Process analysis is the first step to process visualization, improvement and management, which is why process analysis has been gaining popularity, as Figure 1 shows.
Despite the increasing interest, only 15% of business processes are analyzed and managed properly as BPM statistics show. One reason behind such a low rate is the lack of expertise and toolset.
Therefore, this article will explain the top 18 process analysis tools under three categories:
What is process analysis?
Business process analysis evaluates the processes to identify the nature and the root cause of the inefficiencies. Process analysis can:
- Improve processes
- Automate redundant tasks and activities
- Ensure standardization and compliance.
Process analysis can be achieved through various diagrams, charts and software. These tools are explained below based on the category.
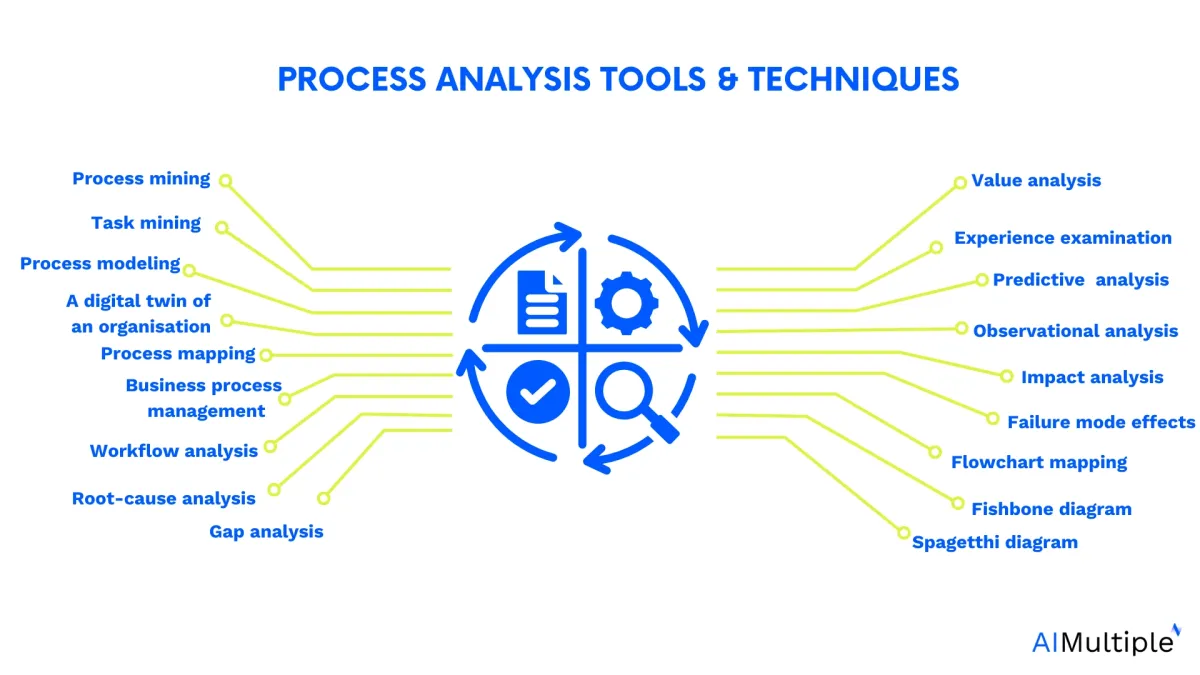
Process analysis software
Business process analysis software refers to technologies that can be applied to extract, discover and model process data for analysis purposes. These tools are also known as automated business process analysis methods.
Automated business process analysis techniques provide a more accurate, efficient, and objective approach to studying business processes compared to traditional manual methods.
