The integration of artificial intelligence (AI) into network monitoring enhances availability and performance. Here are options for you:
- If you’re searching for an AI network monitoring tool, compare the top options and pricing with our curated selections.
- Are you interested in discovering how AI is enhancing network monitoring? Explore how AI technologies streamline operations, boost efficiency, and drive smarter network management.
Real-life Examples
Case study #1 from Juniper Networks
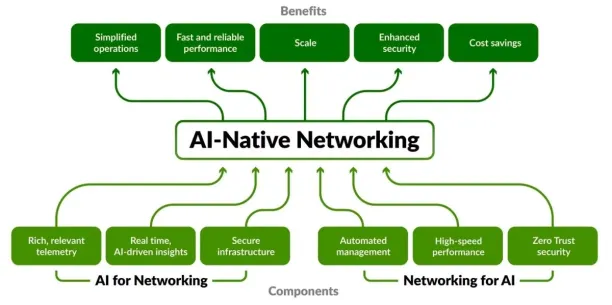
Source: AI-Native Networking Diagram1
Juniper Networks is a networking technology, offering solutions for enterprise and service provider networks. Its AI-native networking platform offers artificial intelligence and machine learning to optimize network performance, enhance security, and automate network management tasks.
Expert, a large electronics reseller in Germany, utilized the Juniper AI-Native Networking Platform, introduced by Wayv, a specialist in wireless network engineering. This adoption aimed to optimize network management and user experience across its operations.
The AI capabilities of the Juniper platform provided experts with enhanced operational insights and automation, significantly improving network reliability and security. Notably, the Marvis Virtual Network Assistant identified and resolved issues such as VLAN misconfigurations and DHCP errors efficiently.
As a result, Expert achieved a streamlined and responsive network environment that supports its diverse operational needs 2 .
Case study #2: DataDog
Watchdog, Datadog’s AI engine, offers automated notifications, insights, and root cause analyses derived from observability data spanning the entire Datadog platform.
Zakir Mohammed’s team at Toyota Motor North America has integrated Datadog. Deploying Datadog proved efficient in cutting:
- Set up time by deploying the agent within minutes
- Mean Time to Resolution (MTTR) by 80%, streamlining troubleshooting processes.
When network disruptions plagued Toyota’s Automated Guided Vehicles (AGVs), Datadog identified the root cause, saving the company substantial production costs and resolving the issue within hours.
Transitioning from reactive to proactive monitoring, Toyota utilizes Datadog’s Watchdog feature to forecast and prevent future outages, enhancing operational reliability.
Case study #3 from Dynatrace Davis AI
Dynatrace offers an AI network monitoring engine called Davis, which is integral to its software intelligence platform. Davis analyzes data across the digital ecosystem, including clouds, applications, and infrastructure.
Prior to 2016, BARBRI, a legal exam prep platform, operated in a hybrid environment, utilizing both on-premises servers and cloud services. Recognizing the need to scale its operations and enhance resource utilization, BARBRI transitioned to a fully cloud-based infrastructure.
Dynatrace’s platform was deployed to monitor BARBRI’s entire Azure environment, ensuring that the migration and ongoing operations were managed efficiently. The AI capabilities of Dynatrace allowed BARBRI to monitor and gain real-time insights into BARBRI’s topology. Therefore, BARBRI scaled its Azure environment to meet demand increases during peak times and enhance the user experience.3
Source: Dynatrace Davis AI User Interface4
Case Study #4 from Cisco AI Network Analytics
Cisco’s AI Network Analytics, part of its DNA Center, uses machine learning to provide insights into network performance. It helps network managers predict issues, optimize network performance based on predictive analytics, and fine-tune the network proactively.
Cisco AI Network Analytics has been utilized in various scenarios, including detecting unusual patterns that may indicate security threats or operational issues, thereby enabling quicker remedial action.
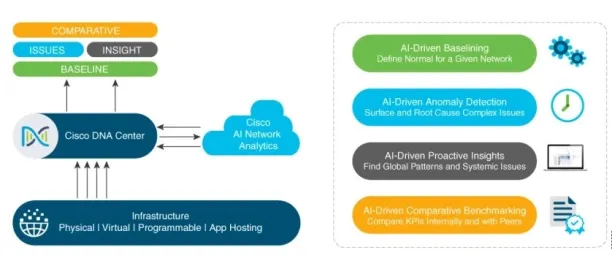
Source: Cisco AI Network Analytics Features 5 .
