LLMs are growing rapidly, but development and fine-tuning remain expensive.1
LLMOps tools help reduce these costs by streamlining LLM management.To better understand the landscape, we’ve also prepared a detailed comparison of LLMOps and MLOps tools to highlight how they differ in capabilities, focus areas, and workflows.
Discover LLMOps tools and compare the top players:
Tool | Evaluation | Cost Tracking | Fine Tuning | Prompt Eng. | Pipeline Cons. | BLEU / ROUGE | Data Storage & Versioning |
---|---|---|---|---|---|---|---|
Deepset AI | ❌ | ❌ | ✅ | ✅ | ✅ | ❌ | ✅ |
Nemo by NVIDIA | ✅ | ❌ | ✅ | ✅ | ❌ | ✅ | ❌ |
Deep Lake | ✅ | ❌ | ❌ | ❌ | ❌ | ❌ | ✅ |
Snorkel AI | ❌ | ❌ | ❌ | ✅ | ✅ | ❌ | ✅ |
ZenML | ✅ | ❌ | ❌ | ❌ | ✅ | ✅ | ❌ |
TrueFoundry | ✅ | ✅ | ✅ | ❌ | ✅ | ✅ | ❌ |
Comet | ✅ | ✅ | ❌ | ❌ | ❌ | ✅ | ❌ |
Lamini AI | ✅ | ✅ | ✅ | ✅ | ✅ | ✅ | ❌ |
Fine-Tuner AI | ✅ | ❌ | ✅ | ✅ | ❌ | ❌ | ✅ |
Sorted by GitHub stars for LLMOps tools. See the extended LLMops and MLOps tools comparison table below for detailed star counts.
A breakdown of each metric is provided below:
Evaluation: Some LLMOps tools include built-in capabilities to assess model outputs based on task-specific criteria, while others depend on external frameworks for more customized or in-depth analysis.
Cost Tracking: Detailed cost analysis and monitoring of resources used during training and inference are either directly supported by tools or achieved through integrations.
Fine Tuning: Some LLMOps tools perform fine-tuning of large language models themselves, whereas others focus on managing or orchestrating the fine-tuning process.
Prompt Engineering: Designing and optimizing prompts is directly handled by some tools, but most provide infrastructure to support this rather than performing it themselves.
Pipeline Construction: Certain tools automate end-to-end LLM workflows—including data preparation, training, and evaluation—while others enable pipeline building through integrations.
BLEU / ROUGE: BLEU and ROUGE are common language evaluation metrics used to assess text quality; some tools support them natively, while others rely on external libraries.
Data Storage & Versioning: Secure storage and version tracking of training data is directly handled by some tools, while others integrate with third-party storage/versioning solutions.
LLMOps Landscape
There are 40+ tools that claim to be LLMOps solutions, which can be evaluated under 6 main categories:
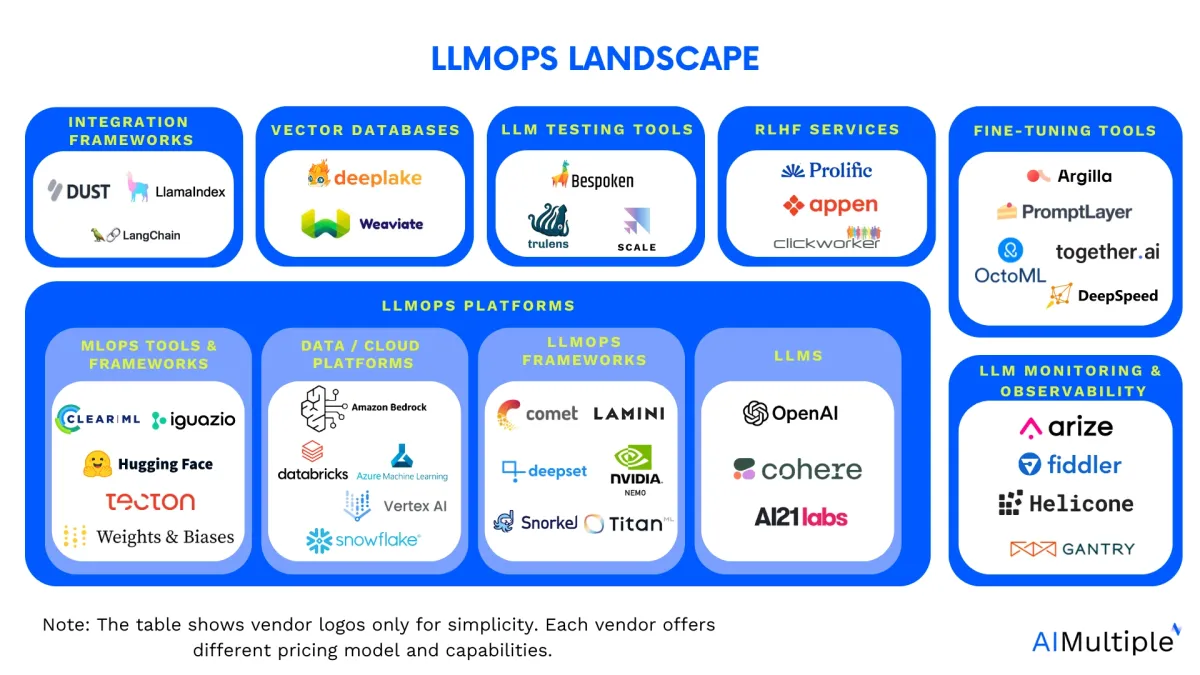
LLMOps Platforms
These are either designed specifically for LLMOps or are MLOps platforms that started offering LLMOps capabilities. They include features that allow carrying out these operations on LLMs:
- Finetuning
- Versioning
- Deploying
These LLM platforms can offer different levels of flexibility and ease of use:
- No-code LLM platforms: Some of these platforms are no-code and low-code, which facilitate LLM adoption. However, these tools typically have limited flexibility.
