Gartner identified generative AI as a top technology trend for the banking and investment industry in the last years.1 Generative AI’s contributions to data privacy, fraud detection, and risk management can be critical to financial services companies.
Key use cases include fraud detection, customer service automation, credit risk assessment, and document processing.
Fraud Detection
Detecting anomalous and fraudulent transactions is one of the applications of generative AI in the banking industry. In a study, researchers constructed a training set of fraudulent transactions and then utilized it in a Generative Adversarial Network (GAN), a generative model based on deep neural networks, to produce synthetic fraudulent transactions.2 The synthetic data was then compared to the genuine data to see whether the GAN was capable of developing sensitivity to identify anomalous transactions. Finally, it is seen that using a GAN-enhanced training set to detect such transactions outperforms that of the unprocessed original data set.
As a result of this study, it appeared that training GANs for the purpose of fraud detection produced successful outcomes because of developing sensitivity after being trained to identify underrepresented transactions. This is an especially important application for financial services providers that deal with enormous number of transactions.
Case Study for Fraud Detection via Generative AI in Banking
Swedbank used GANs to detect fraudulent transactions.3 GANs are trained to learn legal and illegal transactions in order to detect the fraudulent ones by creating graphs that reveal their patterns.
However, employing GANs for fraud detection has the potential to generate inaccurate results (see Figure 1), necessitating additional improvement. Explore machine learning accuracy to learn more.
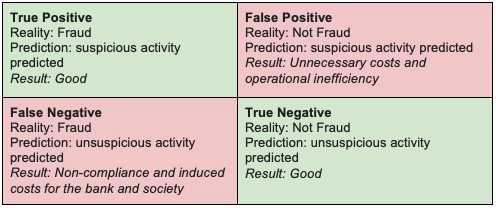
(Figure 1. Source: “Challenges in modeling fraud as a binary classification problem”)
Data Privacy
The use of synthetic data has the potential to overcome the challenges that the banking industry is facing, particularly in the context of data privacy. Synthetic data can be used to create shareable data in place of customer data that cannot be shared due to privacy concerns and data protection laws. Further, synthetic customer data are ideal for training ML models to assist banks determine whether a customer is eligible for a credit or mortgage loan, and how much can be offered.
GANs are capable of producing synthetic data (see Figure 2) and thus appropriate for the needs of the banking industry. Synthetic data generation can be achieved by different versions of GAN such as Conditional GAN, WGAN, Deep Regret Analytic GAN, or TimeGAN.
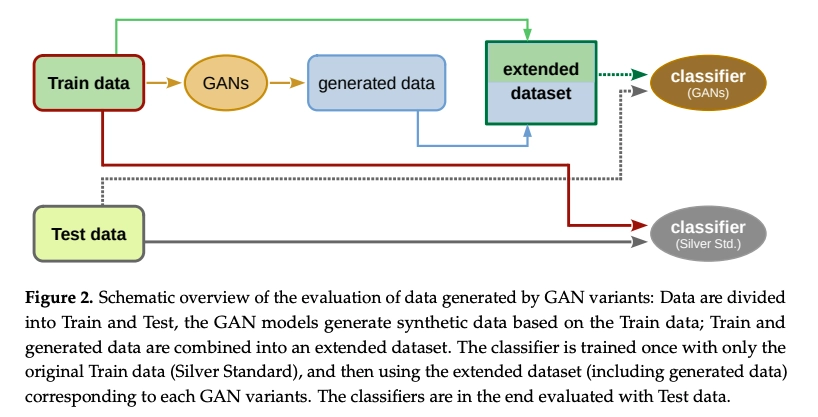
(Figure 2. Source: “GAN-Based Approaches for Generating Structured Data in the Medical Domain”)
Risk Management
Establishing a risk management plan is essential for banks to maintain an appropriate level of risk exposure, identify possible risk areas, and take action to preserve profitability. Banks may suffer losses if liquidity, credit, operational, and other risks are not appropriately handled.
Besides certain software systems for risk minimization, the use of generative AI is one possible solution for minimizing such losses resulting from the lack of adequate risk management.
With GANs, it is possible to calculate value-at-risk estimations that show the potential amount of loss in a particular period of time or to create economic scenarios that are useful for predicting the future of financial markets.4 5
GANs facilitates comprehending the possibilities of volatility by generating novel and assumption-free scenarios (See Figure 3) based on historical data patterns.
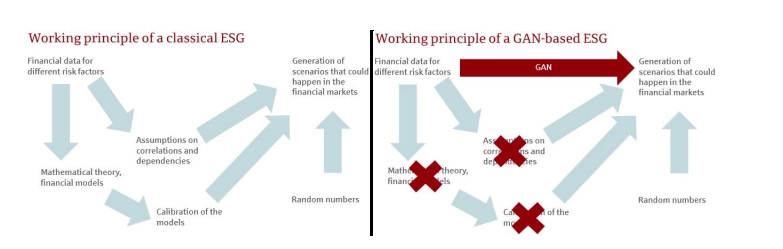
(Figure 3. Source: “Scenario generation for market risk models using generative neural networks”)
Generating Applicant-Friendly Denial Explanations
Loan decisions are one of the uses of AI in the banking sector. AI enables banks to determine whether a customer is qualified for credit lending by risk scoring, as well as make informed decisions about the maximum credit limit that a customer can have, and loan pricing based on the assessed risks.6
Nevertheless, it’s not only decision makers who need insights—loan applicants also require clear explanations of AI-driven decisions, particularly to understand why their applications may have been denied. The reason for such a need is to ensure user trust as well as to increase customer awareness so that they can make more informed applications in the future.
