As the number of consumers increases and users’ data accumulates daily, data explosion is no surprise. Companies get help from data collection and analytics to catch up on their sales, customer insights, or brand reputation. However, even though voice data is the most direct feedback businesses receive from customers, they usually overlook its importance.
To better understand how customers evaluate products & services, explore how to analyze the sentiment in audio files and the top three methods companies can implement:
What is audio sentiment analysis?
Traditional sentiment analysis methods mainly rely on written texts such as reviews, feedback, surveys, etc. However, as human language is complex, nuances such as irony, sarcasm, or intentions are not always easily understood in the written content.
The acoustic tone in audio files carries richer information and gives better insights into the sentiments. Sentiment information can be gathered from various voice characteristics, such as
- pitch
- loudness
- one of voice
- other frequency-related measures
Figure 1. Raw waveform plots for different emotional states using the same sentence
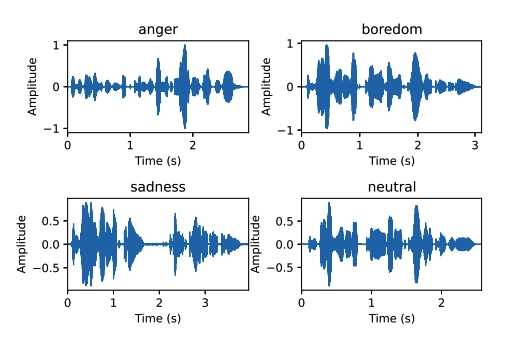
Source: Emo-DB1
So, emotions can be better recognized by combining speech tone and written content analysis than by considering only written feedback.
In recent years, companies started implementing audio sentiment analysis methods to understand their customers’ sentiments better and provide them with a better experience.
To learn more about sentiment analysis, you can check our comprehensive article.
To avoid premature investments into audio sentiment analysis, we have curated this article so adopters and developers can familiarize themselves with the technology, how it works, and the methods to achieve it.
How does audio sentiment analysis work?
Figure 2. A simplified comparison of written content and multimodal (text + audio) sentiment analysis
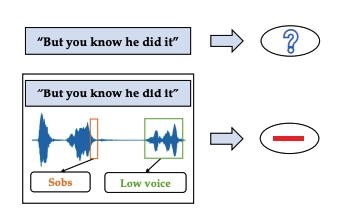
Source: CM-BERT: Cross-Modal BERT for Text-Audio Sentiment Analysis.2
7 methods of conducting audio sentiment analysis
There are three main methods of conducting audio sentiment analysis.
1- Automatic speech recognition (ASR)
Figure 3. An example of how ASR works
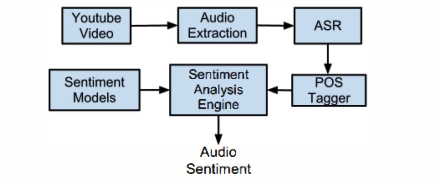
Source: Sentiment extraction from natural audio streams3
Process: ASR transcribes spoken sentences into text using speech recognition. The transcribed text is then analyzed for sentiment using natural language processing (NLP) techniques.
Example: In call centers, ASR can transcribe customer conversations, allowing sentiment analysis models to determine the overall sentiment of the interaction.
2- WaveNet (Raw audio waveform analysis)
Process: WaveNet analyzes raw audio waveforms directly to extract audio features using deep neural networks. This method does not require audio transcription and can capture intricate details in the audio signal. It is a probabilistic method that offers state-of-art results with a multimodal (text+audio) dataset.
Example: WaveNet can detect different emotions from the tone and pitch of the audio, providing a good representation of the speaker’s emotional state.
3- Crossmodal bidirectional encoder representations from transformers (CM-BERT)
Figure 4. The architecture of the CM-BERT network
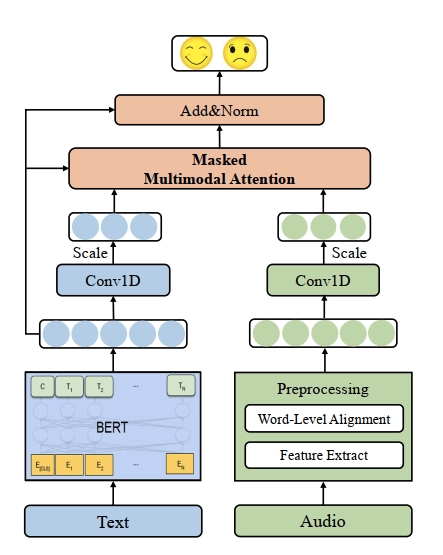
Source: CM-BERT: Cross-Modal BERT for Text-Audio Sentiment Analysis.4
Process: The CM-BERT approach relies on the interaction between text and audio and dynamically adjusts the weight of words by comparing the information from different modalities. It uses machine learning models to analyze both the audio signal and its transcription, leveraging the strengths of both modalities.
