Guide to Hyperautomation in 2024: Technologies, Pros & Cons
Hyperautomation is a term initiated by Gartner. It is the application of automation technologies like RPA and process mining along with machine learning and other emerging technologies to increase the level of automation and digital transformation in companies.
Given the interest in RPA, traditional industry analysts want to capitalize on the growth of enterprise automation and they make up new terms as part of that effort. However, the fact that they made up this specific term also shows that companies are striving to achieve higher levels of automation and are not satisfied by current enterprise automation technologies.
For more on hyperautomation:
What is hyperautomation?
Different industry analysts prefer different names for the concept of increased enterprise automation. Based on all the definitons we see 4 aspects of hyperautomation in enabling higher levels of automation:
- Use of existing automation/digital transformation technologies like RPA and process mining
- Reliance on machine learning to automate operational decision making
- Organizational and cultural change to drive fast experimentation and rapid adoption of automation technologies
- Process simplification to reduce automation challenges
Gartner
Hyperautomation: “As no single tool can replace humans, hyperautomation today involves a combination of tools, including robotic process automation (RPA), intelligent business management software (iBPMS) and AI, with a goal of increasingly AI-driven decision making.”
Gartner explains that the term “hyperautomation” is different than automation because it is not only about products nor services. They define hyperautomation to include significant changes in the company from IT infrastructure to designing approaches of business processes and decisions, therefore, hyperautomation is a holistic approach to automation. This is a familiar pattern, in any change initiative that fails to achieve the change it targets, outsiders such as consultants and industry analysts stick to blaming lack of cultural change. They are partially right, cultural change is indeed necessary but as importantly, different automation tools need to be more interoperable and provide higher levels of automation for automation to be widespread.
AIMultiple
AI Transformation: We believe that the next phase of automation should focus on automating end-to-end operational decision making using machine learning. Our definition of AI transformation accurately describes the gist of hyperautomation. Repetitive processes relying on rules based decision making is already automated at leading edge companies. With advances in AI, more complex operational decision making can also be automated.
Forrester
Digital Process Automation is defined it as “aligning processes on the back-end to support a true end-to-end customer experience.” The focus on process is relevant as complex processes are harder to automate and hold back organizations’ automation efforts. Even when such processes are automated, they are harder to validate, leading to longer pilots and slower adoption. Though increased machine intelligence is making this easier, current state of automation definitely favors simpler processes.
IDC
Intelligent Process Automation (IPA): “IPA is a strategic business imperative for enterprises to accelerate digital transformation by focusing on business process transformation and automation”
To add to the confusion, vendors also use different terminology to imply the same thing:
- Workfusion: Intelligent automation platform
- Cognitive process automation
Why is hyperautomation popular?
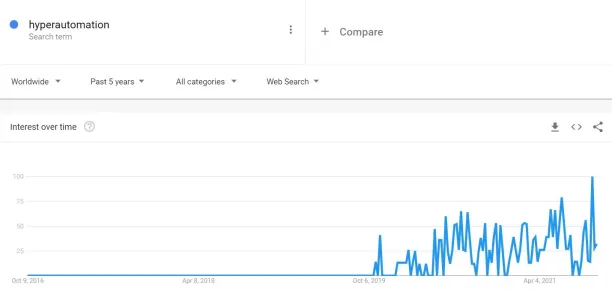
Interest in hyper automation has begun at the end of 2019. Most terms made up by Gartner do not stick, sometimes Gartner comes up with terms like AI Based Accounts Payable Invoice Automation (APIA) which sound neither cool nor easy-to-understand. However, hyperautomation is becoming more commonly used, it is being picked up by vendors like UiPath. It is attractive to vendors since it is
- short
- something that companies aspire to
- vague so any vendor can claim to enable it. Most automation technologies are included in the definition so pretty much any emerging technology can be associated with hyperautomation.
Why is it important now?
Because it has significant potential for impact and companies are frustrated with the current level of progress in their automation efforts.
Gartner, without citing any quantitative backup, claims that organizations will lower operational costs by 30% by combining hyperautomation technologies with redesigned operational processes by 2024. The number is probably wrong but it is beyond doubt that automating operational decision making will be impactful and will be a focus area for companies.
Due to several issues, traditional, product based automation approaches with limited reliance on machine learning have failed to deliver significant benefits:
- Process complexity has slowed down automation efforts even in rules based processes
- Employees have yet to adopt a culture of looking for automation opportunities and rapidly experimenting with new technologies
- Most efforts did not rely on building custom machine learning models which limited application areas
All of these lead companies to look for different approaches.
