Mental health challenges are a worldwide concern, especially after the COVID-19 pandemic, which saw an estimated 76 million additional cases of anxiety disorders.1 This heightened stress strained healthcare systems and increased demand for mental health support. Yet, traditional care faces barriers like professional shortages, high costs, and social stigma.
Integrating AI into healthcare can help with these challenges by enhancing early diagnosis, personalizing treatments, and expanding access through digital tools.
Explore top AI for mental health tools, key components, use cases with real-life examples, and challenges in implementation.
Top 7 AI powered tools for mental healthcare
Tool | Focus | Tool Type |
---|---|---|
Cogito | Real-time AI coaching for customer service, emotional intelligence enhancement, empathy training. | AI Coaching System |
Headspace | Meditation and mindfulness programs, virtual mental healthcare services, guided therapy and psychiatric support. | Mental Wellness Platform |
Limbic | AI automation for mental health professionals, documentation management, patient engagement enhancement. | Healthcare Automation |
Replika | Virtual AI companion, emotional support, conversational engagement for loneliness and social isolation. | Chatbot |
Talkspace | AI-powered therapist matching, text/audio/video therapy sessions, licensed mental health professionals. | Professional Therapy Platform |
Wysa | AI-driven chatbot for mental health support, CBT-based self-help tools, stress and anxiety management. | Chatbot |
Youper | Personalized AI-guided therapy, CBT/ACT/DBT integration, mood tracking, self-reflection. | Chatbot |
AI for mental healthcare use cases
Early detection of mental health disorders
1. Diagnosis with text analysis
Advancements in AI-driven tools have significantly enhanced the ability to detect mental health disorders at an early stage by analyzing speech, text, and facial expressions. NLP techniques extract valuable insights from both spoken and written language, enabling sentiment analysis to detect subtle emotional changes through social media posts, chat interactions, or personal diaries. By assessing shifts in tone, word choice, and phrasing, these tools can identify patterns indicative of mental health conditions such as depression and anxiety.
2. Diagnosis with voice recognition
In addition to text analysis, voice recognition technology can detect variations in speech, including fluctuations in pitch, tone, and rhythm, which may signal underlying mental health concerns. These vocal biomarkers can serve as early indicators of mental illnesses like major depressive disorder (MDD) and post-traumatic stress disorder (PTSD).
3. Diagnosis with facial expression analysis
Similarly, facial expression analysis plays a critical role in assessing mental well-being. AI algorithms detect micro-expressions and minor shifts in facial features, helping to identify distress or other psychological conditions. This capability is particularly beneficial for remote mental health monitoring through mental health apps, video consultations, and mobile platforms.
4. Diagnosis with electronic health records analysis
Beyond speech and facial recognition, AI helps in analyzing electronic health records (EHRs) for the early diagnosis of mental disorders. Machine learning algorithms can process extensive patient data, including medical history, clinical notes, and diagnostic tests, to identify risk factors for mental health conditions. These AI models help flag high-risk patients, allowing mental health professionals to intervene sooner and integrate mental health data into a broader healthcare plan.
Real-life example:
Cogito2 leverages advanced artificial intelligence to enhance mental healthcare by providing real-time emotional intelligence and conversational analysis tools:
- Real-time emotional intelligence coaching: Cogito’s platform analyzes voice signals during patient interactions, offering live guidance to care managers. This assists them in displaying empathy, building trust, and improving patient engagement, which is crucial for successful mental health interventions.
- Behavioral health monitoring: The platform passively and securely collects behavioral data through mobile applications, analyzing it against clinical mental health assessment criteria. This continuous monitoring aids in the early detection of behavioral health issues, enabling timely interventions.
5. Predictive modeling in mental health
AI is increasingly being used to develop predictive models for mental health, considering factors like genetics, environmental influences, lifestyle choices, and social determinants of health. By analyzing these variables, AI can predict an individual’s risk of developing a mental health condition. For instance, genetic data can highlight predispositions, while external factors like trauma or social isolation refine the accuracy of predictions.
The integration of wearable devices and mobile health apps is enhancing predictive models by providing real-time data on behaviors such as physical activity, sleep, and social interactions, making these models even more accurate.
