AI adoption is on the rise. 98% of companies are experimenting with AI, while 26% are advancing to generate tangible value.1
Explore the top 5 types of AI services that can accelerate your business’s AI adoption journey.
1. AI as a Service (AIaaS)
AI as a Service (AIaaS) is a cloud-based model that delivers artificial intelligence capabilities to organizations without requiring dedicated infrastructure or hiring data scientists. It enables integration of AI systems and models through APIs, web interfaces, and chat interfaces. This simplifies the AI journey and supports scalable adoption of machine learning and generative AI solutions.
AIaaS allows businesses to leverage a comprehensive platform to build and deploy AI applications aligned with business objectives, offering faster time-to-value with lower barriers to entry.
Conversational AI / Natural language processing (NLP)
These services apply natural language technologies to improve communication and automate workflows:
- AI chatbots / conversational agents: Digital assistants that handle customer support, internal inquiries, and task automation through text or voice.
- Text analytics: Extracts insights from unstructured text using sentiment analysis, topic modeling, and entity recognition.
- Speech-to-text: Converts spoken language into text for transcription, command execution, and accessibility.
- Text-to-speech: Transforms text into human-like speech, supporting IVR systems and improved accessibility.
Computer vision
Computer vision capabilities enhance interpretation of visual data in business contexts:
- Emotion detection: Analyzes facial expressions to determine emotional states, relevant for customer experience and mental health applications.
- Image recognition: Detects and classifies objects, scenes, or text in images, used in retail, security, and diagnostics.
- Video analysis: Monitors and interprets video content to track events or behaviors in surveillance, media, and sports.
Document understanding
These tools improve productivity and accuracy in document processing:
- Document data extraction: Uses Optical Character Recognition (OCR) and NLP to extract text and key fields from documents such as invoices and contracts, enabling automation and compliance.
Analytics solutions
AI systems applied to business data enable forecasting and anomaly detection:
- Demand forecasting: Uses machine learning on historical data to predict customer demand and optimize inventory.
- Fraud detection: Identifies irregular patterns in financial data to detect fraudulent activity.
- Recommendation systems: Suggests content or products based on user behavior and own data to increase engagement.
Other services
Additional AI capabilities that support broader enterprise use cases:
- Knowledge mapping: Organizes data across systems to enhance discoverability and support decision-making.
- Predictive modeling: Analyzes historical patterns using AI models to forecast business outcomes.
- Security solutions: AI-powered tools that detect threats, automate responses, and safeguard digital assets.
- Automated code review: Evaluates software code for vulnerabilities, inefficiencies, and standards compliance to improve quality and security.
AIaaS enables organizations to explore, develop, and scale AI systems across multiple domains. By offering access to generative AI models, foundation models, and task-specific solutions, these platforms support real results in customer experiences, operational efficiency, and business outcomes.
Top 11 AIaaS providers
Company | Average rating | Use Cases | Pricing Model |
---|---|---|---|
Microsoft Azure AI | 4.4 based on 2,702 reviews | NLP, Vision, ML, Chatbots, Form Recognition | Pay-as-you-go, subscription-based. |
IBM Watson | 4.4 based on 274 reviews | NLP, Chatbots, Speech-to-Text, Language Translation | Pay-as-you-go with options for monthly subscriptions. |
Amazon Web Services (AWS) AI | 4.8 based on 82 reviews | ML, NLP, Vision, Speech, Fraud Detection | Pay-as-you-go, with additional costs for certain products. |
DataRobot | 4.7 based on 62 reviews | ML, Predictive Modeling, Automation | Subscription-based. |
BigML | 4.9 based on 25 reviews | Predictive Modeling, Clustering, Anomaly Detection | Pay-as-you-go, subscription-based. |
Clarifai | 4.5 based on 22 reviews | Vision, Image/Video Recognition, Custom Models | Pay-as-you-go, subscription-based for enterprise options. |
Google Cloud AI | 4.4 based on 8 reviews | NLP, Vision, Speech-to-Text, ML, AutoML | Pay-as-you-go, subscription-based for specific products. |
Hugging Face | 4.9 based on 6 reviews | NLP, Text Generation, Custom Model Training | Open-source, pay-as-you-go for API calls. |
OpenAI (API) | 3.8 based on 3 reviews | NLP, Text Generation, Code Generation, Vision | Pay-as-you-go, with pricing based on tokens or compute usage. |
C3.ai | 4.5 based on 1 reviews | Predictive Maintenance, Fraud Detection, Industry-Specific AI | Subscription-based. |
Runway | 5.0 based on 1 reviews | Creative AI, Generative Media, Video Editing | Pay-as-you-go, subscription-based. |
Notes:
- The providers selected offer a broad range of AI services, including natural language processing (NLP), computer vision, machine learning (ML), deep learning, and speech-to-text. This ensures that they cater to various use cases, from basic automation and predictive analytics to more advanced tasks like generative AI and model training.