Here are some top automated business process analysis platforms listed in alphabetical order within their tool category, with their average score and the total number of reviews on the major B2B user review platforms:
Products | Tool type | Rating |
---|---|---|
Appian | Process Mining | 4.3 based on 617 reviews |
Celonis | Process Mining | 4.3 based on 431 reviews |
IBM process mining | Process Mining | 4.4 based on 215 reviews |
Apromore | DTO | 4.5 based on 18 reviews |
iGrafix | DTO | 4.7 based on 126 reviews |
QPR ProcessAnalyzer | DTO | 4.0 based on - reviews |
ARIS Process Mining | Task Mining | 4.2 based on 163 reviews |
Microsoft Power Automate | Task Mining | 4.5 based on 524 reviews |
Pega Platform | Task Mining | 3.5 based on 344 reviews |
Lucidchart | Process Modelling Software | 4.5 based on 6,674 reviews |
Visio | Process Modelling Software | 4.4 based on 3,671 reviews |
Quickbase | Process Modelling Software | 4.5 based on 1,299 reviews |
Process Street | BPM | 4.5 based on 985 reviews |
Smartsheet | BPM | 4.3 based on 17,831 reviews |
Wrike | BPM | 4.2 based on 6,210 reviews |
Monday.com | Workflow management | 4.5 based on 13,988 reviews |
PowerApps | Workflow management | 4.1 based on 197 reviews |
Nintex Platform | Workflow management | {{7558265->score} based on 708 reviews |
For more tools, please checkout these lists:
- Process mining software
- Task mining software
- Process modelling software
- DTOs
- BPM software
- Workflow management software
Process Intelligence
Process intelligence platforms can collect and analyze process data to deliver process performance insights.
1. Process mining
Process mining is an analytical discipline and technology to collect, analyze and model business process data recorded in IT systems. Process Mining utilizes event logs generated by enterprise systems to create a detailed process map of a process. It identifies the sequence of tasks, their dependencies, and variations, providing an objective view of the process based on factual data.
Learn more on process mining through:
2. Task mining
Task mining or task intelligence is the technology to capture user interactions on desktops, such as task executions on spreadsheets, screen recordings or keystrokes. It’s particularly useful for processes involving a lot of human interaction.
Explore task mining benefits and 3 differences between task mining and process mining.
3. Process modeling software
Process modeling software models processes and executes these models.
Discover what are the differences between process modeling software vs. process mining.
4. A digital twin of an organization
A digital twin of an organization (DTO) creates virtual models of the processes, services, or organizational structures to simulate scenarios.
Check out DTO use cases to find out 9 more DTO applications.
5. Process mapping
Process mapping illustrates process flow using symbols to provide insights into parties involved, interactions and each step taken.
6. Business process management (BPM) software
Business process management (BPM) software automatically analyzes and manages processes and workflows.
Sponsored
Process Street is a prominent player in this field that allows users to map out their processes, identify inefficiencies, and implement corrective actions. Also, Process Street’s analytics and reporting features offer valuable insights, enabling informed decision-making for continuous process improvement and organizational success.
Learn more on BPM by reading:
Check out our data-driven list for BPM software.
7. Workflow analysis software
Workflow analysis software can automatically pinpoint inefficiencies and track performance KPIs. The software provides a chart to increase visibility and help locate the issues in the workflow.
Explore workflow management tools, such as:
Process analysis techniques
There are various techniques that can be applied to analyze process data. Some of these include:
8. Gap analysis
Gap analysis is a high-level technique that allows analysts to identify the difference between the process performance they target and what they achieve. Gap analysis can highlight redundancies, wasteful activities, lack of management and missing steps.
Gap analysis is a useful technique for process analysts that aim to reorient their process performance and reconnect with their goals.
9. Value-added analysis
This analysis is a broad sorting technique to assess whether each process step meets business or customer needs. Value-added analysis technique investigates all activities in a process flow and then label and sort steps to:
- Define business value-added steps
- Identify real value-added (RVA) steps
- Discover non-value-added (NVA) steps
To remove and modify when they do not meet customer or business needs.
10. Root cause analysis
This technique finds out the core reasons behind the detected problems by estimating probabilities for potential causes. Root cause analysis allows analysts to discover hidden deep issues and develop a remedy accordingly. The technique can investigate:
- Guides and instructions
- Equipment such as devices and tools
- Methods like requesting an order
- Employee activities
- Environment as onsite and offsite spaces.
Some process intelligence tools can automatically employ root cause analysis. Learn more on automated root cause analysis.
11. Observational analysis
This technique shows overlooked steps and absent activities in actual processes. Observational analysis can require:
- Collecting data from interviews, process maps and other documents to observe passively
- Participate in the process and monitor workflow actively.
Analysts can benefit from observational analysis to assess process accuracy.