Real-life example:
Headspace, previously Ginger and Headspace Health, use predictive analytics to identify individuals at risk of mental health conditions by monitoring behaviors such as increased stress, sleep disturbances, and social withdrawal.
When patterns suggest a potential issue, the platform proactively reaches out, offering additional support, like connecting users with mental health coaches.3
Mental health treatment
6. Personalized treatment plans
AI has introduced a data-driven approach to mental health therapy, enabling highly personalized treatment plans. By leveraging machine learning algorithms, AI can analyze an individual’s unique characteristics—including genetic predispositions, past treatment responses, behavioral patterns, and real-time physiological data. This analysis allows AI to tailor treatment recommendations, ensuring that each patient receives the most effective intervention suited to their specific needs.
One of the most promising applications of AI-driven mental health care is in medication management. AI models can evaluate a patient’s genetic profile to predict their response to antidepressants or other psychiatric medications.
For instance, individuals with a particular genetic makeup may respond more favorably to a specific class of antidepressants, while others may experience adverse side effects. By optimizing medication selection, AI-driven treatment minimizes trial-and-error prescribing, reducing side effects and increasing the likelihood of positive mental health outcomes.
7. AI-assisted therapy
AI is also transforming how mental health therapy evolves throughout a patient’s journey. Rather than relying solely on periodic assessments, AI-driven systems continuously analyze patient progress, adjusting treatment plans in real time based on shifting mental health symptoms and responses. This reduces the reliance on trial-and-error methods commonly seen in psychological practice, ensuring a more efficient and effective therapeutic process.
For example, AI-enhanced Cognitive Behavioral Therapy (CBT) can modify treatment techniques according to a patient’s unique cognitive processes. If an individual displays perfectionist tendencies, the AI can adapt CBT strategies to directly address these patterns, ensuring a more personalized and impactful intervention.
Real-life example:
Wysa offers emotional support and self-help tools based on cognitive behavioral therapy (CBT) techniques:
- Psychologist-designed, therapist-assisted AI CBT programs for anxiety and other mental health concerns.
- Each program includes 6-8 modules with psychoeducational videos, interactive CBT techniques, and daily check-ins to boost engagement.
- Tracks clinical recovery and NHS KPIs, aligning with mandated targets.
- Integrates with EPRs, reducing clinician workload and ensuring data security.
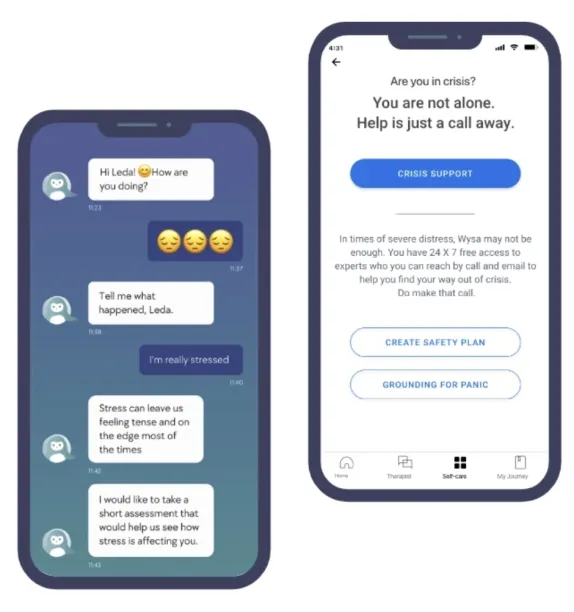
Figure 1: Wysa dashboard for AI assisted CBT program.4
AI can also suggest alternative interventions if a patient isn’t responding well to a particular approach, recommending more suitable strategies based on the patient’s profile and progress. This enables therapists to tailor treatments more precisely.
Moreover, AI helps therapists by automating administrative tasks like scheduling and billing, allowing them to focus more on the therapeutic aspects of their work. This collaboration enhances treatment effectiveness and optimizes mental health outcomes.
Real-life example:
Talkspace is a mental health platform that leverages AI to match users with licensed therapists based on individual needs, preferences, and the nature of their concerns. It creates a personalized experience by using algorithms to analyze data like communication style, therapeutic approaches, and specialty areas to ensure the best possible match between user and therapist.