- Code-first platforms: These platforms target machine learning engineers and data scientists. They tend to offer a higher level of flexibility.
LLMOps platforms can be examined under these categories:
MLOps tools & frameworks
Certain MLOps platforms now come equipped with specialized toolkits tailored for large language model operations (LLMOps).
Machine Learning Operations (MLOps) is the discipline focused on orchestrating the full lifecycle of machine learning, from development through to deployment and maintenance. Since LLMs are also machine learning models, MLOps vendors are naturally expanding into this domain.
Data and cloud platforms
Data or cloud platforms are starting to offer LLMOps capabilities that allow their users to leverage their own data to build and finetune LLMs. For example, Databricks acquired MosaicML for $1.3 billion.2
Cloud platforms
Cloud leaders Amazon, Azure and Google have all launched their LLMOps offering which allows users to deploy models from different providers with ease
LLMOPs frameworks
This category includes tools that exclusively focus on optimizing and managing LLM operations. Here’s a breakdown of the tools and their core LLMOps functions:
Tool | LLMOps Role |
---|---|
Comet | Experiment Tracking & Observability |
ZenML | Pipeline Management & Reproducibility |
Lamini AI | Model Customization Platform |
Deep Lake | Data Storage & Versioning |
Nemo (NVIDIA) | Large-Scale Foundation Model Framework |
Snorkel AI | Programmatic Labeling & Data-Centric AI |
Deepset AI | Retrieval-Augmented Generation (RAG) Framework |
Fine-Tuner AI | Lightweight Tuning & Inference Optimization |
Valohai | CI/CD & Infrastructure Automation for ML/LLM Pipelines |
TrueFoundry | End-to-End LLM Lifecycle Management |
Here is a brief explanation for each tool in alphabetical order:
Comet
Comet streamlines the ML lifecycle, tracking experiments and production models. Suited for large enterprise teams, it offers various deployment strategies. It supports private cloud, hybrid, and on-premise setups.
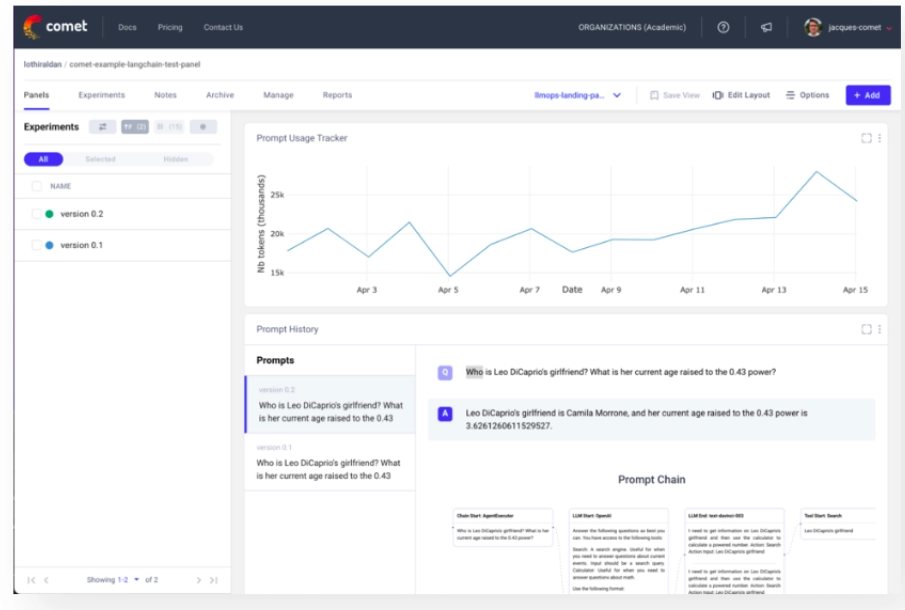
DeepLake
Deep Lake combines the capabilities of Data Lakes and Vector Databases to create, refine, and implement high-quality LLMs and MLOps solutions for businesses. Deep Lake allows users to visualize and manipulate datasets in their browser or Jupyter notebook, swiftly accessing different versions and generating new ones through queries, all compatible with PyTorch and TensorFlow.