12. Experience examination analysis
Experience examination requires observing and interviewing longtime employees to capture process knowledge. Experienced employees can help identify:
- Process inefficiencies and potential reasons behind them
- Well-functioning processes and happy paths
Analysts can review their process instructions and update their employee onboarding process based on the results.
13. Predictive analysis
Predictive analysis, also known as simulation analysis, is a technique to predict the impact of changes on process performance. Predictive analysis can help develop and prioritize process improvement strategies.
For example, analysts can:
- Identify areas that can benefit from automation the most
- Decide on change type, like standardization.
Predictive maintenance, predictive process monitoring and predictive process mining are some software that allows analysts to forecast their processes.
14. Impact analysis
Impact analysis is a value metric to assess the impact of interactions between processes, systems and applications.
Digital transformation demands that companies implement new systems and applications. Impact analysis can streamline deploying new tools and systems and decide when to change them by allowing firms to understand their dependencies.
15. Failure mode effects analysis (FMEA):
This technique is a step-by-step approach to locating potential design, process, or service failures. Failure mode effects analysis focuses on the consequences of failures to eliminate such errors in the long run.
Diagrams and charts
Diagrams and charts are broadly employed to visualize or model processes. Some of these visualization techniques can be utilized for process analysis.
16. Flowchart mapping
Process flowcharts map out simple processes as separate process steps in sequential order. The chart illustrates:
- Inputs and outputs, such as materials, end products and services
- People involved
- Decision points
- Time for each step
- Process performance KPIs.
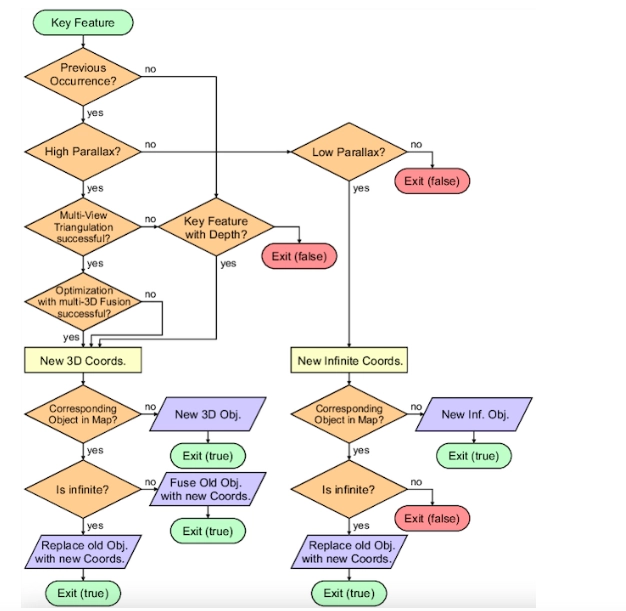
17. Spaghetti diagram
The spaghetti diagram represents processes as a continuous flow of lines. Spaghetti diagrams are useful to trace the path of activities, tasks and resources.
These diagrams can help identify redundancies and opportunities in the given flow. Yet, they can be too complicated and useless for complex processes.
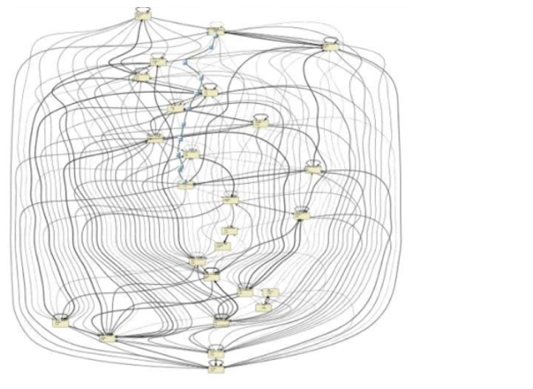
Discover two ways process mining can overcome complicated spaghetti diagrams:
- Use Multi-level Process Mining to Tackle 3 Arduous Challenges.
- What is Object-Centric Process Mining & 5 Reasons to Apply it?
18. Fishbone diagram
Fishbone diagrams show cause-and-effect relationships of an issue in the given process. They are combined with root cause analysis. Fishbone diagrams consider:
- Equipment, such as software and machinery used
- Onsite and offsite environment
- Process documents, policies, rules and procedures
- Employees.
By drilling down these factors, the diagram enables analysts to determine the main reasons behind the problem. For example, Figure 4 below shows potential issues for each aspect.