Once matched, Talkspace facilitates therapy sessions in multiple formats, including text, audio, and video messages, allowing users to choose the mode that feels most comfortable for them.
Additionally, Talkspace is designed to provide continuous support, with users able to communicate with their therapists asynchronously, at their own pace, whenever they need guidance or simply someone to talk to.5
Read healthcare AI use cases for more.
What is AI for mental health?
AI for mental health refers to the application of artificial intelligence (AI), computational technologies, and algorithms to improve the understanding, diagnosis, and treatment of mental health disorders. As AI becomes increasingly integrated into daily life, it is making its way into the mental healthcare space, transforming the way mental health challenges are addressed.
The application of AI in mental health encompasses several key areas, such as identifying and diagnosing mental disorders, analyzing electronic health records (EHRs), creating personalized treatment plans, and utilizing predictive analytics for initiatives like suicide prevention.
What are the key components of AI for mental health?
Supervised Machine Learning (SML) for mental health diagnosis
In mental health diagnosis, Supervised Machine Learning (SML) relies on pre-labeled data to differentiate between conditions, such as major depressive disorder (MDD) and the absence of depression.
The algorithm learns to recognize patterns in diverse data sources, including electronic health records, sociodemographic factors, biological markers, and clinical assessments. By establishing relationships between these features, SML enhances the accuracy of diagnosing mental health conditions and predicting mental health outcomes.
The algorithm is trained with labeled datasets and later tested on unseen data to ensure its capability in accurately classifying mental health conditions. This method supports mental health professionals in making informed decisions regarding mental health treatment and intervention.
Unsupervised Machine Learning (UML) for discovering new insights
Unlike SML, Unsupervised Machine Learning (UML) operates without predefined labels, allowing it to detect hidden patterns within vast datasets. This capability is especially useful in uncovering subtypes of mental disorders, such as schizophrenia, through neuroimaging biomarkers.
UML helps identify clusters of biomarkers, facilitating early identification of psychiatric conditions and aiding in tailored mental health therapy approaches. By analyzing large-scale datasets, UML contributes to personalized mental health care by refining treatment outcomes and improving patient outcomes.
Artificial Neural Networks (ANNs) and Deep Learning (DL)
Inspired by the cognitive processes of the human brain, Artificial Neural Networks (ANNs) and Deep Learning (DL) enable AI to process raw, complex data with minimal human intervention.
These AI models excel in analyzing brain imaging data from MRI and CT scans, identifying structural abnormalities associated with mental illnesses.
Additionally, DL enhances mental health applications by improving speech and text recognition, facilitating early detection of mental health issues, and supporting automated mental health diagnosis.
Natural Language Processing (NLP) for mental health monitoring
Natural Language Processing (NLP) plays a crucial role in analyzing mental health symptoms by processing and interpreting human language in real-time. NLP-powered AI tools assess written or spoken language to detect emotional states and behavioral changes, assisting mental health professionals in monitoring patients’ well-being.
Additionally, AI-driven virtual therapists use NLP to engage users in text-based dialogues, offering mental health support, coping strategies, and referrals to mental health services when needed.
NLP is also widely applied in analyzing mental health information from social media, identifying early warning signs of distress, and facilitating early intervention for individuals at risk.
Multiple studies6 suggest that NLP-powered chatbots can effectively detect mental health issues by employing a structured, question-based approach similar to that used by mental health practitioners.
These chatbots assess key aspects such as mood, stress levels, energy, and sleep patterns. By analyzing user responses, the system can recommend appropriate interventions, ranging from simple behavioral modifications—such as walking, meditation, or relaxation exercises—to suggesting professional mental health support when necessary.
Moreover, if the chatbot detects an urgent risk to the individual’s safety, it can immediately alert a healthcare provider, ensuring timely intervention.
AI chatbots, especially those with voice features, are increasingly perceived as human-like, prompting more users to seek emotional support and companionship from them.
However, this raises concerns about potential effects on loneliness and real-world social interaction.
A four-week randomized, controlled study (981 participants, over 300,000 messages) examined how different chatbot interaction styles (text, neutral voice, expressive voice) and conversation types (open-ended, non-personal, personal) influence psychological outcomes such as loneliness, emotional reliance on AI, and social engagement.7
Key findings include:
- Voice-based chatbots were initially more effective than text in reducing loneliness and dependence, but these benefits declined with heavy use—especially when the chatbot used a neutral voice.
