Network observability offers insights into a network’s performance metrics, allowing organizations to identify and address vulnerabilities. Our approach supports network monitoring and analysis for timely issue resolution. Leveraging AI, it facilitates the automatic detection of anomalies in network traffic and network performance.
Top 9 network observability tools
Vendors | Reviews | Free Trial | Employees** | Price |
---|---|---|---|---|
Paessler PRTG | 5.6/5 based on 91 reviews | ✅ for 30-days | 350 | Starting $1,750 /month -50 devices |
AKIPS | 5/5 based on 4 reviews | ✅ for 30-days | 14 | Not shared publicly |
Obkio | 4.9/5 based on 51 reviews | ✅ for 14-days | 24 | Starting $399 /month -10 agents |
ManageEngine Site24x7 | 4.7/5 based on 233 reviews | ✅ for 30-days | 302 | Standard Edition: $245 [25 devices] Professional Edition: $345 [25 devices] and more options |
Dynatrace | 4.5/5 based on 1,242 reviews | ✅ for 15-days | 4,200 | Full-Stack Monitoring: $0.08 per hour for an 8 GB host. Infrastructure Monitoring: $0.04 per hour Kubernetes Monitoring: $0.002 per hour and more options. |
Cisco ThousandEyes | 4.5/5 based on 77 reviews | ✅ for 15-days | 761 | Not shared publicly |
LogicMonitor | 4.5/5 based on 502 reviews | ✅ for 15-days | 1,070 | Logs correlation: $4.00 USD/GB/month for 30 day retention $7.00 USD/GB/month for yearly retention and more options |
Datadog | 4.3/5 based on 464 reviews | ✅ for 14-days | 5,200 | Starting $15 /month per host |
Zabbix | 4.3/5 based on 185 reviews | N/A | 126 | open source |
* Reviews are based on Capterra and G2. Transparency statement: Vendors are ranked according to their average ratings, except sponsors which receive links.
** The number of workforce is gathered from the companies’ LinkedIn pages.
Common Features of Network Observability Tools
Below are the key shared features of network observability tools that make these tools indispensable for organizations:
- Real-time network monitoring: All mentioned tools can monitor network performance in real time.
- Cloud, hybrid, and on-premises support: These network observability tools are designed to integrate with various environments, including cloud-based, hybrid, and on-premises setups.
- Analytics and visualization: With the deployment of analytics and visualization tools, these platforms provide insights into network performance.
- Root Cause Analysis: Helps pinpoint the sources of problems for faster troubleshooting.
- Web app monitoring (HTTP, FTP, Browser): The network observability tools offer insights into web application performance.
- Scalability for large networks: Platforms such as AKIPS emphasize scalability, ensuring that they can handle monitoring demands.
Read more: Network security software
Leading Network Observability Tools
1. Paessler PRTG
Paessler PRTG Network Monitor is a network monitoring tool designed to track and analyze network traffic, bandwidth usage, and device performance. PRTG is used for its versatility and ability to adapt to networks of different sizes and complexities.
Key features:
- Customizable Alerts: Allows users to set up notifications for specific conditions, such as high bandwidth usage or device failures.
- Dashboards and Reporting: Offers a range of visualization options to make network data easier to understand and analyze.
- Network Observability: Tracks and visualizes the entire network infrastructure, helping identify bottlenecks, anomalies, and inefficiencies.
Pros:
- User-Friendly Interface: The setup process is intuitive, and the interface is straightforward, which reduces the learning curve.
- Customizable Alerts: Users can define thresholds for different metrics and receive alerts via email, SMS, or push notifications.
Cons:
- Resource Intensive: Deploying PRTG on larger networks may require substantial system resources, which can impact performance on older servers.
- Overwhelming for Small Networks: PRTG’s extensive features might be excessive and unnecessary for very small or simple networks.
2. AKIPS Network Monitoring
AKIPS Network Monitoring specializes in network observability for complex environments, offering monitoring capabilities that ensure network visibility. Explore AKIPS with a free trial.
