A/B Testing in 2024: Why it matters, Methods & Challenges
For businesses that wish to survive in today’s highly competitive market, figuring out how to improve the conversion rate is a top priority. Businesses should build a scalable experimentation culture where A/B testing is at the core to achieve this goal.
This article explains FAQs about A/B testing including what it is, why it matters, alternative methods, applications, examples & challenges.
What is A/B testing?
A/B testing a testing method that is widely used by marketers for conversion rate optimization. They evaluate two different alternatives of a product, service, landing page, or process by splitting traffic into two equal sizes. The main purpose of A/B testing is to understand the audience better so that you can choose the version that works better.
Why is A/B testing important for businesses?
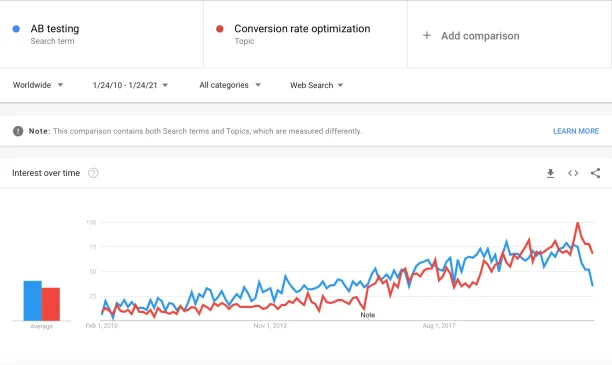
Though A/B testing is mostly associated with websites and apps, the method is almost 100 years old, and it is widely used by businesses. Studies claim that 75% of the top 500 internet retailers use A/B testing platforms. With A/B testing, businesses can
- understand pain points in the conversion funnel
- achieve higher ROI from existing traffic
- improve user experience
What if you have more than two hypotheses?
Businesses, most of the time, want to compare multiple combinations (e.g., price points, colors, etc.), and the A/B/C/D/… testing approach starts to be difficult to implement, especially for low-traffic websites. For such cases, approaches like multi-armed bandit can be preferred
What are the methods of A/B testing?
Some A/B testing methods are
- Split Testing is the most basic one. You test two different versions of the website and compare their conversion rates.
- Multivariate testing is like split testing yet, you split your audience into more than 2 pieces.
- Multi-armed bandit test is a smarter way of multivariate testing. It is designed to minimize the potential revenue loss you will experience during the testing process. First, you allocate an equal amount of traffic to each version, and as you get results, you start allocating less traffic to variations that are underperforming. There are also AI-powered tools that collect data and make allocation decisions dynamically.
What are A/B testing automation areas?
Almost all aspects of A/B testing can be automated and there are vendors within our conversion optimization vendor list that provide such AI enabled automation. The steps of the process are:
- Identifying hypotheses: Based on external factors and user metrics, machine learning models can generate hypotheses
- Creating tests: Machine learning models are able to create more attractive versions of text copy or buttons thanks to their database of best performing benchmarks
- Running tests: Almost all users automate this functionality using A/B testing tools that aim to create similar audiences for the different tests.
- Evaluating results: This is also an analytical exercise and better completed by machines
- Rolling out the new version: Human oversight may be necessary before rolling out the winning test to the full website. Sometimes anomalies that are not communicated clearly to machines, like the COVID-19 pandemic, may dramatically change user behavior and human oversight may be necessary to stop machines from making the wrong conclusions.
What are A/B testing use cases?
Common application areas of A/B testing are website, application, chatbot and email marketing testing. There are a variety of parameters you can test in these applications. Some examples are
- Titles
- Content including its tone and format
- Design and layout
- Navigation buttons
- CTAs
- Pricing – Feel free to read our research on dynamic pricing for more
- Social proofs
What are A/B testing examples?
Workzone
Workzone, a US-based project management software company, has tested changing the colors of a “what customers say” section. Their hypothesis was that customer testimonial logos were overshadowing the form. They made customer logos black and white. After 22 days of the data collection process, the change resulted in a 34% increase in form submissions and marked a 99% statistical significance.