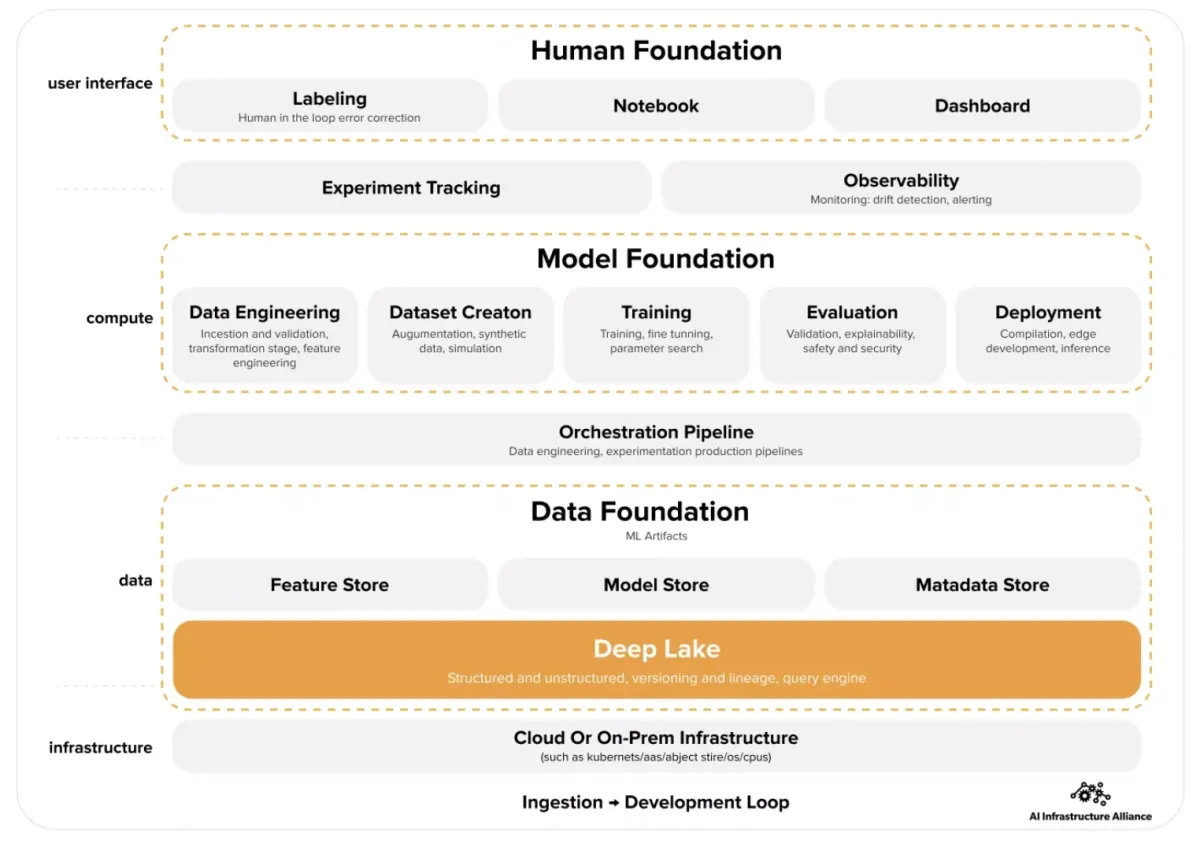
Deepset AI
Deepset AI is a comprehensive platform that allows users to integrate their data with LLMs to build and deploy customized LLM features in their applications. Deepset supports Retrieval-augmented generation (RAG) and Enterprise knowledge search, as well.
Lamini AI
Lamini AI provides an easy method for training LLMs through both prompt-tuning and base model training. Lamini AI users can write custom code, integrate their own data, and host the resulting LLM on their infrastructure.
Nemo by Nvidia
Nvidia offers an end-to-end, cloud-native enterprise framework to develop, customize, and employ generative AI models and LLM applications. The framework can execute various tasks required to train LLMs, such as token classification, prompt learning and question answering.
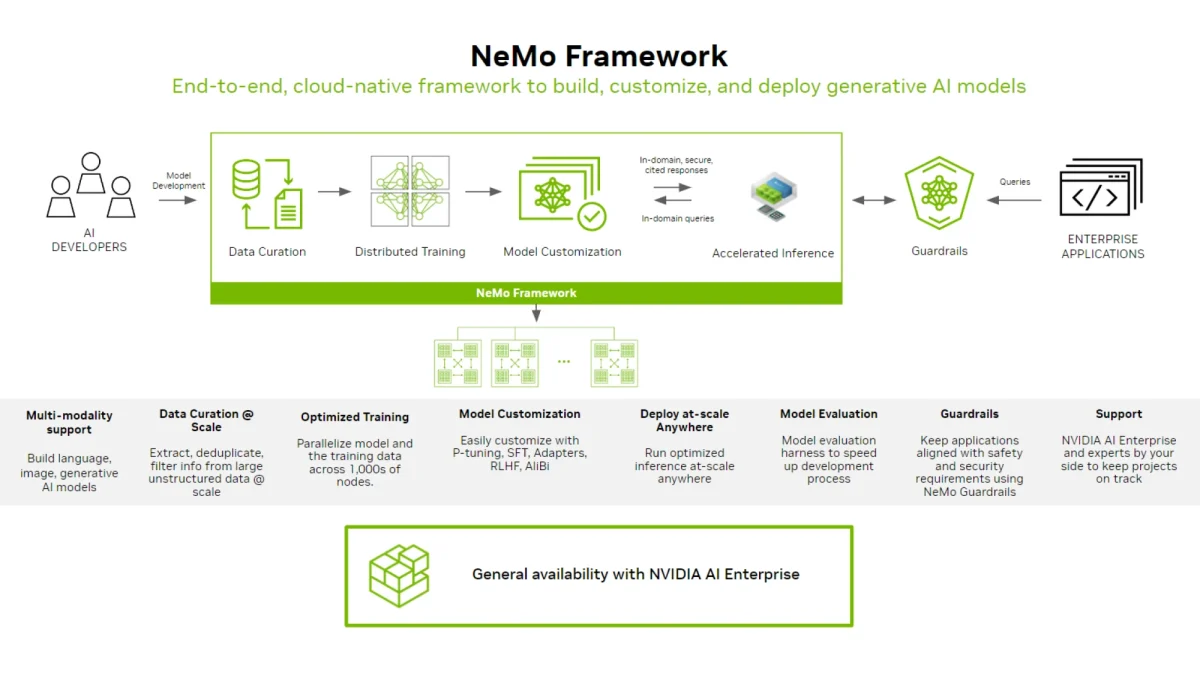
Snorkel AI
Snorkel AI empowers enterprises to construct or customize foundation models (FMs) and large language models (LLMs) to achieve remarkable precision on domain-specific datasets and use cases. Snorkel AI introduces programmatic labelling, enabling data-centric AI development with automated processes.