REWE Group implemented Cisco AI Network Analytics to enhance their network management capabilities. This collaboration has reduced the time needed to resolve network issues, allowing the IT team to allocate more time to new projects and innovations crucial to business operations.
The application of AI/ML has simplified the handling of network workloads, making daily management tasks less time-consuming and highlighting critical alerts that indicate connectivity or performance issues.6 .
Case study #5 from Anadot
Anodot is a provider of AI-powered analytics solutions designed to detect anomalies in real-time data. Its platform utilizes machine learning algorithms to identify deviations from expected patterns, enabling businesses to address issues and capitalize on opportunities.
LivePerson, a conversational AI platform, implemented Anodot’s real-time analytics to monitor a complex array of nearly 2 million metrics every 30 seconds across its global data centers. This deployment was crucial to ensuring 24/7 service uptime and the continuous availability of customer data.
Anodot’s AI capabilities enable LivePerson to detect and respond to anomalies in real-time, thereby maintaining high customer satisfaction and operational efficiency. 7
Based on these case studies, AIMultiple identified AI use cases in network monitoring:
AI Use Cases In Network Monitoring
By using AI capabilities, businesses can enhance their network monitoring practices in various ways. Here are some use cases of AI in network monitoring:
- Anomaly detection: AI network monitoring tools can quickly identify unusual patterns or deviations from normal network behavior, which might indicate a security breach or system failure.
- Predictive analytics: By analyzing historical data, AI can predict potential network failures or performance degradations before they occur.
- Automated configuration and optimization: AI can automate routine network configuration tasks and optimize network settings based on current traffic patterns and demands.
- Security enhancement: AI enhances network security by detecting and responding to threats in real time. It can quickly identify malware, ransomware, and other malicious activities, minimizing potential damage.
- Root cause analysis: When problems occur, AI can help diagnose the root cause more quickly than traditional methods. By correlating various data points and identifying patterns, AI reduces the time needed to troubleshoot and resolve issues.
- Capacity planning: AI can forecast future network needs based on trend analysis, helping organizations plan upgrades and expansions more effectively.
AI Network Monitoring Tools
Vendors | Reviews | Number Of Employees | Free Trial | Pricing |
---|---|---|---|---|
NinjaOne | 4.7 based on 1,428 reviews | 1,219 | ✅ (14-day) | Not shared publicly. |
Dynatrace | 4.4 based on 1,494 reviews | 5,018 | ✅ (15-day) | Full-Stack: $0.08 per hour / 8 GiB host Infrastructure: $0.04 per hou Application Security: $0.018 per hour / 8 GiB host Real User: $0.00225 Per session Synthetic: $0.001 Per synthetic request |
LogicMonitor | 4.5 based on 843 reviews | 1,122 | ✅ (14-day) | Infrastructure Monitoring: $22 USD per resource/month Cloud IaaS Monitoring: $22 USD per resource/month and more options. |
Auvik | 4.3 based on 508 reviews | 346 | ✅ (14-day) | Not shared publicly. |
** Reviews are based on Capterra and G2. Vendors are ranked according to their number of reviews
*** Free trial periods and pricing are included if the content is publicly shared.
1. Dynatrace
Dynatrace offers its AI engine, Davis, which significantly enhances network and application monitoring capabilities. Davis automates complex processes, such as root cause analysis, anomaly detection, and predictive insights, making it a powerful tool for proactive monitoring.
Dynatrace isn’t limited to just network monitoring—it excels in Application Performance Monitoring (APM), cloud infrastructure management, and digital experience monitoring, offering a comprehensive solution for managing the entire digital ecosystem.
2.LogicMonitor
With its AI-driven insights, LogicMonitor can automate anomaly detection, enabling it to identify unusual network behaviors before they escalate into critical issues. The platform’s AI also supports predictive analytics, allowing IT teams to anticipate potential network problems and address them proactively.
Additionally, LogicMonitor utilizes AI to provide intelligent troubleshooting, thereby reducing the time required to resolve incidents and enhancing overall network performance. This makes LogicMonitor a powerful tool for organizations that require real-time network monitoring, along with the added benefits of AI-driven automation and intelligence.
