Synthetic Data for Healthcare: Benefits & Case Studies in 2024
From robot-assisted surgeries to medical imaging techniques, artificial intelligence applications in healthcare are rapidly changing the healthcare industry and providing improvements in cost and quality of service. For example, Accenture states that AI clinical health applications can create $150 billion annual savings for the US healthcare industry by 2026.
However, data privacy concerns limit the extent of innovation in the healthcare industry. Patient medical data contains highly sensitive and personally identifiable data types such as:
- Full medical histories
- Ongoing conditions
- Social security numbers
- Payment and credit card information
This is why regulations such as HIPAA heavily protect patient medical records. Nevertheless, HIPAA Journal reports that more than 40 million healthcare records have been exposed or disclosed without permission between July 2020 to June 2021 in the US. Hacking and unauthorized disclosures by malicious insiders are two of the most common causes of data breaches in the healthcare industry.
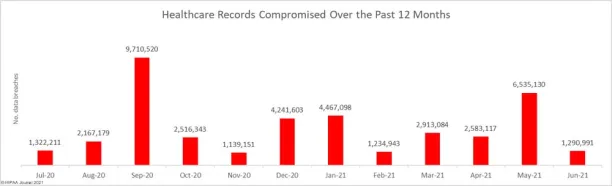
Accenture’s survey reports that one in five healthcare employees would be willing to sell patient data to unauthorized parties for as little as $500. Therefore, data privacy seems to be the biggest roadblock on the path to innovation and more advanced AI applications in healthcare. Synthetic (i.e. artificially generated) patient data can be the solution to the challenges of healthcare innovation.
How can synthetic data help the healthcare industry?
Sharing healthcare data among researchers, institutions, and companies building AI solutions can have numerous benefits. However, sharing patient data safely is a serious challenge in the healthcare industry because of regulations such as HIPAA. Synthetic data can help healthcare researchers create shareable data and overcome these challenges.
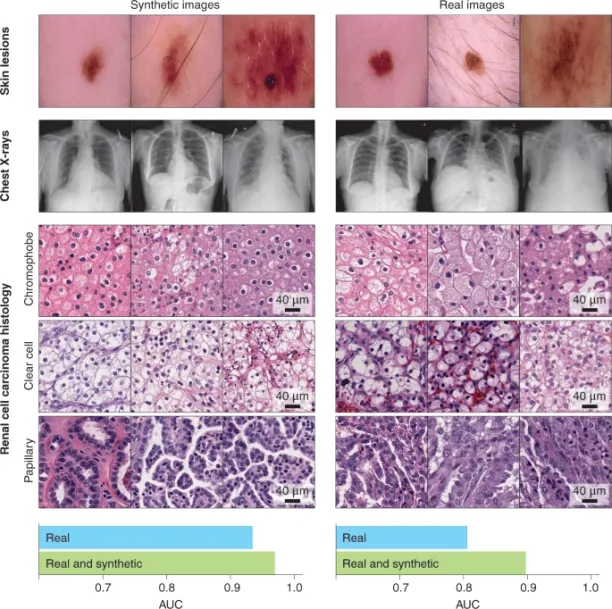
Improves machine learning model accuracy
Machine learning and deep learning models are used in numerous AI applications in healthcare, such as medical imaging, patient data analytics, or drug discovery. Feeding these algorithms with sufficient and accurate training patient data is crucial for successful prediction.
Synthetic data improves machine learning/deep learning model accuracy by increasing the training dataset size without violating data privacy regulations.
Enables prediction of rare diseases
Conducting clinical trials with few patients leads to inaccurate results. Synthetic data can be used to create control groups for clinical trials related to rare or recently discovered diseases that lack sufficient existing data, enabling the prediction of rare diseases.
This is similar to synthetic data’s benefit of supporting ML model accuracy but this benefit can be more pronounced in cases where data is scarce.
Enables collaboration
Collaboration between medical and pharmaceutical institutions can help medical professionals quickly diagnose patients or accelerate drug discovery. Synthetic patient data that recreates the characteristics of real patients can facilitate collaboration.
Provides reproducibility for medical research
Being able to reproduce the results of a research or experiment is an important part of scientific progress. However, patient data privacy regulations can hinder reproducibility for clinical research. By conducting research on and sharing synthetic patient datasets, clinical researchers can ensure that their results are reproducible.
What are its alternatives?
Models built on real data or a combination of real and synthetic data can outperform models that rely only on synthetic data. However, real data needs to be annotated when it is in the form of an image. Annotation companies offer platforms for data annotation.
What are some case studies?
- M-sense is a migraine monitoring and health assistance mobile application. It allows users to understand and reduce their migraine symptoms. The application also provides synthetic user data based on real data to the scientific community for migraine research.
- The Office of the National Coordinator for Health Information Technology (ONC) is leading a project to enhance an open-source synthetic data engine to accelerate scientific research. They aim to generate high-quality synthetic data for opioid addiction, pediatrics, and complex care use cases.
- US Department of Veteran Affairs provides synthetic medical data for research on the factors that impact veteran health. Researchers and medical professionals can access veteran health data through Lighthouse API.
If you want to learn more about synthetic data and its applications, you can check our other articles:
- Synthetic data: Pros, Cons, Case studies & AI Applications
- Top 20 Synthetic Data Use Cases & Applications
If you are looking for synthetic data generation software, check our data-driven, sortable/filterable list of vendors.
You can also check our sortable/filterable data annotation services, and tools lists:
If you still have questions about synthetic data, do not hesitate to contact us:

Cem has been the principal analyst at AIMultiple since 2017. AIMultiple informs hundreds of thousands of businesses (as per similarWeb) including 60% of Fortune 500 every month.
Cem's work has been cited by leading global publications including Business Insider, Forbes, Washington Post, global firms like Deloitte, HPE, NGOs like World Economic Forum and supranational organizations like European Commission. You can see more reputable companies and media that referenced AIMultiple.
Throughout his career, Cem served as a tech consultant, tech buyer and tech entrepreneur. He advised businesses on their enterprise software, automation, cloud, AI / ML and other technology related decisions at McKinsey & Company and Altman Solon for more than a decade. He also published a McKinsey report on digitalization.
He led technology strategy and procurement of a telco while reporting to the CEO. He has also led commercial growth of deep tech company Hypatos that reached a 7 digit annual recurring revenue and a 9 digit valuation from 0 within 2 years. Cem's work in Hypatos was covered by leading technology publications like TechCrunch and Business Insider.
Cem regularly speaks at international technology conferences. He graduated from Bogazici University as a computer engineer and holds an MBA from Columbia Business School.
To stay up-to-date on B2B tech & accelerate your enterprise:
Follow on
Comments
Your email address will not be published. All fields are required.