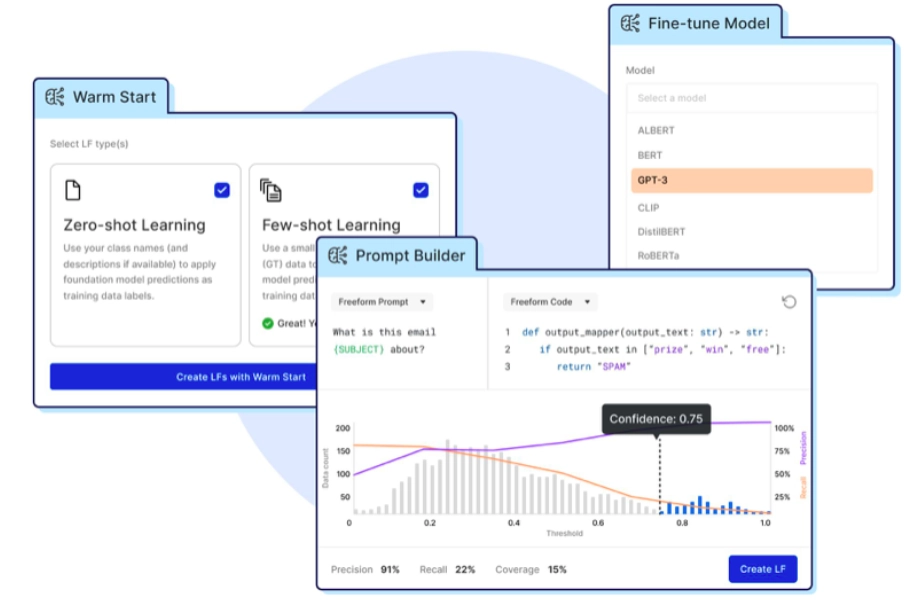
Titan ML
TitanML is an NLP development platform that aims to allow businesses to swiftly build and implement smaller, more economical deployments of large language models. It offers proprietary, automated, efficient fine-tuning and inference optimization techniques. This way, it allows businesses to create and roll out large language models in-house.
TrueFoundry
TrueFoundry is a complete LLMOps platform that simplifies deployment, fine-tuning, and monitoring of large language models. It offers GPU-optimized infrastructure, secure access controls, and built-in model and prompt management. With observability and safety features, it’s designed for scalable enterprise use.
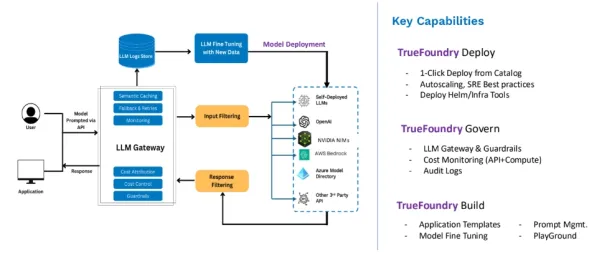
Valohai
Valohai streamlines MLOps and LLMs, automating data extraction to model deployment. It can store models, experiments, and artefacts, making monitoring and deployment easier. Valohai creates an efficient workflow from code to deployment, supporting notebooks, scripts, and Git projects.
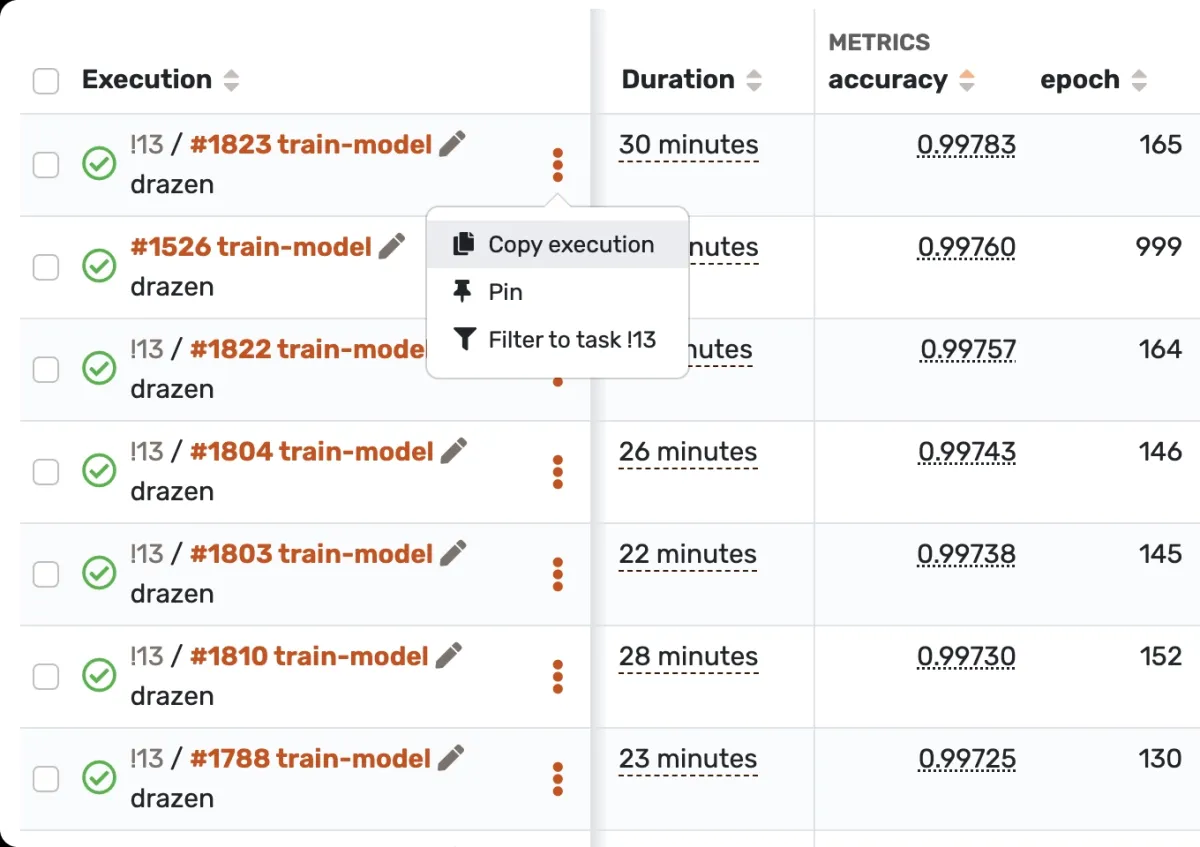
Zen ML
ZenML primarily focuses on machine learning operations (MLOps) and the management of the machine learning workflow, including data preparation, experimentation and model deployment.