- All providers in the list are cloud-based, meaning they offer scalable, flexible solutions that are accessible via APIs or platform-based services.
- The providers cater to both developers (offering APIs, model training, and customization) and enterprises (with ready-made solutions, integration capabilities, and security features).
Machine learning in AIaaS
Machine learning, a core component of AI, allows models to learn from historical data, refine predictions, and adapt over time. Within the AIaaS ecosystem, companies can train and fine-tune AI models using their own data, ensuring that solutions align with their specific business context.
2. Custom AI development
Custom generative AI models, foundation models, and intelligent agents are increasingly being used to support industries ranging from enterprise applications to mobile services.
Despite the growing interest in AI adoption, challenges remain. According to the IBM report (See Figure 1), one challenge hindering AI adoption is insufficient proprietary data to customize models.
Building a custom solution may be necessary for cases when the off-the-shelf AI system doesn’t exist or is insufficient for your company’s needs. You can either build an in-house solution or hire outsourcing partners.
The right choice depends on:
- Your business’s AI capabilities.
- Data science knowledge of your employees.
- Budget for the project.
- Ownership of data.
- Privacy requirements for your data.
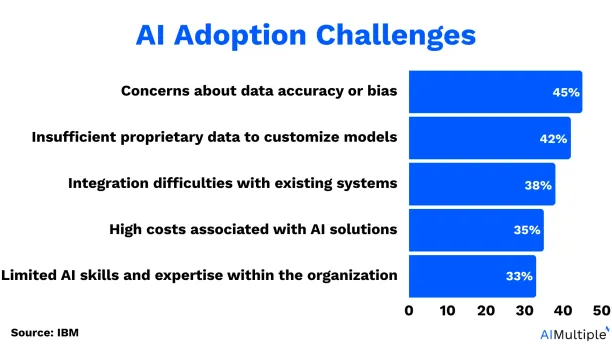
Figure 1: Top 5 AI adoption challenges.2
3. Services for enabling internal AI development
Organizations aiming to advance their AI journey require supporting services that facilitate the development, deployment, and management of AI models.
These services help integrate AI capabilities internally, optimize the AI lifecycle, and align AI efforts with broader business objectives.
3.1. Consulting
If your company is new to AI and can invest significantly in AI transformation, you can consider hiring AI consultants. Since AI projects are filled with challenges, the experience of AI consultants in the market can help you avoid common pitfalls and apply best practices, such as reducing bias in the dataset.
AI consulting services include:
- Assessing the maturity of your company’s AI transformation.
- Identification of areas where leveraging AI systems or machine learning can create value.
- Formulating an AI strategy to launch new pilot products/services.
- Building AI solutions.
- Training your employees for upcoming AI technology implementations.
For more on AI consulting and consultants, check out AI consulting.
3.2. AI talent recruitment
As the demand for AI expertise grows, recruiting AI talent has become essential to maintaining competitiveness. Businesses face challenges in sourcing skilled data scientists and AI engineers due to a limited supply of professionals.
- Partnering with on-demand recruiting services: Companies engage with specialized recruiting firms to access pre-vetted AI and data science professionals.
- Flexible hiring models: Includes a mix of full-time hires and contract-based experts to meet dynamic project needs.
This approach supports rapid scaling of AI capabilities while controlling costs and increasing access to specialized skills.
3.3. Data collection
High-quality data is critical for training effective AI models. Developing datasets for generative AI models and large-scale machine learning applications often requires extensive effort.
- Working with data collection providers: Businesses collaborate with vendors that curate domain-specific and task-specific datasets.
- Ensuring relevance and scale: Services are designed to match the business context and ensure the volume and diversity required for reliable model performance.
These services are especially valuable in developing LLMs, where training data impacts model accuracy and fairness.
For example, Clickworker offers scalable AI datasets through a crowdsourcing platform. Its global network of over 4.5 million workers fulfilled the data needs of 4 out of 5 tech giants in the U.S., including Google, Samsung, Microsoft, and Apple.
3.4. RLHF (Reinforcement Learning from Human Feedback) services
RLHF is an approach within the broader spectrum of reinforcement learning (RL). In RLHF, the usual rewards coming from the environment are combined with or replaced by feedback derived from humans. This becomes especially useful when obtaining real-world rewards is impractical or too expensive.