How is it different than automation?
Though RPA has been the fastest-growing segment of the global enterprise software market and the payback period is shorter than other digital transformation technologies, RPA has limitations such as its reliance on structured data. A traditional RPA software cannot understand the context and learn itself by using unstructured data. It requires humans to code rules-based tasks, interpret data, and make decisions about automation.
Compared to conventional RPA automation, hyperautomation shifts the focus of automation to decision making and more complex work. It also includes more focus on process simplification and culture in enabling automation.
Top 6 technologies enabling hyperautomation
Robotic Process Automation (RPA)
RPA is at the core of hyperautomation.
Combining technologies enables RPA to become more intelligent and extends the reach of RPA.
If you want to learn more on RPA, feel free to read our following articles or visit our sortable, up-to-date RPA vendor list:
- 100+ RPA Use cases/ Applications/ Examples for all Industries
- RPA Architecture: In-depth Guide to How RPA Works
- Become an RPA Developer in 2022
Intelligent Business Process Management Suites (iBPMS)
An iBPMS is an integrated set of technologies that coordinates people and machines in process delivery. An iBPMS enables companies to model, implement, and execute sets of interrelated processes by applying business rules.
Though it is less popular with the emergence of RPA, it is still a useful tool for process automation.
Process Mining
Process mining is an analytical discipline to gain a deep understanding of a company’s processes. Process mining has a broad range of use cases from common applications such as process optimization to industry-specific applications like risk identification in an audit. Process mining is critical for process simplification and process understanding which are major enablers of hyperautomation. You can discover all the latest process mining software vendors in the market from our comprehensive list.
APIs
The technology and frameworks to provide black box software interfaces has been around for a long time. Jeff Bezos famously pushed all Amazon to embrace APIs back in 2002. However, even today numerous enterprises rely on legacy interfaces to exchange data between applications. Embracing APIs facilitates machine-to-machine communication and therefore automation.
Computer Vision
Computer vision (CV) is a combination of AI techniques including image classification and segmentation, and object detection and tracking, which enable machines to interpret information from unstructured data such as images and videos.
Natural Language Processing (NLP)
NLP helps businesses automate tasks that knowledge workers would do. It enables machines to understand unstructured data from emails, social media posts, videos. Then it performs sentiment analysis, automatic language translation or automatic classification of texts into categories depending on your business’ automation needs. Thanks to NLP technology, RPA bots can understand the context of the task.
To explore NLP use in-depth, read our article on top 30 NLP use cases for different business functions.
Other Artificial Intelligence/ Machine Learning
Artificial intelligence (AI) mimics human intelligence processes by machines, and machine learning is the subcategory of AI that contains learning algorithms for machines. Organizations use machine learning to carry out specific tasks without explicit programming, relying on data.
If you want to learn more on AI, feel free to read our following articles:
- 100+ AI Use Cases / Applications
- AI in analytics: How AI is shaping analytics in 2020
- AI in Automation: Discover tasks to automate with AI
Optical Character Recognition (OCR)
OCR can eliminate manual labor for certain tasks. OCR can extract data in documents and convert it machine-readable characters. When it is combined with AI and RPA, businesses can automate the processes where human interaction is needed to capture data from documents, analyze it and take action to handle the task.
Digital Twin of an Organization (DTO)
A digital twin is a virtual replica of a product, service, or process. A digital twin of an organization (DTO) makes the previously unseen interactions between processes, functions, and key performance indicators visible by the organization to test the results of automated processes even before automating the process. To learn more about digital twin of an organization applications in business, feel free to check our in-depth guide on DTOs.
You can also check our article on hyperautomation trends to learn more about the most important technologies that enables hyperautomation.
Which processes are ripe for hyperautomation?
Applications of hyperautomation vary depending on which technologies are combined. Some example use cases could be:
- Customer support using conversational AI and RPA: Customer support services involve processes that require understanding and replying emails, questions, and queries, as well as making changes to customer data. By combining these technologies, companies can automatically respond to customer queries.
- Approving financial transactions: With RPA, businesses can extract relevant information on user’s transactions from different data sources. Then an NLP system can process this information and perform analytics to identify any fraudulent actions.
- Claim processing for auto insurance: RPA has been used to automate claims processing by insurance companies. With the integration of computer vision and NLP, auto insurers can process images about car crashes and understand the damages covered by the customers’ policy. They can then use this data to identify whether the claim is covered with the current contract or not.