Key Aspects:
- Network Discovery: Utilizes a method by pinging every IP address, avoiding network overload and eliminating the need for manual CSV file inputs for network discovery.
- Device Fingerprinting: Employs SNMP v3 engine ID and interface MAC addresses for precise device identification, automatically removing duplicate device entries.
- Vendor-Specific MIB Detection: Identifies and configures vendor-specific Management Information Base (MIB) objects, enhancing device compatibility.
Pros:
- Filtering options: It offers the ability to filter devices by sysName, vendor, model, or location, facilitating targeted network management.
- Alerting and event handling: The tool features alert and event management capabilities, with the ability to filter out noise and prioritize critical events through regex and grouping rules.
Cons:
- Learning curve for advanced features: The features of AKIPS might present a learning curve, especially for users new to network management systems, requiring time to fully leverage its capabilities.
- Potential Over-reliance on Ping Methodology: The ping-based discovery method may not capture all nuanced network scenarios or devices with ping responses disabled.
3. Obkio
Obkio simplifies network observability, focusing on monitoring and the end-user experience to detect and resolve network issues before they impact business operations.
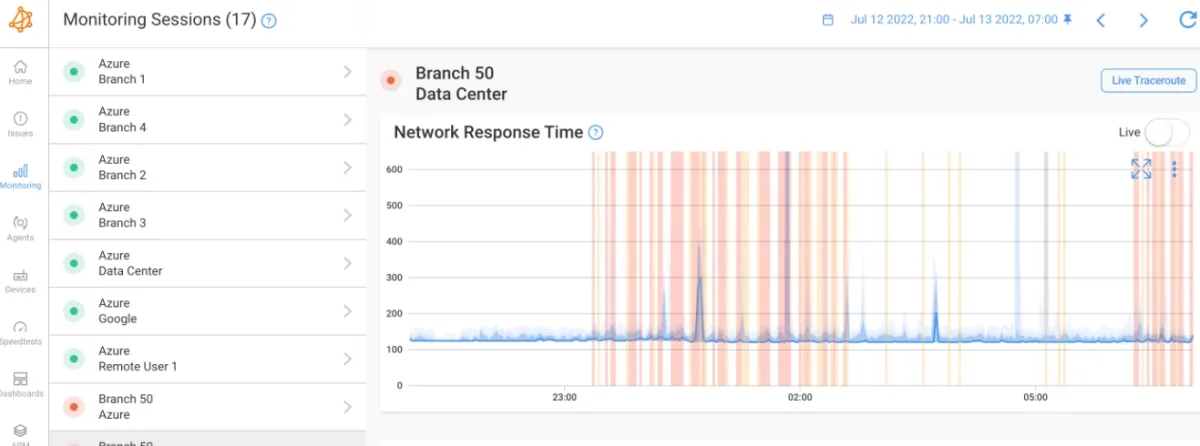
Figure 2 : Obkio Monitoring Sesions
Source: Obkio Website1
Key Aspects:
- Monitoring agent deployment: Obkio’s Monitoring Agent can be installed on various systems like Windows, Linux, VMWare, and Hyper-V, offering flexibility in monitoring different environments.
- Plug-and-Play Hardware Appliance: For locations lacking IT servers, Obkio provides a Hardware Agent that’s easy to deploy, ensuring branch offices are not left out of the monitoring ecosystem.
Pros:
- Obkio’s user-friendly design simplifies network monitoring for IT teams
- Its plug-and-play hardware agent makes it easily deployable in locations without IT infrastructure.
Cons:
- Obkio involves limitations in customization for advanced users
- The reliance on cloud storage might raise concerns for businesses with strict data sovereignty requirements.
4. ManageEngine Site24x7
ManageEngine Site24x7 provides insights into uptime and operational efficiency from a global perspective, leveraging over 130 monitoring locations worldwide and private network options.
Key Aspects:
- Public and Private Cloud Monitoring: Ensures visibility across cloud resources, supporting platforms like AWS, Azure, GCP, and VMware for workload monitoring and app performance troubleshooting.
- Synthetic Web Transaction Monitoring: The tool records and simulates multi-step user interactions in real browsers to optimize web applications like login forms and shopping carts.