Working with an RLHF partner offers businesses standardized workflows for training models with human feedback. An RLHF partner brings expertise in integrating human insights with machine learning, ensuring that AI systems are trained more safely, ethically, and aligned with nuanced human values.
By collaborating with a specialized partner, businesses can leverage this hybrid training approach without the steep learning curve, accelerating AI project timelines and achieving more reliable and human-centric outcomes.
Since RLHF requires a high level of human intervention, service providers usually offer it through a crowdsourcing platform where a large network of workers conducts RLHF in the form of micro-tasks.
3.5. Data labeling
Supervised learning, a core component of many AI systems, relies on accurately labeled data for training. Multiple approaches are used to generate labeled datasets:
- In-house development: Internal teams handle data annotation using business-specific standards.
- Outsourced employees: External contractors label data based on detailed guidelines.
- Data labeling agencies: Specialized firms offer scalable annotation services with domain expertise.
- Crowdsourcing: A distributed workforce provides annotations at scale, suitable for less specialized tasks.
Each method supports different stages of the AI lifecycle and varies in terms of quality control, scalability, and cost.
3.6. Data science competitions
Organizations can use data science competitions to enhance model development:
- Crowdsourced model building: Competitions attract developers and data scientists to solve defined AI problems.
- Operational focus for internal teams: Internal teams can concentrate on deploying and maintaining models rather than building them from scratch.
This model supports innovation, reduces deployment time, and expands access to external expertise.
3.7. AI / MLOps platforms
AI and MLOps platforms manage the development, deployment, and management of AI applications:
- Model building and deployment at scale: These platforms automate workflows from data preparation to model monitoring.
- Integration with existing systems: Supports faster transition from experimental models to production-ready AI products.
- Support for responsible AI: Provides tools for bias detection, auditability, and performance tracking.
AI/MLOps platforms enable real results by operationalizing AI models, reducing latency, and improving productivity in AI initiatives.
4. AI hardware and infrastructure services
As AI and machine learning models grow in complexity and size, the demand for specialized hardware and infrastructure has significantly increased. The computational requirements of training deep neural networks, running simulations for reinforcement learning, or serving millions of predictions in real-time have transcended the capabilities of conventional hardware.
4.1. Types of specialized hardware:
- GPUs (Graphics Processing Units): Initially for graphics rendering, GPUs now power AI with their parallel processing, ideal for neural network computations.
- TPUs (Tensor Processing Units): Google’s ASICs, designed for deep learning, optimize tensor operations for faster, more efficient neural network performance.
- FPGAs (Field-Programmable Gate Arrays): Reconfigurable post-manufacturing, FPGAs balance GPU flexibility and TPU specialization, supporting AI training and inference.
4.2. Infrastructure services:
- Cloud Services: These scalable, pay-as-you-go services provide AI-optimized infrastructure, letting users rent GPUs, TPUs, or FPGAs on demand.
- On-Premises Solutions: For businesses needing greater control over data due to security or regulations, on-premises hardware integrates specialized racks into company data centers.
5. Model monitoring and maintenance
Once AI models are transitioned from the development stage to production, post-deployment models require ongoing monitoring. These models interact with real-world data, which is dynamic and can change over time. Such changes necessitate regular monitoring and maintenance of these models to ensure consistent performance.
As organizations struggle to fill the AI talent gap, working with model monitoring and maintenance partners can help business leaders sustain the performance of their AI solutions.
Conclusion
The use of AI services is becoming a core operational requirement. Companies moving from experimentation to scaled deployment are better positioned to meet business objectives. This requires using AI tools that match specific business needs, supported by infrastructure, talent, and data strategies.
AI should not be approached as a one-time project. It is an ongoing process that involves building, deploying, monitoring, and improving AI models. Organizations that treat AI as part of their long-term strategy will be better prepared to create measurable results, improve productivity, and maintain operational efficiency.
FAQ
What are AI services?
AI services, including generative AI, offer prebuilt machine learning models that simplify AI integration into applications and business operations. Cloud solutions like Azure AI provide tools such as large language models and chatbots, accessible via APIs. These services help developers analyze unstructured text, enhance customer experiences, and create intelligent apps without extensive data science expertise.
Why are AI services important?
AI services are important because they enable organizations to integrate advanced AI capabilities, such as natural language processing and image recognition, into their applications and operations, enhancing customer experiences and driving innovation. These cloud-based services, like Azure AI, offer scalable and cost-effective solutions, allowing businesses to leverage prebuilt and customizable models without needing extensive data science expertise. This results in improved efficiency, actionable insights, and the ability to meet specific business needs in real time.
External Links
- 1. Where’s the Value in AI?. BCG Global
- 2. AI Adoption Challenges | IBM.
Comments
Your email address will not be published. All fields are required.