Example: In a project analyzing audio recordings from podcasts, CM-BERT can provide insights into the sentiment expressed in both the spoken words and the audio features.
You can also check our article on sentiment analysis datasets to train algorithms.
4- Mel-Frequency cepstral coefficients (MFCCs)
Process: MFCCs are used to represent the short-term power spectrum of sound. They are extracted from audio recordings and used as features for sentiment analysis models.
Example: By analyzing MFCCs, machine learning models can recognize different emotional states in audio files, such as happiness, sadness, or anger.
5- Prosodic features analysis
Process: This method analyzes prosodic features like intonation, stress, and rhythm in speech. These features are crucial for understanding the emotional tone in audio recordings.
Example: Prosodic features analysis can be used in customer service interactions to identify stress or frustration in a customer’s voice, helping improve the user interface and response strategies.
6- Deep neural networks (DNNs)
Process: DNNs can be trained on large datasets of audio recordings to recognize patterns and classify sentiments. They are capable of learning complex representations of audio data.
Example: DNNs can be employed in sentiment analysis projects where high accuracy is required, such as in social media audio posts to gauge public opinion.
7- Recurrent neural networks (RNNs) and long short-term memory (LSTM) networks
Figure 5. Recurrent neural networks with two hidden layers
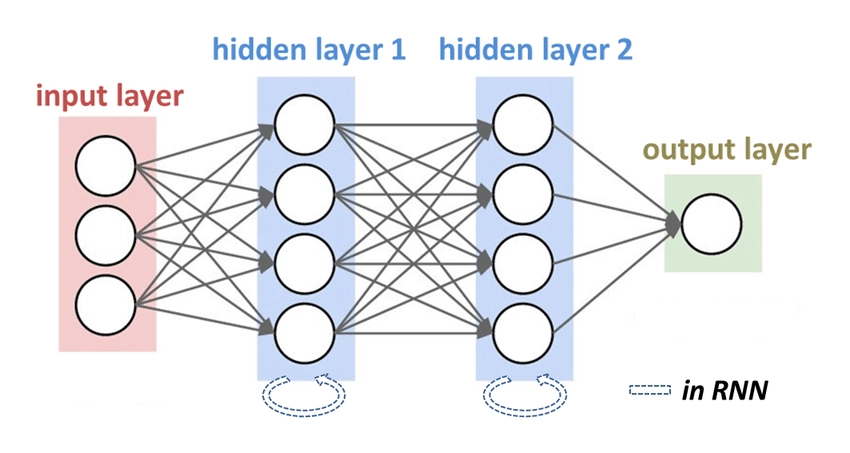
Source: Classification and prediction of wave chaotic systems with machine learning techniques.5
Process: RNNs and LSTMs are designed to handle sequential data, making them suitable for analyzing temporal dependencies in audio signals. They can capture the progression of emotions over time.
Example: In analyzing long audio recordings like interviews or speeches, RNNs and LSTMs can track the changes in sentiment throughout the entire audio file.
You can also check our article on sentiment analysis datasets to train algorithms.
Top 8 applications of audio sentiment analysis
Audio sentiment analysis has a wide range of applications in various fields, enhancing processes and providing valuable insights across industries.
1- Call centers
In call centers, audio sentiment analysis is used to analyze customer interactions. By performing sentiment analysis on audio recordings, companies can determine the sentiment expressed during calls, whether positive, negative, or neutral. This information can help improve customer service by:
- Identifying issues: Detecting negative sentiments early allows call center agents to address customer concerns more effectively.
- Training purposes: Understanding the emotional states of customers during calls can be used to train agents, enhancing their ability to handle different emotions.
- Quality Assurance: Sentiment analysis results can be used to monitor and maintain the quality of service, ensuring consistent customer satisfaction.
2- Emotion recognition
Detecting different emotions in audio recordings can significantly enhance user interfaces and create more empathetic AI systems. Emotion recognition through audio sentiment analysis involves:
- Personalized experiences: Tailoring responses based on the detected emotions to provide a more personalized and engaging user experience.
- Mental health applications: Monitoring emotional states can aid in mental health applications by recognizing signs of stress, anxiety, or depression in audio recordings.