Pros:
- Advanced Analytical Tools and AIOps Integration: Utilizes cutting-edge analytical tools and integrates artificial intelligence for IT operations (AIOps), enhancing anomaly detection and facilitating swift incident resolution.
- Real User and Synthetic Monitoring Capabilities: Equips organizations with both real user monitoring (RUM) and synthetic transaction monitoring, enabling a thorough application performance from multiple perspectives.
Cons:
- Challenges in Plugin Implementation: Using standard or custom plugins is challenging due to the complexity of measurement methods and the setup of thresholds and availability checks, often leading to false positives.
- Difficulty in Configuring Network Elements: Configuring Network element is not easy as configuring WEB and Servers.
5. Dynatrace
Dynatrace offers an AI-enhanced platform for network observability, providing insights from the underlying infrastructure to user experience aspects.
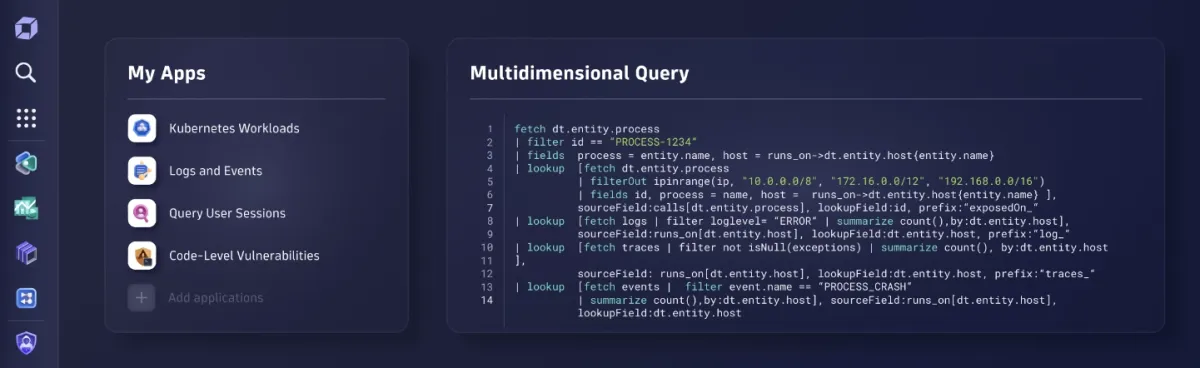
Figure 4: Dynatrace User Interface
Source: Dynatrace Website2
Key Aspects:
- PurePath Technology: Provides end-to-end transaction tracing for detailed visibility.
- Davis AI: Dynatrace’s AI engine, detects anomalies and provides root cause analysis.
Pros:
- Unified monitoring: Dynatrace’s OneAgent technology enables automatic data collection and full-stack instrumentation.
- Integration capabilities: With over 600 technology integrations, Dynatrace offers connectivity with a wide range of cloud platforms.
Cons:
- Learning Curve: The platform’s advanced features and capabilities require a significant time investment to learn and master, potentially slowing initial adoption.
- Dependence on Proprietary Technology: Dynatrace’s OneAgent creates a dependency on proprietary technology, which could limit flexibility in integrating with custom or niche monitoring solutions.
6. Cisco ThousandEyes
Cisco ThousandEyes focuses on internet, cloud, and digital experiences to optimize user interactions across global networks.
Key Aspects:
- Internet and Cloud Intelligence: The tool provides insights into external dependencies that impact network performance.
- Path Visualization: Cisco ThousandEyes offers a view of network paths, identifying issues across internal and external networks.
Pros:
- Visibility: Cisco ThousandEyes offers application-aware monitoring and DNS, BGP, and VoIP/UCaaS monitoring, enabling thorough understanding and oversight of digital experiences.
- End User Experience Monitoring: Provides insights into user experience from employee devices, including network path visualization and performance metrics (Wi-Fi strength, VPN, DNS).
Cons:
- Learning Curve: Users report a significant learning curve to fully grasp and utilize the product’s extensive capabilities, which can delay maximizing its potential.