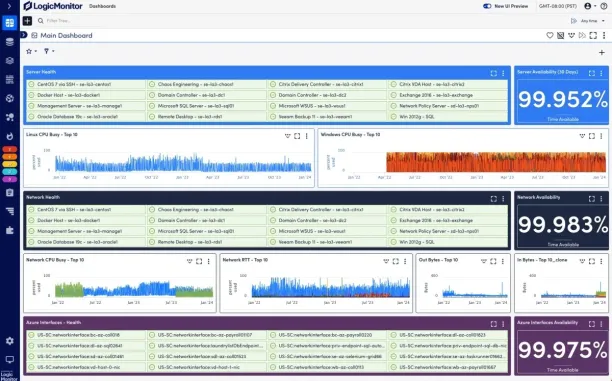
3. Auvik
Auvik integrates AI-driven features to enhance its network monitoring and management capabilities. Auvik’s AI helps network operations by automating tasks such as network mapping, device discovery, and configuration backups.
Its anomaly detection features utilize AI to identify unusual patterns in network behavior, enabling IT teams to spot potential issues before they escalate quickly. Additionally, Auvik’s AI-powered insights offer predictive analytics, enabling proactive maintenance and optimization of network performance.
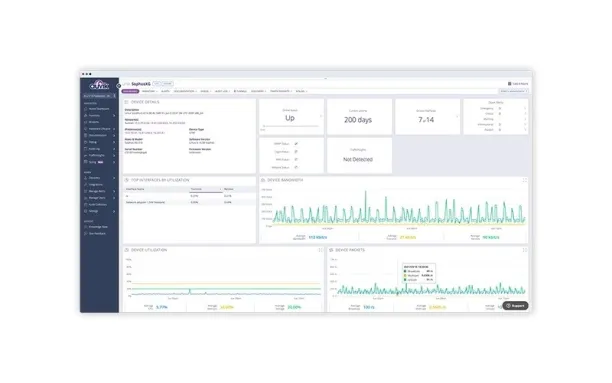
4. NinjaOne
NinjaOne integrates AI-driven features to enhance its network monitoring capabilities. It focuses on automation, real-time monitoring, and proactive issue resolution. Key AI-powered features include automated anomaly detection and alerts.
NinjaOne also supports predictive analytics to prevent problems before they escalate, and it automates routine tasks such as network discovery, device monitoring, and patch management. This level of automation reduces downtime and improves network reliability.
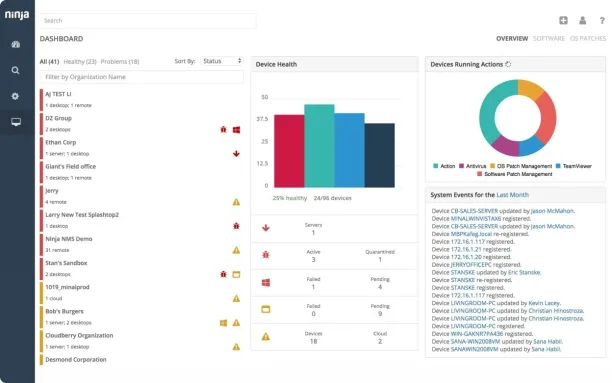
FAQs for AI Network Monitoring
How does AI improve network monitoring?
AI enhances network monitoring by automating tasks, improving anomaly detection accuracy, offering predictive insights, and managing large-scale and complex networks more effectively.
Are there any drawbacks to AI network monitoring?
While AI network monitoring offers numerous benefits, challenges include integration complexities with existing systems and the requirement for significant initial setup and tuning.
Can AI network monitoring adapt to changes in network architecture?
Yes, AI network monitoring systems are generally designed to adapt to changes in network architecture. They continuously learn from network behavior, enabling them to effectively adjust to new devices, configurations, and traffic patterns.
How does AI-based network monitoring address security concerns?
AI network monitoring enhances security by continuously analyzing network traffic for unusual patterns, detecting potential threats in real-time, and automating responses to security incidents, thereby improving overall network resilience.
Can AI network monitoring reduce operational costs?
Yes, AI network monitoring can significantly reduce operational costs by automating routine tasks, minimizing downtime through predictive maintenance, and optimizing network performance, which in turn reduces the need for frequent hardware upgrades and manual troubleshooting.
External Links
- 1. What is AI-Native Networking? | HPE Juniper Networking US.
- 2. expert Case Study | HPE Juniper Networking US.
- 3. Dynatrace Case Study.
- 4. Meet Davis, our powerful AI-engine.
- 5. “Cisco AI Network Analytics Overview”. Accessed: April 24 2024.
- 6. Cisco AI Network Analytics: Making Networks Smarter and Simpler to Manage - Cisco Blogs. Cisco Systems
- 7. LivePerson Uses AI-Powered Analytics to Address Most Challenging Customer Engagement Issues - Anodot.
Comments
Your email address will not be published. All fields are required.