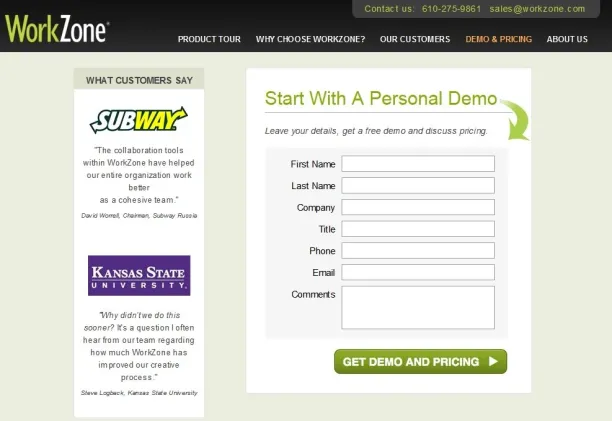
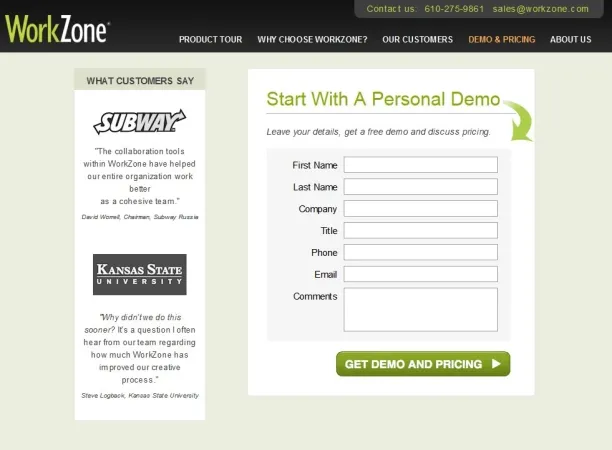
For more examples on A/B testing, check our conversion rate optimization article, where we explained best practices and real-world examples comprehensively.
What are the challenges of A/B testing?
Small sample size
Testing a website with low traffic provides inconsistent results that cannot be trusted. You should have enough visitors to have at least a 95% confidence rate statistically. Multivariate testing requires more data than split testing to provide 95% confidence rate, therefore it is almost impossible to perform multivariate tests in websites with low traffic.
Biased sample data
Biased sampling influences data-driven decision making badly. In biased samples, data is inconsistent because it is collected in such a way that some members of the intended population have a lower or higher sampling probability than others.
Creating designs that are producing statistically significant results
Every idea you test will not produce significant results yet, it will take your time and resources. Some trivial changes may boost metrics for a short time but these changes may be due to sampling biases.
Formulating a hypothesis before A/B tests is commonly skipped, leading to ineffective tests. Business should drive their experimentation via hypotheses and focus on user experience rather than colors or fonts.
If you are looking for A/B testing vendors, feel free to check our sortable and transparent vendor list, and data-driven list of testing tools.
If you want to read more about CROs, feel free to download our comprehensive whitepaper on the topic:
If you still have questions, don’t hesitate to contact us:

Cem has been the principal analyst at AIMultiple since 2017. AIMultiple informs hundreds of thousands of businesses (as per similarWeb) including 60% of Fortune 500 every month.
Cem's work has been cited by leading global publications including Business Insider, Forbes, Washington Post, global firms like Deloitte, HPE, NGOs like World Economic Forum and supranational organizations like European Commission. You can see more reputable companies and media that referenced AIMultiple.
Throughout his career, Cem served as a tech consultant, tech buyer and tech entrepreneur. He advised businesses on their enterprise software, automation, cloud, AI / ML and other technology related decisions at McKinsey & Company and Altman Solon for more than a decade. He also published a McKinsey report on digitalization.
He led technology strategy and procurement of a telco while reporting to the CEO. He has also led commercial growth of deep tech company Hypatos that reached a 7 digit annual recurring revenue and a 9 digit valuation from 0 within 2 years. Cem's work in Hypatos was covered by leading technology publications like TechCrunch and Business Insider.
Cem regularly speaks at international technology conferences. He graduated from Bogazici University as a computer engineer and holds an MBA from Columbia Business School.
To stay up-to-date on B2B tech & accelerate your enterprise:
Follow on
Comments
Your email address will not be published. All fields are required.