Despite the controversies surrounding this technology, the facial recognition systems (FRS) market continues to grow1 . Facial recognition applications are everywhere, from helping improve mental disorder diagnoses to finding fugitives. Developing and improving these systems requires facial data, which sometimes can be challenging to obtain due to security and privacy-related concerns of people.
Choosing the proper method is a crucial step in simplifying the facial recognition data collection process; therefore, we explained:
- What does data collection mean for facial recognition systems
- What are the top 4 methods of collecting facial data
- And our recommendations on how to select the proper method for your project.
What does data collection mean for facial recognition systems?
We have discussed data collection and how it fuels AI/ML models before. Facial recognition is a branch of image recognition that also functions through AI/ML models. Data collection for facial recognition falls under image data collection and involves gathering face images to train and improve FRSs.
Face images are taken of different people, annotated, and then fed into a machine learning model, which uses them to learn how to scan, identify, and process facial features.
The basic steps included in in-house face image data collection:
- Understand the scope of the project (what kind of faces it will scan, the size of the population it will be deployed on, etc.)
- Recruit participants
- Take images based on the requirements of the project
- Organize and pre-process the photos based on attributes
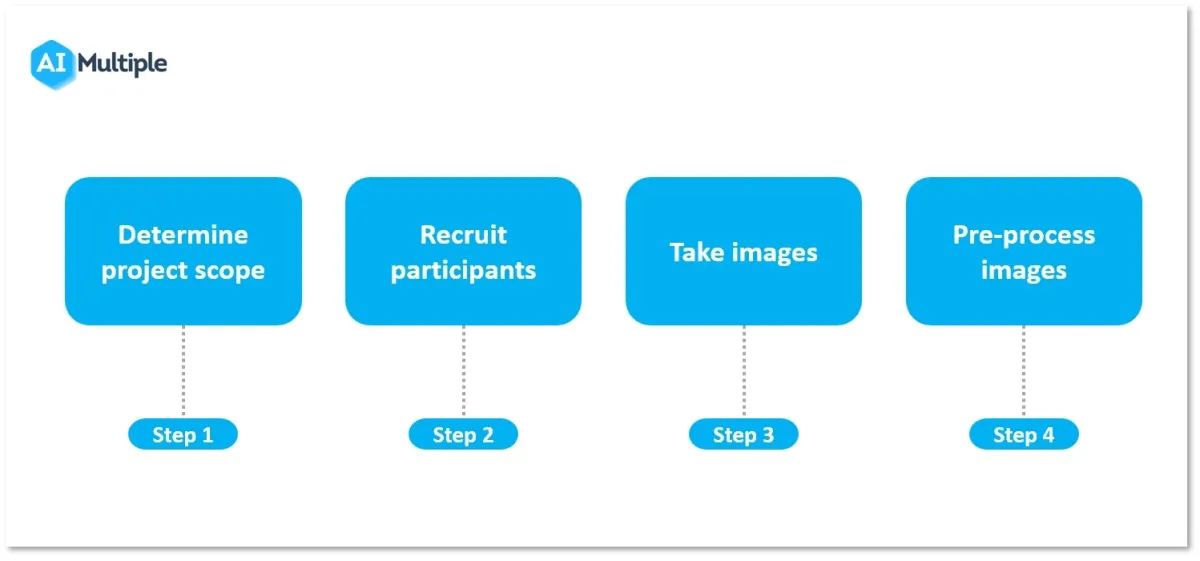
What are the top 4 methods of collecting face image data?
Different methods of data collection are used for facial recognition systems. Here, we identify the most commonly used ones.
1. Prepackaged/Public face image dataset
A third party creates these datasets, which are ready to use after purchase. Prepackaged datasets are also sometimes available for free, like the CelebA dataset 2 .
Advantages
- Easy to access: They are readily available online and can be downloaded quickly.
- Cheaper: They are more affordable than in-house image data collection and sometimes free.
Disadvantages
- Lack of customization: The dataset is not unique to your project requirements. For instance, if the dataset does not have images with a specific type of obstruction on the face, such as masks or glasses, then additional data must be added, which can add preprocessing costs to the budget.
- Quality level: The quality can be low since such datasets (especially public datasets) do not go through rigorous quality checks and are collected by the general public.
2. Face image data collection through crowdsourcing
The crowdsourcing method includes gathering fresh face image datasets from the public. If done in-house, the company needs to develop an online platform allowing the crowd to register, get data collection projects, and submit the data in exchange for compensation.