- Dependency on External Agents: The reliance on Cloud and Enterprise Agents for data collection might introduce complexities in deployment and management.
7. LogicMonitor
A cloud-based service that offers network observability, emphasizing automation and coverage of network devices and flows.
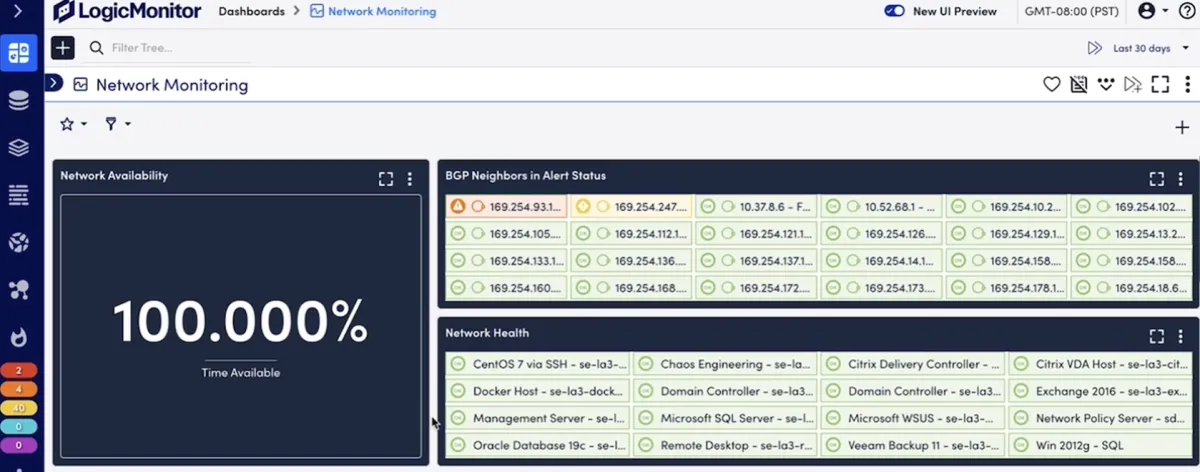
Figure 6: LogicMonitor User Interface
Source: LogicMonitor Website3
Key Aspects:
- Dynamic Topology Mapping: Offers real-time network visualization through dynamic topology maps, enhancing understanding of network structure and performance.
- Agentless Collector for Quick Deployment: Utilizes an agentless collector for rapid deployment, providing network visibility without extensive setup.
Pros:
- Network visibility: LogicMonitor’s support for over 2000 integrations allows device and network type monitoring.
- Ease of setup and maintenance: The agentless collector facilitates deployment and management, providing quick setup and network insights with minimal operational overhead.
Cons:
- Complexity for Smaller Organizations: The functionalities and integrations presents a steep learning curve for smaller organizations.
- Cost Consideration: The cost of LogicMonitor, especially at scale with its advanced monitoring capabilities, is a significant consideration for budget-conscious businesses.
8. Datadog
Datadog enhances network observability by offering a cloud-based platform that aggregates real-time data from applications.
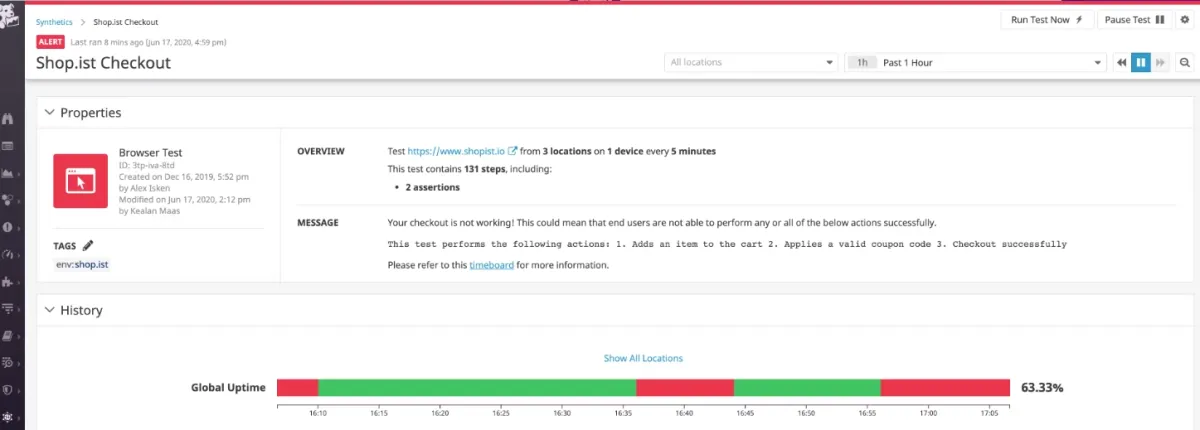
Figure 8: Datadog User Interface
Source: Datadog Website4
Key Aspects:
- Live network maps: Datadog offers a dynamic visualization of network traffic flow and dependencies.