Hyperautomation is not limited to above applications. If you want to learn more on hyperautomation processes, feel free to read our article on hyperautomation examples.
What are the benefits of hyperautomation?
Automation initiatives mainly focus on cost reduction and increase in compliance. In addition to those, top benefits of hyperautomation for businesses include:
- Agility: The business doesn’t need to rely on a single technology for automation purpose. Reliance on a suite of tools along with cultural change, enable organizations to achieve scale and flexibility in operations.
- Enabling employees and improving their productivity: Automation frees the time of employees so that they can focus on more value-added tasks.
- Improved collaboration: Hyperautomation enables businesses integrate digital technologies across their processes and legacy systems. With the integration of technologies, stakeholders have better access to data and can communicate seamlessly throughout the organization.
You can check our comprehensive article on the benefits of hyperautomation for businesses.
What are the challenges of hyperautomation?
Technologies that are a part of hyperautomation may complement each other. For example, RPA bots can enable the integration of other technologies with legacy systems. However, there are still significant challenges due to the limitations of these technologies as well as organizational challenges. Common challenges are:
- Challenges in using AI in automation:
- Training data may be unavailable or may include personal data: Risk of data privacy issues may occur when you share personal data with AI vendors, yet, you can’t build everything yourself. Therefore, companies should invest in privacy enhancing technologies such as data masking.
- Training data creation can be slow. Synthetic data sets can speed up training data generation in some cases.
- Edge cases: In any complex process that is automated with machine learning, there will be cases when humans need to step in. An easy-to-use human-in-the-loop solution is critical for the success of AI in automation
- AI systems may contain biases that are either in training data or embedded into algorithm via prejudiced assumptions.
- For more, please see our article on challenges of AI.
- Challenges in process simplification:
- Lack of process understanding: Most processes are not well documented. Process mining tools can help organizations understand processes that rely on log files but still important process information such as the content of calls are hard to analyze, creating challenges.
- Specific customer demands: Custom solutions for specific customer needs increase customer satisfaction but reduce maintainability and introduce process complexity. Companies need to smartly trade-off between process simplification and customer satisfaction. A customer with extremely complex requirements may not be the right one to be served with a standardized process serving many other customers.
- Organizational challenges: Avoidance of potential errors and inertia are responsible for most cases of slow adoption of automation. Employees should be empowered to run experiments on sandboxes and digital twins to quickly see the impact and challenges of potential automation technologies.
If you are looking for vendors that can provide necessary technologies to achieve hyperautomation, check our data-driven lists for:
- Automation tools and software
- OCR tools
- Machine learning APIs
- Process intelligence tools
- Digital twin software
And don’t hesitate to contact us:

Cem has been the principal analyst at AIMultiple since 2017. AIMultiple informs hundreds of thousands of businesses (as per similarWeb) including 60% of Fortune 500 every month.
Cem's work has been cited by leading global publications including Business Insider, Forbes, Washington Post, global firms like Deloitte, HPE, NGOs like World Economic Forum and supranational organizations like European Commission. You can see more reputable companies and media that referenced AIMultiple.
Throughout his career, Cem served as a tech consultant, tech buyer and tech entrepreneur. He advised businesses on their enterprise software, automation, cloud, AI / ML and other technology related decisions at McKinsey & Company and Altman Solon for more than a decade. He also published a McKinsey report on digitalization.
He led technology strategy and procurement of a telco while reporting to the CEO. He has also led commercial growth of deep tech company Hypatos that reached a 7 digit annual recurring revenue and a 9 digit valuation from 0 within 2 years. Cem's work in Hypatos was covered by leading technology publications like TechCrunch and Business Insider.
Cem regularly speaks at international technology conferences. He graduated from Bogazici University as a computer engineer and holds an MBA from Columbia Business School.
To stay up-to-date on B2B tech & accelerate your enterprise:
Follow onNext to Read
Hyperautomation in Banking: Use Cases & Best Practices [2024]
Healthcare Hyperautomation: Use Cases & Best Practices [2024]
Top 12 Hyperautomation Use Cases & Examples in 2024
Hey Cem,
I found it interesting how different industry analysts have their own terms for this concept, yet the common thread is clear – achieving more automation. The insights you provided from Gartner, AIMultiple, Forrester, and IDC offer a well-rounded perspective on hyper automation’s significance. And the explanation of top technologies enabling hyperautomation, such as RPA, iBPMS, and AI, makes the whole concept more tangible.
Thank you for such insightful content.
Regards,
Shyama Patel
Comments
Your email address will not be published. All fields are required.