting to the CEO. He has also led commercial growth of deep tech company Hypatos that reached a 7 digit annual recurring revenue and a 9 digit valuation from 0 within 2 years. Cem's work in Hypatos was covered by leading technology publications like TechCrunch and Business Insider.
Cem regularly speaks at international technology conferences. He graduated from Bogazici University as a computer engineer and holds an MBA from Columbia Business School.
To stay up-to-date on B2B tech & accelerate your enterprise:
Follow on
Comments
Your email address will not be published. All fields are required.