- Service-level objective (SLO) tracking: This tool monitors and ensures the network performance aligns with business objectives.
Pros:
- Network Observability: Datadog enables end-to-end visibility into application performance, infrastructure health, and security posture by integrating distributed tracing with metrics, logs, and telemetry.
- Advanced Problem Detection: With features like automatic anomaly detection, code profiling, and real-time service monitoring, Datadog identifies operations and infrastructure issues, facilitating timely optimizations and preventing potential disruptions.
Cons:
- Complexity for Smaller Teams: The features of Datadog can be overwhelming for smaller teams or organizations without dedicated DevOps or security personnel, potentially steepening the learning curve.
- Cost at Scale: The price of the tool can increase significantly with the ingestion and processing of extensive logs and telemetry data, potentially affecting projects with tight budgets.
9. Zabbix
Zabbix provides a network observability solution, designed to accommodate the expansive monitoring needs from smart home applications to large, multi-tenant enterprise environments.
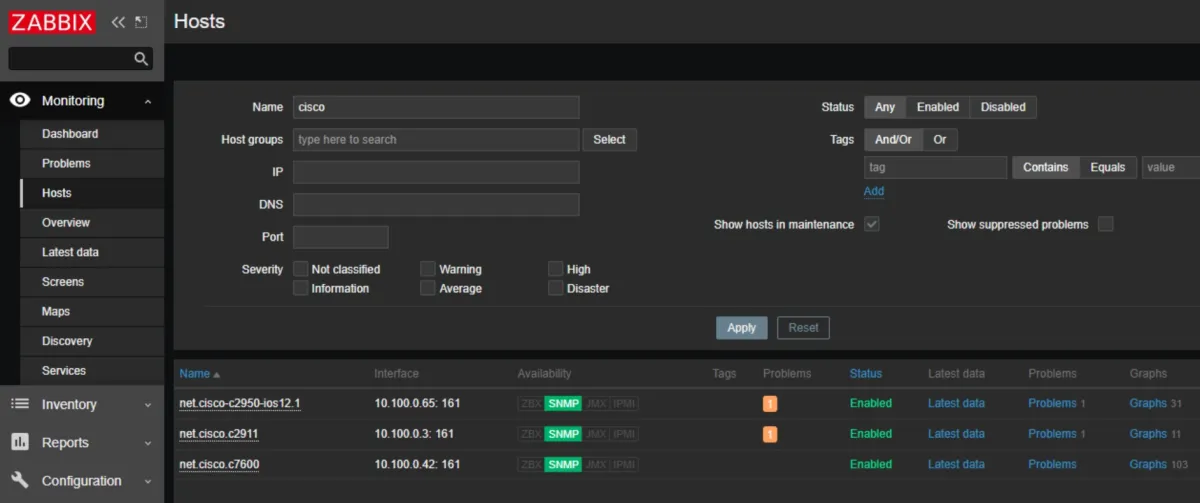
Figure 10: Zabbix Advanced Visualization, dark theme
Source: Zabbix Website5
Key Aspects:
- Integration capabilities: The tool offers ready-to-use templates for easy integration with existing alerting, ticketing, IoT, and ITSM systems.