LLMs
Some LLM providers, especially OpenAI, are also providing LLMOps capabilities to fine-tune, integrate and deploy their models.
Integration frameworks
These tools are built to facilitate developing LLM applications such as document analyzers, code analyzers, chatbots etc.
Vector databases (VD)
VDs store high-dimensional data vectors, such as patient data covering symptoms, blood test results, behaviors, and general health. Some VD software like DeepLake can facilitate LLM operations.
Fine-tuning tools
Fine-tuning tools are frameworks, or platforms for fine-tuning pre-trained models. These tools provide a streamlined workflow to modify, retrain, and optimize pre-trained models for natural language processing, computer vision, and more tasks.
Some libraries are also designed for fine-tuning, such as Hugging Face Transformers, PyTorch, and TensorFlow.
RLHF tools
RLHF, short for reinforcement learning from human feedback, enables AI systems to refine their decisions by incorporating guidance provided by humans. In reinforcement learning, an agent improves its behavior through trial and error, guided by feedback from the environment in the form of rewards or punishments.
In contrast, RLHF tools (e.g. Clickworker or Appen) include human feedback in the learning loop. RLHF can be useful to:
- Enhance LLM fine-tuning by large data labeling
- Implement AI governance by reducing biases in LLM responses and moderating content
- Customize model
- Improve contextual understanding.
LLM testing tools
LLM testing tools evaluate and assess LLMs by testing model performance, capabilities, and potential biases in various language-related tasks and applications, such as natural language understanding and generation. Testing tools may include:
- Testing frameworks
- Benchmark datasets
- Evaluation metrics.
LLM monitoring and observability
LLM monitoring and observability tools ensure their proper functioning, user safety, and brand protection. LLM monitoring includes activities like:
- Functional monitoring: Keeping track of factors like response time, token usage, number of requests, costs and error rates.
- Prompt monitoring: Checking user inputs and prompts to evaluate toxic content in responses, measure embedding distances, and identify malicious prompt injections.
- Response monitoring: Analyzing to discover hallucinatory behavior, topic divergence, tone and sentiment in the responses.
Benchmark: TrueFoundry vs Amazon SageMaker vs Manual (no LLMOps tools)
We benchmarked TrueFoundry,Amazon SageMaker, and a manual setup to evaluate the real-world benefits of LLMOps tools. Using the same model, dataset, and hardware, we measured training and evaluation times.
Metric | TrueFoundry | SageMaker | Manual |
---|---|---|---|
Training Time (sec) | 569 | 548 | 2572 |
Evaluation Time (sec) | 40 | 42 | 174 |
Infra Model | Self-hosted on K8s | AWS-managed only | Manual Setup |
Observability | Full: UI + logs | Basic logs only | Manual Setup |
Support SLA | 24/7 Slack + AM | 1h–24h (tiered) | None |
AWS Integration | Moderate | Native + deep | Manual CLI/SDK |
LLM Flexibility | Easy self-hosting of open-source LLMs with gateway routing | AWS Bedrock locked; external model hosting limited | Manual setup, no built-in LLM hosting |
Built-in Tools | Advanced observability, debugging, Kafka integration | Built-in AutoML, data labeling, feature engineering | Manual tooling and setup |
Both platforms reduced training from 2,572 seconds to under 570, and evaluation from 174 seconds to around 40. While SageMaker was slightly faster in training and TrueFoundry in evaluation, the overall difference was negligible—both delivered major improvements over manual setup.
You can see our methodology here.
Choosing the right infrastructure for LLMOps depends not only on speed but also on cost, automation, and integration quality. SageMaker offers deep AWS integration, TrueFoundry provides fast deployment with high cost efficiency, while manual setups are flexible but usually slower.
Tools for secure and complaint LLMs
Some LLMOps integrate with AI governance and LLM security technologies to ensure safe, unbiased, and ethical LLM deployment and operation. Check out more on these:
- Compare Top 25 AI Governance Tools: A Vendor Benchmark
- Compare 20 LLM Security Tools & Open-Source Frameworks
Which LLMOps tool is the best choice for your business?
We now provide relatively generic recommendations on choosing these tools. We will make these more specific as we explore LLMOps platforms in more detail and as the market matures.
Here are a few steps you must complete in your selection process:
- Define goals: Clearly outline your business goals to establish a solid foundation for your LLMOps tool selection process. For example, if your goal requires training a model from scratch vs fine-tuning an existing model, this will have important implications to your LLMOps stack.
