AI is integral to Alphabet and Google is no exception:
We are now witnessing a new shift in computing: the move from a mobile-first to an AI-first world.
Sundar Pichai @ blog.google
Current products
From smartphone assistants to image recognition and translation, a myriad of AI functionality hides within google apps that you daily use. We mapped this functionality leveraging Smart Faktory’s Google strategy framework.
- Google the search engine is powered by AI: According to Wired’s Cade Metz; Google’s search engine was always driven by algorithms that automatically generate a response to each query. But these algorithms amounted to a set of definite rules. Google engineers could readily change and refine these rules. And unlike neural nets, these algorithms didn’t learn on their own. But now, Google has incorporated deep learning into its search engine. And with its head of AI taking over search, the company seems to believe this is the way forward.
- Google Ads and Doubleclick both incorporate Smart Bidding which is a machine learning powered automated bidding system.
- Google Maps’ Driving Mode estimates where you are headed and helps you navigate without any commands.
- Youtube Safe Content uses machine learning techniques to ensure that brands are not displayed next to offensive content.
- Google Photos suggesting which photos you should share with friends.
- Gmail Smart Reply suggesting replies that match your style and the email you received.
- Google Drive Smart Scheduling suggests meeting schedules based on user’s existing schedule and habits.
- Google Calendar Quick Access feature predicts which files will be used improving performance and user experience.
- Nest Cam Outdoor leveraged machine learning to set up an automated outdoor security camera.
- Google Translate uses an artificial neural network called Google Neural Machine Translation (GNMT) to increase fluency and accuracy of translations.
- Google Chrome uses AI to
- present short and highly related parts of a video while searching for something in Google Search.
- analyze the images on a website and plays an audio description or the alt text(when available) for people who are blind or have low vision.
- Google News uses AI to understand the people, places and things involved in a story as it evolves, organize them based on how they relate to one another as explained in Google Blog.
- Google Assistant is a voice assistant for smart phones or wearables that can search online your flight status or the weather when you get there. Touch and hold the Home button and find your Google Photos, access your music playlists and more. Both Siri and Google Assistant do a decent enough job of finding restaurants, bars, and other kind of businesses nearby, but Google’s app came out on top in our tests, not just on the places it returned, but on the interface: results are presented in a simple carousel and you can quickly jump to a Google Maps view. Also Google Assistant remembers what you’ve already said, speaks in foreign languages. Like Apple’s Siri, it is much more than an assistant, despite the name: it will read you poetry, tell you a joke, or play a game with you.
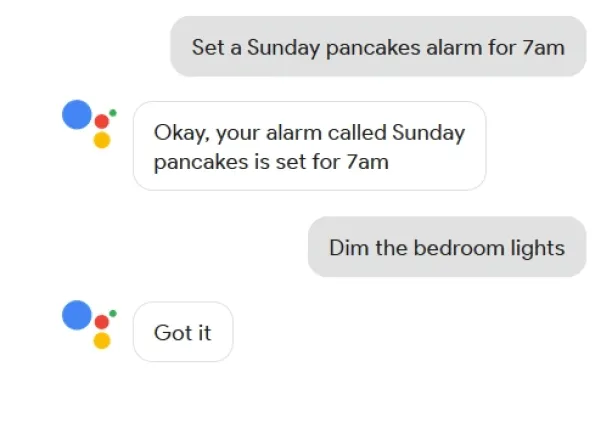
- Google Home: You will be able to get hands-free help from your Assistant embedded in Google Home. Say “Ok Google” to get the morning news or manage your schedule.
- Waymo an autonomous driving technology company became a subsidiary of Alphabet in 2016. Though Waymo announced in 2018 that they would be making self-driving cars available by 2020. We still don’t see them hitting the road as of 2021 due to regulatory concerns and complexity of self-driving.
- For fun and experimentation you can visit AI experiments for videos on AI experiments.
Discountinued products
- Messaging App Allo and its Smart Reply functionality was launched with significant functionality but shut down in 20181 : Google joined the messaging app battle with Allo. As a messaging app, Allo lets you express yourself with stickers, doodles, HUGE emojis & text. Furthermore it brings you the Google Assistant without leaving the conversation. The AI algorithm used to learn your style and provide more suggestions, which makes your message more ‘you’. You could get creative with the photos you send by doodling on them or adding text or draw a smiley face, turn your friends into memes, and mix in some color.
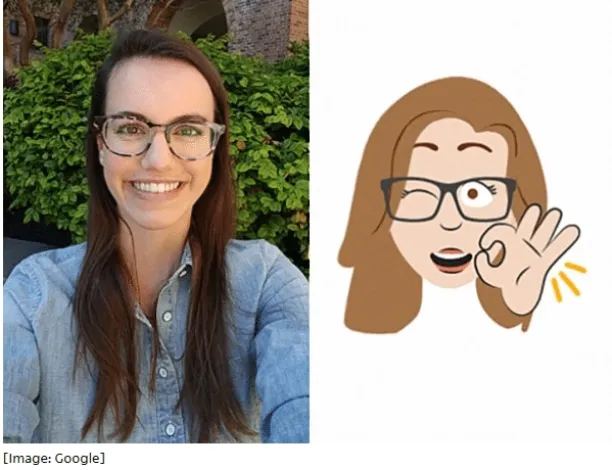
Adding a new feature turning a selfie into an emoji, Google says that the algorithms powering the new tool are powerful, machine-learning technologies that could generate 563 quadrillion different faces. The search giant is using neural networks to create custom emojis.
Now that you know what Google is up to in AI, you can explore the rest of the AI universe. You can check out
- AI applications in marketing, sales, customer service, IT, data or analytics.
- enterprise automation use cases such as process mining, RPA, conversational AI or document automation.
You can also our list of AI tools and services:
And If you have a business problem that is not addressed here:
Comments
Your email address will not be published. All fields are required.