- Global partner and vendor support: Zabbix is uniquely supported by over 250 global partners and various external vendors.
Pros:
- Monitoring capabilities: Zabbix excels in its ability to monitor various metrics, offering numerous data collection, transformation, and visualization options to suit different monitoring needs.
- Availability and security: It provides a high availability solution to ensure continuous uptime and incorporates features like external vault storage for sensitive information, emphasizing data security and infrastructure reliability.
Cons:
- Complex Configuration: Zabbix has a complex setup and configuration process, requiring a steep learning curve for new users.
- Resource intensity for large deployments: In very large or complex environments, Zabbix can become resource-intensive, necessitating careful planning and potentially significant hardware investment to maintain performance.
Free network monitoring tools are an option for SMEs looking to improve their network efficiency and security without high expenses. If you are associated with a small or medium-sized business, check out the top free network monitoring tools.
FAQ
What distinguishes network observability from network monitoring?
While related, network monitoring and observability tools serve distinct functions in managing network health. Network observability tools are focused on tracking specific metrics and thresholds to maintain network performance. In contrast, network observability offers a more expansive and in-depth perspective, incorporating network monitoring’s capabilities while adding context, real-time visibility, and adaptability for complex and evolving networks.
Think of network observability tools as an advanced stage of network monitoring. It enhances monitoring’s foundational aspects by incorporating more diverse data sources, sophisticated analytics, and intelligent automation. This evolution enables deeper insights into network infrastructure, facilitating informed decision-making for superior network performance, security, and reliability.
What are top key must-have features of Network Observability tools?
Problem-Solving and Root Cause Identification: Provides detailed insights into network operations, facilitating quicker and more accurate troubleshooting and issue resolution for network observability tools.
Network Performance and Resource Optimization: Allows for the analysis of network usage and performance data to identify optimization opportunities, improving efficiency and user experience.
Network Capacity Management and Growth Planning: Supports strategic planning for network capacity and scalability by analyzing usage trends and forecasting future demands.
Network Behavior Insights: Offers a holistic view of the network, aiding in the understanding of complex interactions and the optimization of network performance.
Data-Informed Strategic Decisions: Empowers organizations to make informed decisions regarding network management and optimization based on actionable data insights.
Can small businesses benefit from network observability tools?
While the scale and complexity may vary, small businesses also face network observability and security challenges that can be effectively managed with the right observability tools.
How do AI and machine learning enhance network observability tools?
AI and machine learning algorithms analyze vast amounts of data to identify patterns, anomalies, and predict potential issues, enabling more proactive and efficient network observability.
What is Telemetry?
Telemetry is the automated process of collecting data from remote or inaccessible devices and systems, crucial for network monitoring. It enables the real-time tracking of network observability, health, and operational metrics, facilitating proactive issue detection and resolution, thereby enhancing network observability and efficiency.
How to select the best observability tools?
Choosing the network observability tools depends on several factors, including the size and complexity of your network, specific monitoring and analytics needs, budget constraints, and integration requirements with existing systems.
Integration: Look for solutions that seamlessly integrate with your existing IT ecosystem.
Customization: Customizable dashboards and alerts can greatly enhance usability and relevance.
Support and Community: Consider the level of support offered by the vendor and the presence of an active user community for sharing best practices.
For more on network monitoring
- Top 7 Free Network Monitoring Tools based on 1,000+ Reviews
- Top 10 Network Monitoring Software based on 3,000+ Reviews
- In-Depth Look at AKIPS Network Monitoring Software
- Top 5 Network Performance Monitoring Tools
External Resources
- 1. Obkio Reviews 2025. Verified Reviews, Pros & Cons | Capterra.
- 2. Obkio Reviews 2025. Verified Reviews, Pros & Cons | Capterra.
- 3. Obkio Reviews 2025. Verified Reviews, Pros & Cons | Capterra.
- 4. Obkio Reviews 2025. Verified Reviews, Pros & Cons | Capterra.
- 5. Obkio Reviews 2025. Verified Reviews, Pros & Cons | Capterra.
Comments
Your email address will not be published. All fields are required.