- Define requirements: Based on your goal, certain requirements will become more important. For example, if you aim to enable business users to use LLMs, you may want to include no code in your list of requirements.
- Prepare a shortlist: Consider user reviews and feedback to gain insights into real-world experiences with different LLMOps tools. Rely on this market data to prepare a shortlist.
- Compare functionality: Utilize free trials and demos provided by various LLMOps tools to compare their features and functionalities firsthand.
What is LLMOps?
LLMOPS stands for Large Language Model Operations, denoting a strategy or system to automate and refine the AI development pipeline through the utilization of expansive language models. LLMOPs tools facilitate the continuous integration of these substantial language models as the underlying backend or driving force for AI applications.
Key components of LLMOps:
- Selection of a foundation model: A starting point dictates subsequent refinements and fine-tuning to make foundation models cater to specific application domains.
- Data management: Managing extensive volumes of data becomes pivotal for accurate language model operation.
- Deployment and monitoring model: Ensuring the efficient deployment of language models and their continuous monitoring ensures consistent performance.
- Prompt engineering: Creating effective prompt templates for improved model performance.
- Model monitoring: Continuous tracking of model outcomes, detection of accuracy degradation, and addressing model drift.
- Evaluation and benchmarking: Rigorous evaluation of refined models against standardized benchmarks helps gauge the effectiveness of language models.
- Model fine-tuning: Fine-tuning LLMs to specific tasks and refining models for optimal performance.
How Is LLMOps Different Than MLOps?
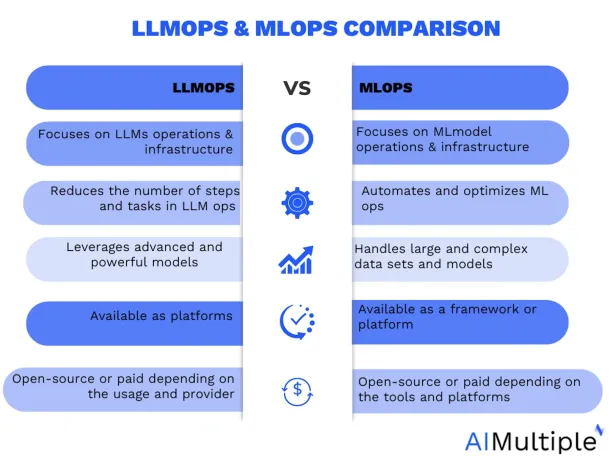
LLMOps is specialized and centred around utilising large language models. At the same time, MLOps has a broader scope encompassing various machine learning models and techniques. In this sense, LLMOps are known as MLOps for LLMs. Therefore, these two diverge in their specific focus on foundational models and methodologies:
Aspect | LLMOps | MLOps |
---|---|---|
Computational resources | High compute, GPUs | Less compute |
Transfer learning | Fine-tuning | From scratch |
Human feedback | RLHF | Less used |
Hyperparameter tuning | Cost & performance | Accuracy focus |
Performance metrics | BLEU, ROUGE | Accuracy, AUC, F1 |
Prompt engineering | Critical | Not relevant |
Constructing pipelines | Chained LLM calls | Automation focus |
Computational resources : NVIDIA L40 vs L40S
Training and deploying large language models require significant computational power, often relying on specialized hardware such as GPUs to handle large datasets efficiently. Access to these resources is essential for effective model training and inference. Additionally, managing inference costs through techniques like model compression and distillation helps reduce resource consumption without sacrificing performance.
For example, NVIDIA’s L40 and L40S GPUs represent two powerful options designed to meet these demanding computational needs. The NVIDIA L40 is a versatile GPU aimed at general-purpose graphics and compute workloads, delivering strong performance for a wide range of applications. In contrast, the NVIDIA L40S is optimized specifically for AI and deep learning tasks, featuring enhanced tensor processing capabilities that accelerate neural network training and inference. This makes the L40S particularly well-suited for large-scale language models, offering higher throughput and efficiency for AI workloads.
Transfer learning
Unlike conventional ML models built from the ground up, LLMs frequently commence with a base model, fine-tuned with fresh data to optimize performance for specific domains. This fine-tuning facilitates state-of-the-art outcomes for particular applications while utilizing less data and computational resources.
Human feedback
Advancements in training large language models are attributed to reinforcement learning from human feedback (RLHF). Given the open-ended nature of LLM tasks, human input from end users holds considerable value for evaluating model performance. Integrating this feedback loop within LLMOps pipelines simplifies assessment and gathers data for future model refinement.
Hyperparameter tuning
While conventional ML involves hyperparameter tuning primarily to enhance accuracy, LLMs introduce an added dimension of reducing training and inference costs. Adjusting parameters like batch sizes and learning rates can substantially influence training speed and cost. Consequently, meticulous tuning process tracking and optimisation remain pertinent for both classical ML models and LLMs, albeit with varying focuses.
Performance metrics
Traditional ML models rely on well-defined metrics such as accuracy, AUC, and F1 score, which are relatively straightforward to compute. In contrast, evaluating LLMs entails an array of distinct standard metrics and scoring systems—like bilingual evaluation understudy (BLEU) and Recall-Oriented Understudy for Gisting Evaluation (ROUGE)—that necessitate specialized attention during implementation.
Prompt engineering
Models that follow instructions can handle intricate prompts or instruction sets. Crafting these prompt templates is critical for securing accurate and dependable responses from LLMs. Effective, prompt engineering mitigates the risks of model hallucination, prompt manipulation, data leakage, and security vulnerabilities.