In this context, a conditional GAN— a variant of GAN in which the generator and discriminator are conditioned on class labels —is useful for generating applicant-friendly denial explanations (See Figure 4). A two-level conditioning framework is established by arranging the causes of denial from simple to complex in a hierarchical structure, generating more transparent and comprehensible explanations.
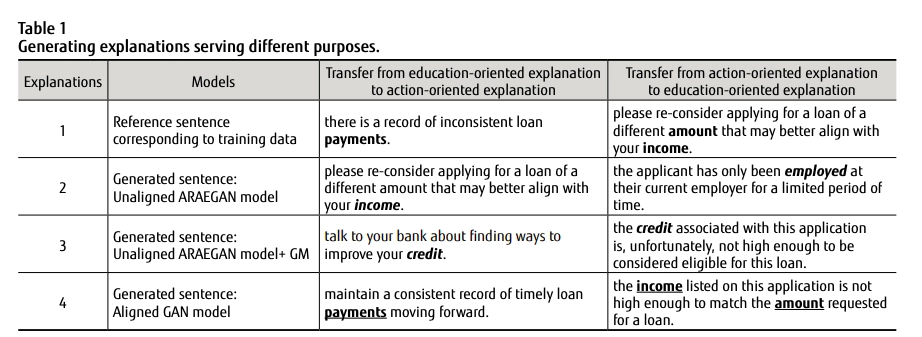
(Figure 4. Source: “Generating User-Friendly Explanations for Loan Denials Using Generative Adversarial Networks”)
For all GenAI applications in financial services, not just in banking, read our article on generative AI in financial services.
The latest regulations require generative AI tools to ensure AI compliance, manage an AI inventory, mitigate AI bias, and other generative AI risks. One way to overcome these challenges is to deploy AI governance tools and responsible AI platforms.
For more information on other financial technology capabilities of AI, feel free to read our articles:
- KYC Automation: 5 Technologies That Increase Transparency
- Anti Money Laundering Algorithms: Tackling AML with AI
FAQ for Generative AI in Banking
How does generative AI enhance personalized customer interactions in banking?
Generative AI can process and analyze vast amounts of customer data to create tailored interactions. It can:
Generate Personalized Content: AI algorithms can generate personalized responses and recommendations based on a customer’s transaction history, financial goals, and behavior.
Automate Routine Queries: Chatbots and virtual assistants powered by generative AI handle common queries, reducing wait times and enhancing response accuracy.
Optimize Marketing Campaigns: By generating tailored marketing content, generative AI allows banks to offer relevant products, increasing customer satisfaction and engagement.
In what ways does generative AI improve fraud detection and prevention?
Generative AI enhances fraud detection by identifying patterns and anomalies across customer transactions and account activities. Key benefits include:
Real-Time Analysis: AI models can continuously analyze transactions and flag suspicious activities in real-time, allowing for immediate action.
Behavioral Analysis: Generative AI learns and adapts to recognize typical customer behaviors, detecting deviations that may indicate fraud.
Synthetic Data Generation for Training Models: AI can generate synthetic data to train fraud detection models, helping improve the detection accuracy without compromising customer data privacy.
What role does generative AI in banking play in risk management and compliance?
Generative AI assists banks in meeting regulatory standards and managing risk by automating complex compliance tasks:
Automated Document Processing: AI can review and generate compliance documentation, reducing manual workloads.
Real-Time Compliance Checks: AI continuously monitors activities for regulatory adherence, helping banks stay compliant with changing regulations.
Enhanced Risk Assessment: Generative AI produces detailed risk profiles by analyzing customer data, transaction history, and market indicators, enabling proactive risk mitigation.
What challenges do banks face in implementing generative AI?
Key challenges include:
Integration with Legacy Systems: Many banks operate on legacy systems that are incompatible with AI, requiring costly updates.
High Data Quality Requirement: Generative AI in banking models depend on high-quality data for accurate outputs; poor data quality can hinder AI performance.
Compliance and Regulatory Complexity: Banking regulations are complex and continually evolving, making it challenging to stay compliant when deploying new AI models.
Resource and Skill Gaps: Implementing AI requires specialized knowledge and resources that many banks need to acquire or develop.
How will generative AI in banking evolve in the future?
The future of generative AI in banking will likely involve:
More Personalization: AI will provide even more refined, real-time personalization as algorithms become more sophisticated.
Increased Automation: AI-driven automation will expand, covering more banking functions, including underwriting, loan approvals, and risk management.
Enhanced Security Features: AI will continuously improve fraud detection capabilities, offering real-time intervention tools.
Integration with Other Emerging Technologies: Generative AI may combine with blockchain, IoT, and quantum computing to further revolutionize financial services.
External Links
- 1. “Gartner Identifies Three Technology Trends Gaining Traction in Banking and Investment Services” (Press release). Stamford, Connecticut: Gartner. May 24, 2022. Retrieved November 11, 2022.
- 2. “Fiore U.; De Santis A.; Perla F.; Zanetti P.; Palmieri F. (2019).
“Using generative adversarial networks for improving classification effectiveness in credit card fraud detection”. Information Sciences. Volume 479: 448-455.
- 3. Detecting Financial Fraud Using GANs at Swedbank with Hopsworks and NVIDIA GPUs | NVIDIA Technical Blog. NVIDIA Technical Blog
- 4. “Fiechtner L. (2019). “Risk Management with Generative Adversarial Networks”. [MSc Dissertation, University of Oxford]
- 5. Scenario Generation for Market Risk Models Using Generative Neural Networks.
- 6. “AI-powered decision making for the bank of the future” (PDF). McKinsey & Company. March 2021. Retrieved November 11, 2022.
Comments
Your email address will not be published. All fields are required.