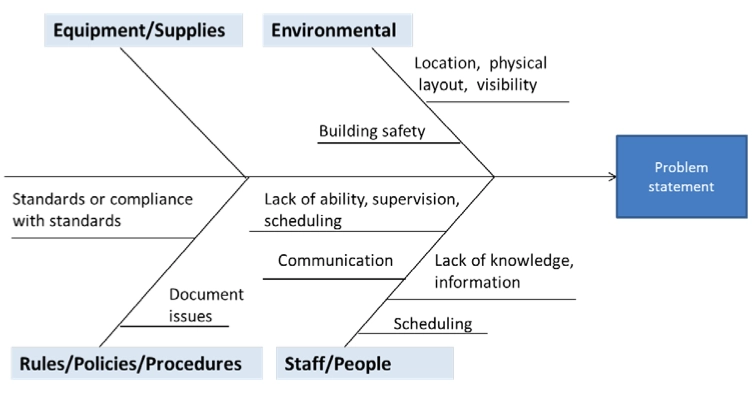
There are some overlapping technologies, solutions and techniques with other process management and process improvement tools. Check out to find out the differences, use cases and real-life examples:
- process improvement methodologies and techniques, such as Kaizen, six sigma or Lean
- Process visualization techniques such as flowchart mapping.
FAQs
How is business process analysis conducted?
Here is a step by step guide:
1. Identify the process for analysis: Select a process with high business value and low complexity. The chosen process should be easier to document and beneficial to optimize.
2. Identify the stakeholders: List all key stakeholders: process owners, team members, clients, and external partners. Invite stakeholders to commit to the analysis process to ensure a successful outcome.
3. Determine the inputs/outputs at each stage: Identify inputs (data or materials) required at each step of the process. Document outputs generated at each stage, which are typically more consistent.
4. Observe and record the process:
– For manual processes: Monitor each step closely, recording metrics like time spent and costs.
– For automated processes: Leverage process analysis software to:
– Capture tasks through task mining tools.
– Collect process logs from systems to automatically record process flows.
– Analyze and visualize the process flow, which provides real-time insights into process performance.
– Document the “happy path” (ideal workflow) and exception paths (unexpected variations).
5.Interview process stakeholders: Gather insights from stakeholders to identify bottlenecks and issues. Use their feedback as valuable input for the analysis.
6.Create a process map: Use gathered data to visually document the process in a map.
– For automated processes: Process mapping tools can automatically generate maps from collected logs, showing each step, inputs, outputs, and common exceptions.
7. Analyze the process: Conduct a detailed review of the process map, maintaining a quantifiable approach. Look for bottlenecks, redundancies, errors, and deviations. Address any issues raised by stakeholders and focus on areas for improvement.
Using process analysis software for automated processes can enhance accuracy, enable quicker insights, and provide a visual overview of process performance.
What are business process analysis tools used for?
Business process analysis tools and techniques aim to understand business processes, analyze, and improve business operations for increased efficiency and productivity.
How often should you do business process analysis?
The frequency of analyzing business processes varies depending on the organization and its needs. However, as a general rule, they should be carried out regularly to ensure continuous improvement.
Which business process analysis tool is best suited for my business?
The choice of process analysis tool largely depends on the specific requirements of your organization, the complexity of your current processes, and the capabilities of your process owners.
What is the difference between business process analysis and data analytics?
The difference is that business process analysis focuses specifically on analyzing and optimizing business processes, while data analytics involves the broader examination and interpretation of data to extract insights and inform decision-making.
Further reading
Learn other process tools and methods used to discover, manage and improve process mining:
- What is Process Technology & 3 Reasons to apply it?
- Process Improvement Technology: 4 Best Tools to know
- Pair Methodology with Technology for Process Improvement
Check out our data-driven comprehensive solutions to compare vendors for:
- Process mining
- Task mining
- DTO
- BPM Software
- Workflow management software
- Low-code/No-code development platform
- Onboarding software
If you have more questions, let us help you:
External Links
- 1. ResearchGate - Temporarily Unavailable.
- 2. ResearchGate - Temporarily Unavailable.
- 3. ”How to Use the Fishbone Tool for Root Cause Analysis.” CMS. Revisited March 3, 2023.
Comments
Your email address will not be published. All fields are required.