Constructing LLM pipelines
LLM pipelines string together multiple LLM invocations and may interface with external systems such as vector databases or web searches. These pipelines empower LLMs to tackle intricate tasks like knowledge base Q&A or responding to user queries based on a document set. In LLM application development, the emphasis often shifts towards constructing and optimizing these pipelines instead of creating novel LLMs.
Additionally, large multimodal models extend these capabilities by incorporating diverse data types, such as images and text, enhancing the flexibility and utility of LLM pipelines.
Here is a categorized overview of key tools across the LLMOps and MLOps landscape:
Dust
LlamaIndex
Langchain
Deep Lake
Weaviate
Tools | Type | GitHub stars |
---|---|---|
Dust | Integration framework | 997 |
LlamaIndex | Integration framework | 37.4k |
Langchain | Integration framework | 96.5k |
Deep Lake | Vector databases | 8.3k |
Weaviate | Vector databases | 11.8k |
Bespoken | LLM testing tools | Not open source |
Trulens | LLM testing tools | 2.2k |
Scale | LLM testing tools | Not open source |
Prolific | RLHF services | Not open source |
Appen | RLHF services | Not open source |
Clickworker | RLHF services | Not open source |
Argilla | Fine-tuning tools | 4.1k |
PromptLayer | Fine-tuning tools | 532 |
Octo ML | Fine-tuning tools | Not open source |
Together AI | Fine-tuning tools | Not open source |
DeepSpeed | Fine-tuning tools | 36k |
Phoenix by Arize | LLM monitoring & observability | 4.3k |
Fiddler | LLM monitoring & observability | Not open source |
Helicone | LLM monitoring & observability | 2.7k |
Gantry | LLM monitoring & observability | 1k |
Clear ML | MLOPs tools & frameworks | 5.8k |
Ignazio | MLOPs tools & frameworks | 5.3k |
HuggingFace | MLOPs tools & frameworks | 137k |
Tecton | MLOPs tools & frameworks | Not open source |
Weights & Biases | MLOPs tools & frameworks | 9.3k |
Amazon Bedrock | Data / cloud platforms | Not open source |
DataBricks | Data / cloud platforms | Not open source |
Azure ML | Data / cloud platforms | Not open source |
Vertex AI | Data / cloud platforms | Not open source |
Snowflake | Data / cloud platforms | Not open source |
Nemo by Nvidia | LLMOps frameworks | 12.5k |
Deep Lake | LLMOps frameworks | 8.3k |
Fine-Tuner AI | LLMOps frameworks | 1.5k |
Snorkel AI | LLMOps frameworks | 5.8k |
Zen ML | LLMOps frameworks | 4.3k |
Lamini AI | LLMOps frameworks | 2.5k |
Comet | LLMOps frameworks | 3.8k |
TrueFoundry | LLMOps Frameworks | 4.1k |
Titan ML | LLMOps frameworks | Not open source |
Haystack by Deepset AI | LLMOps frameworks | 18.3k |
Valohai | LLMOps frameworks | Not open source |
OpenAI | LLMs | Not open source |
Anthropic Claude | LLMs | Not open source |
Cohere | LLMs | Not open source |
AI21 Labs | LLMs | Not open source |
LLMOPS vs MLOPS: Pros and Cons
While deciding which one is the best practice for your business, it is important to consider benefits and drawbacks of each technology. Let’s dive deeper into the pros and cons of both LLMOps and MLOps to compare them better:
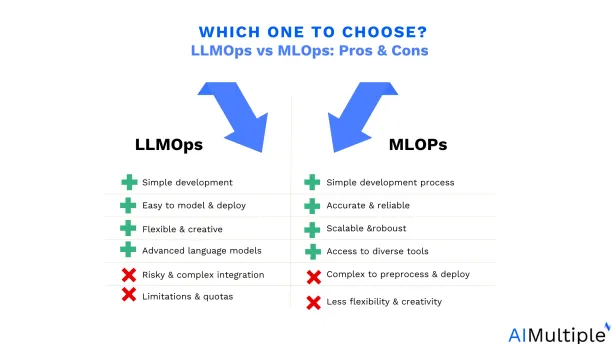
LLMOPS Pros
- Simple development: LLMOPS simplifies AI development significantly compared to MLOPS. Tedious tasks like data collection, preprocessing, and labeling become obsolete, streamlining the process.
- Easy to model and deploy: The complexities of model construction, testing, and fine-tuning are circumvented in LLMOPS, enabling quicker development cycles. Also, deploying, monitoring, and enhancing models are made hassle-free. You can leverage expansive language models directly as the engine for your AI applications.
- Flexible and creative: LLMOPS offers greater creative latitude due to the diverse applications of large language models. These models excel in text generation, summarization, translation, sentiment analysis, question answering, and beyond.
- Advanced language models: By utilizing advanced models like GPT-3, Turing-NLG, and BERT, LLMOPS enables you to harness the power of billions or trillions of parameters, delivering natural and coherent text generation across various language tasks.
LLMOPS Cons
- Limitations and quotas: LLMOPS comes with constraints such as token limits, request quotas, response times, and output length, affecting its operational scope.
- Risky and complex integration: As LLMOPS relies on models in beta stages, potential bugs and errors could surface, introducing an element of risk and unpredictability. Also, Integrating large language models as APIs requires technical skills and understanding. Scripting and tool utilization become integral components, adding to the complexity.
MLOPS Pros
- Simple development process: MLOPS streamlines the entire AI development process, from data collection and preprocessing to deployment and monitoring.
- Accurate and reliable: MLOPS ensures the accuracy and reliability of AI applications through standardized data validation, security measures, and governance practices.
- Scalable and robust: MLOPS empowers AI applications to handle large, complex data sets and models seamlessly, scaling according to traffic and load demands.