Advantages
- Scalable and customizable: You can customize and scale face image datasets by specifying your requirements to the service provider.
- Cheaper: Since the crowd uses cameras/smartphones, this method becomes more affordable than other in-house collections.
- More diverse data: The image dataset collected with crowdsourcing is more varied (Images of people with different skin colors, hairstyles, ages, etc.) since more contributors can be reached worldwide.
- No copyright issues: When the crowd registers through the platform, they agree to the terms and conditions, which transfer the rights of the image data to the company.
Disadvantages
- Quality issues: The quality can be affected since the images are collected by the contributor’s smartphones/cameras. For this, the company needs to provide clear and comprehensive quality instructions or spend extra effort in preprocessing the images the crowd collects.
- Platform cost: Crowdsourcing is done through an online platform (usually a mobile application); developing or purchasing such software can add extra time and costs to the process.
Case Study: Fielddrive’s Event Check-In System
Fielddrive, an event management platform, uses crowdsourcing to gather facial data from global attendees. Participants opt in during registration and submit selfies via a mobile app. This method enabled Fielddrive to create a diverse dataset spanning 50+ countries, which powers their 6-second check-in system. However, the company invests heavily in preprocessing to standardize image quality due to variations in smartphone cameras. 3
3. Automated face image data collection
Image data for facial recognition systems can also be automated by integrating machine learning into the process. This can be done through web scraping or crawling, where the data is scraped from specified or unspecified online sources.
Advantages
- No human input: This does not require human input after the initial setup.
- It does not tire: It can collect face images non-stop without human error.
- Cheaper: It is more affordable since no equipment or recruitment of contributors is required.
Disadvantages
- Additional preprocessing costs: It can add preprocessing costs to the budget since the scraped/crawled data needs to be cleaned and processed.
- Limitations from anti-scraping techniques: Online sources use various anti-scraping techniques to block web scraping bots. Check out this article to learn some best practices to overcome web scraping challenges.
Case Study: Clearview AI
Clearview AI scraped billions of images from public websites and social media to build its controversial facial recognition database. Law enforcement agencies use this dataset to identify suspects in real time. However, this method faces legal challenges due to privacy violations, highlighting the ethical risks of unregulated scraping. 4
4. In-house face image data collection
This method involves creating a separate image data collection project to develop a facial recognition system. The team must purchase cameras and other lighting equipment and hire contributors to take their images.
Advantages
- Higher data protection: Good for projects that are confidential in nature. For instance, a facial recognition system is being used by the FBI or the government 5 .
- More control: The data collection process can be more controlled. The team can use the cameras, contributors, and settings (lighting, background, angle, etc.) of their choice.
- Complete data ownership: Since an image of someone’s face is their biometric information, getting ownership rights is essential before using it. In-house image data collection allows the company to own the data completely and eliminates the risk of future data-related lawsuits. This is because the contributors sign an agreement with the company during recruitment.
Disadvantages
- Expensive: This method is the most expensive since the company covers all the expenses itself.
- Lack of diversity: This method limits the level of diversity in the dataset since a smaller number of contributors can be hired compared to, for instance, crowdsourcing.
- Time-consuming: This method is also more time-consuming than other methods, such as using prepackaged data or working with a crowdsourcing data collection service.
Recommendations on how to choose the right method
Selecting the proper method for your facial recognition project depends on your project. The following factors can be considered:
Project’s scope
It is important to consider the size of your project. For instance, if a facial recognition system is deployed in only one country, pre-packaged or even public datasets can be used to train it. However, crowdsourcing would be more suitable if it were deployed in multiple countries and required a dataset from different people.
Project’s level of confidentiality and complexity
Confidential projects can benefit from in-house image data collection. In contrast, projects that are not secretive or have a complicated model can use public datasets.
Project’s budget
As previously mentioned, in-house data collection can be expensive and time-consuming; therefore, working with prepackaged datasets or crowdsourcing data collection service providers can be more suitable if the project has time and budget limitations.
References
- 1. Facial recognition global market size 2032| Statista. Statista
- 2. Liu, Z., Luo, P., Wang, X., & Tang, X. (2018). Large-scale celebfaces attributes (celeba) dataset.
- 3. Streamlined Event Check-Ins with Facial Recognition | fielddrive.
- 4. https://www.oaic.gov.au/news/media-centre/clearview-ai-breached-australians-privacy
- 5. Facial recognition used in arrest of 9/11 conspiracy theory lawyer accused of trying to disarm officer on Jan. 6. NBC News
Comments
Your email address will not be published. All fields are required.