- Chatting about personal topics slightly increased loneliness but lowered emotional dependence compared to open-ended conversations.
- Non-personal conversations led to greater emotional dependence among frequent users.
- Overall, increased daily usage—regardless of mode or topic—was linked to more loneliness, higher dependence on AI, more problematic usage, and reduced socialization with real people.
- Users with stronger emotional attachment traits or higher trust in AI were more prone to loneliness and emotional dependence.
Reinforcement Learning (RL) for personalized mental health therapy
Reinforcement Learning (RL) enables AI systems to adapt dynamically to patient responses, making it valuable for personalized mental health therapy.
RL-powered AI technology is used in mental health apps such as virtual reality exposure therapy, where the AI agent adjusts treatment intensity based on patient reactions. This ensures a balance between progress and distress reduction, optimizing therapy sessions and treatment outcomes. Over time, RL fine-tunes its approach, enhancing the efficacy of mental health interventions.
Computer Vision for emotion recognition and mental health assessment
Computer Vision powered applications interprets visual data to detect facial expressions and body language, providing insights into mental health conditions.
By analyzing microexpressions and gestures, AI-powered systems can assess emotional states and identify mental health concerns such as depression and anxiety. This technology is particularly useful in post-traumatic stress disorder (PTSD) detection, mental health crisis assessment, and autism spectrum disorder (ASD) support.
Additionally, computer vision aids in telehealth by enabling remote patient monitoring, helping mental health providers assess individuals who may not have access to in-person mental healthcare.
Challenges of integrating AI into mental healthcare
AI has the potential to improve mental healthcare, from diagnostics to treatment. However, its integration comes with significant challenges that must be addressed to ensure ethical and effective use:
1. Ethical and regulatory challenges
The lack of clear guidelines for AI in mental healthcare is a major concern. Although some regulations, like those from the FDA, are starting to take shape, there’s still no universally accepted framework.
2. Maintaining the human element in therapy
AI should enhance, not replace, human therapists. While most tools are designed to support therapy between sessions, keeping the human touch in treatment is essential. Patients must also be aware when AI is part of their therapy, to ensure transparency and build trust.
3. Privacy and data security
Mental health data is highly sensitive. AI tools in mental healthcare require strong security measures to protect patient data from breaches.
AI systems must also comply with privacy laws like HIPAA to maintain confidentiality. Patients should have control over their data and understand how it will be used.
4. Bias and fairness
AI can perpetuate biases present in training data, leading to unfair treatment for certain groups. If AI tools are trained on data from only specific demographics, they may not be accurate for others, resulting in misdiagnosis or ineffective treatment.
To address this, diverse datasets and regular audits are needed to ensure fairness in AI decision-making.
5. Reliability and accountability
AI tools in mental health must be able to handle the complexity of diagnosing disorders, which often require understanding a patient’s background and personal experiences. AI can produce false positives or miss important details, and its algorithms need to stay updated with the latest research to remain accurate.
When AI tools are involved in making treatment decisions, it can be unclear who is responsible if something goes wrong. Determining accountability in cases of misdiagnosis or harm is complex and requires clear guidelines about who holds the responsibility, whether developers, clinicians, or institutions.
Further reading
- Generative AI Healthcare: 15 Use Cases, Challenges & Outlook
- Healthcare APIs: Top 7 Use Cases & Challenges with Examples
External Links
- 1. Additional depression and anxiety cases due to COVID-19 worldwide 2020| Statista. Statista
- 2. Contact Center AI | Healthcare | Cogito. Cogito Corp
- 3. Headspace for Organizations | Formerly Headspace Health and Ginger.
- 4. Wysa AI Support for NHS Talking Therapies | Enhance Care and Support Better Outcome For Service Users.
- 5. Effective, Affordable Online Therapy & Counseling » Try Talkspace.
- 6. Artificial intelligence in positive mental health: a narrative review - PMC .
- 7. How AI and Human Behaviors Shape Psychosocial Effects of Chatbot Use: A Longitudinal Controlled Study — MIT Media Lab.
Comments
Your email address will not be published. All fields are required.