- Virtual assistants: Improving virtual assistants’ interactions by enabling them to respond more appropriately to the user’s emotional tone.
3- Market research
In market research, audio sentiment analysis of audio files from focus groups or customer feedback can provide valuable insights. By analyzing sentiments in spoken responses, companies can:
- Understand consumer preferences: Gain insights into customer opinions about products or services, helping businesses make informed decisions.
- Product development: Use sentiment data to guide the development and improvement of products based on customer feedback.
- Brand perception: Monitor and analyze public sentiment towards a brand, enabling companies to adjust their strategies accordingly.
4- Social media monitoring
Audio sentiment analysis can also be applied to audio files from podcasts or video content shared on social media platforms. This application helps in:
- Public opinion analysis: Analyzing sentiments in spoken content to gauge public opinion on various topics.
- Content strategy: Influencing content creation strategies by understanding the audience’s emotional reactions to different types of content.
- Trend analysis: Identifying emerging trends and sentiments in social media conversations, allowing companies to stay ahead in their marketing efforts.
5- Healthcare
In the healthcare sector, audio sentiment analysis can be applied to patient-doctor interactions, telemedicine consultations, and patient feedback. This can lead to:
- Enhanced patient care: Understanding patient emotions can help healthcare providers offer more empathetic and tailored care.
- Early detection of conditions: Recognizing changes in a patient’s emotional state can assist in the early detection of mental health issues or other conditions.
- Patient satisfaction: Analyzing patient feedback to improve the quality of healthcare services and ensure patient satisfaction.
6- Education
In educational settings, audio sentiment analysis can be used to analyze student interactions, teacher feedback, and classroom discussions. This can support:
- Student engagement: Understanding students’ emotional responses can help educators adjust their teaching methods to keep students engaged.
- Performance monitoring: Monitoring sentiment in student feedback can provide insights into the effectiveness of educational programs and teaching strategies.
- Emotional support: Identifying students who may need additional emotional support, enabling timely intervention.
7- Entertainment Industry
The entertainment industry can leverage audio sentiment analysis to analyze audience reactions to movies, music, and other media content. This can lead to:
- Content improvement: Using sentiment analysis results to improve scripts, dialogues, and overall content based on audience reactions.
- Marketing strategies: Tailoring marketing campaigns to resonate better with the audience’s emotional responses.
- Audience engagement: Creating more engaging and emotionally resonant content by understanding audience sentiments.
8- Human Resources
In human resources, audio sentiment analysis can be applied to employee feedback, interviews, and performance reviews. This can enhance:
- Employee satisfaction: Analyzing sentiments in employee feedback to improve workplace conditions and address concerns.
- Recruitment processes: Understanding candidates’ emotional responses during interviews to make better hiring decisions.
- Performance management: Using sentiment data to support performance reviews and provide constructive feedback.
Further reading on sentiment analysis
- Top 7 Sentiment Analysis Tools
- Sentiment Analysis Services Benchmarking
- Top 3 MonkeyLearn Alternatives for Sentiment Analysis
For those interested, here is our data-driven list of sentiment analysis services.
If you need any assistance, do not hesitate to contact us:
External Links
- 1. Berlin Database of Emotional Speech. Emodb. Accessed: 6/August/2024.
- 2. Kaicheng Yang, Hua Xu, and Kai Gao. 2020. CM-BERT: Cross-Modal BERT for Text-Audio Sentiment Analysis. In Proceedings of the 28th ACM International Conference on Multimedia (MM ’20). Association for Computing Machinery, New York, NY, USA, 521–528.
- 3. L. Kaushik, A. Sangwan and J. H. L. Hansen, “Sentiment extraction from natural audio streams,” 2013 IEEE International Conference on Acoustics, Speech and Signal Processing, Vancouver, BC, Canada, 2013, pp. 8485-8489.
- 4. Kaicheng Yang, Hua Xu, and Kai Gao. 2020. CM-BERT: Cross-Modal BERT for Text-Audio Sentiment Analysis. In Proceedings of the 28th ACM International Conference on Multimedia (MM ’20). Association for Computing Machinery, New York, NY, USA, 521–528.
- 5. Ma, S., Xiao, B., Hong, R., Addissie, B., Drikas, Z., Antonsen, T., … & Anlage, S. (2019). Classification and prediction of wave chaotic systems with machine learning techniques. arXiv preprint arXiv:1908.04716.
Comments
Your email address will not be published. All fields are required.