- Access to diverse tools: MLOPS provides access to many tools and platforms like cloud computing, distributed computing, and edge computing, enhancing development capabilities.
MLOPS Cons
- Complex to deploy: MLOPS introduces complexity, demanding time and effort across various tasks like data collection, preprocessing, deployment, and monitoring.
- Less flexible and creative: While versatile, MLOPS confines the application of machine learning to specific purposes, often employing less sophisticated models than expansive language models.
Which one to choose?
Choosing between MLOps and LLMOps depends on your specific goals, background, and the nature of the projects you’re working on. Here are some instructions to help you make an informed decision:
1. Understand your goals: Define your primary objectives by asking whether you focus on deploying machine learning models efficiently (MLOps) or working with large language models like GPT-3 (LLMOps).
2. Project requirements: Consider the nature of your projects by checking if you primarily deal with text and language-related tasks or with a wider range of machine learning models. If your project heavily relies on natural language processing and understanding, LLMOps is more relevant.
3. Resources and infrastructure: Think about the resources and infrastructure you have access to. MLOps may involve setting up infrastructure for model deployment and monitoring. LLMOps may require significant computing resources due to the computational demands of large language models.
4. Evaluate expertise and team composition by determining if your expertise lies in machine learning, software development, or both. Do you have specialists in machine learning, DevOps, or both? MLOps requires collaboration between data scientists, software engineers, and DevOps professionals for deploying and managing machine learning models. LLMOps deals with deploying, fine-tuning, and maintaining large language models as part of real-world software systems.
5. Industry and use cases: Explore the industry you’re in and the specific use cases you’re addressing. Some industries may heavily favour one approach over the other. LLMOps might be more relevant in industries like content generation, chatbots, and virtual assistants.
6. Hybrid approach: Remember that there’s no strict division between MLOps and LLMOps. Some projects may require a combination of both systems.
Benchmark methodology
We benchmarked the training and evaluation times of a DistilBERT-based sentiment classification model across three environments: a manual setup (CPU-only), TrueFoundry, and Amazon SageMaker. To ensure consistency, we used the same codebase, pretrained model (distilbert-base-uncased), and the first 5,000 samples from the Amazon Reviews dataset across all runs.
The dataset was filtered to include ratings from 1 to 5, relabeled into five classes (0–4), and split into stratified 80/20 training and validation sets. Tokenization was performed with a fixed maximum sequence length of 128.
The model was trained for one epoch using identical batch sizes (16 for training, 32 for evaluation). Both TrueFoundry and SageMaker used the same GPU instance type, while the manual setup was intentionally run on CPU to reflect a typical local or non-specialized environment.
This setup highlights not only the platform-level optimizations provided by modern LLMOps tools, but also the substantial performance benefit of seamless GPU access. The benchmark illustrates how using managed platforms like TrueFoundry and SageMaker can reduce training and evaluation time compared to running the same code manually on CPU, especially in real-world, resource-limited scenarios.
FAQ
What are LLMOps benefits?
LLMOps delivers significant advantages to machine learning projects leveraging large language models:
1.) Increased Accuracy: Ensuring high-quality data for training and reliable deployment enhances model accuracy.
2.) Reduced Latency: Efficient deployment strategies lead to reduced latency in LLMs, enabling faster data retrieval.
3.) Fairness Promotion: Promoting fairness in AI means actively reducing AI biases in algorithms to uphold equity and prevent AI ethics violations.
LLMOps challenges & solutions
Challenges in large language model operations require robust solutions to maintain optimal performance:
1.) Data Management Challenges: Handling vast datasets and sensitive data necessitates efficient data collection and versioning.
2.) Model Monitoring Solutions: Implementing model monitoring tools to track model outcomes, detect accuracy degradation, and address model drift.
3.) Scalable Deployment: Deploying scalable infrastructure and utilizing cloud-native technologies to meet computational power requirements.
4.) Optimizing Models: Employing model compression techniques and refining models to enhance overall efficiency.
LLMOps tools are pivotal in overcoming challenges and delivering higher quality models in the dynamic landscape of large language models.
Why do we need LLMOps?
The necessity for LLMOps arises from the potential of large language models in revolutionizing AI development. While these models possess tremendous capabilities, effectively integrating them requires sophisticated strategies to handle complexity, promote innovation, and ensure ethical usage.
Real-World Use Cases of LLMOps
In practical applications, LLMOps is shaping various industries:
Content Generation: Leveraging language models to automate content creation, including summarization, sentiment analysis, and more.
Customer Support: Enhancing chatbots and virtual assistants with the prowess of language models.
Data Analysis: Extracting insights from textual data, enriching decision-making processes.
Further reading
Explore more on LLMs, MLOps and AIOps by checking out our articles:
- MLOps Tools & Platforms Landscape: In-Depth Guide
- 15 Best AiOps Platforms: Streamline IT Ops with AI
- ChatGPT AIOps in IT Automation: 8 Powerful Examples
If you still have questions about LLMOps tools and landscape, we would like to help:
External sources
- 1. “The CEO’s Roadmap on Generative AI“
- 2. Databricks Signs Definitive Agreement to Acquire MosaicML, a Leading Generative AI Platform - Databricks.
- 3. Open-Source LLM Evaluation Platform | Opik by Comet.
- 4. Introducing Deep Lake, the Data Lake for Deep Learning.
- 5. NVIDIA NeMo Framework - NVIDIA Docs. NVIDIA Docs
- 6. The Snorkel AI Blog | Home. Snorkel AI
- 7. Valohai | The Scalable MLOps Platform.
Comments
Your email address will not be published. All fields are required.