Predictive analysis was ranked among the top 10 process mining capabilities in last year’s process mining trends.
Predictive process mining enables businesses to maintain their processes running smoothly by predicting possible outcomes, issues and steps in processes.
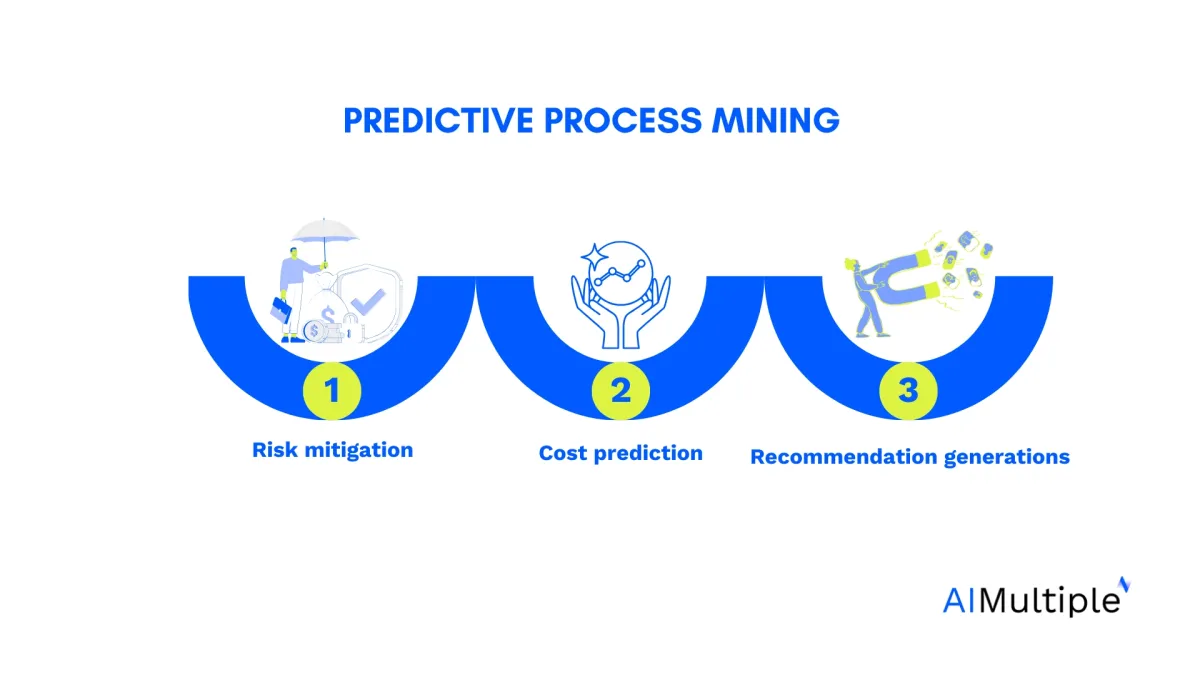
Explore how predictive process mining works, its types and top use cases:
What is predictive process mining?
Predictive process mining is a process mining method that leverages predictive analytics to predict the time and outcomes of ongoing cases by analyzing historical data. For example, in continuous sales processes, the result would be either finalizing the purchase or not.
Some of the capabilities of predictive process mining include:
- Labeling normal or deviant case
- Estimating activity duration and the time remaining to accomplish a task
- Predicting following activity
- Forecasting possible outcome.
Leading vendors leverages ML algorithms for predictive analytics within their process mining solutions to help their customers predict possible bottlenecks and improve scenario capabilities.
What is predictive process monitoring?
Predictive process monitoring is an advanced subfield of Process Mining that focuses on forecasting future events, outcomes, or performance measures in ongoing business processes using historical event data. Some sources use predictive process monitoring and mining interchangeably.
Predictive monitoring of business processes include applications like:
- The sequence of future events
- Remaining execution time
- Case outcomes.
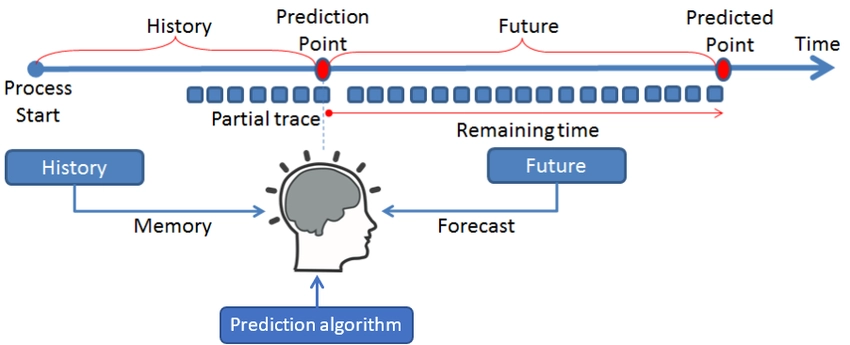
By integrating predictions into decision-making processes, predictive process monitoring enables organizations to optimize resources, improve operational efficiency, and respond proactively to challenges.
Predictive process mining use cases
1. Risk mitigation
Predictions allow businesses to mitigate risks before any harm is done. Predictive process mining enables the discovery of potential issues and the reasons behind them. It can leverage either risk prediction or time prediction categories:
- Risk predictions are categorial predictions that estimate and inform about risk levels in decision-making processes by configuring process risk indicators and examining historical data.
- Time predictions are numeric predictions that are applied to measure the delays or remaining time in a continuing process.
For example, logistics firms can leverage predictive process mining to detect the delays in the delivery of goods and can identify their root cause. Once the delays are classified depending on the root cause, predictive analytics can alert analysts of risk levels for areas or goods that are expected to have a late delivery.
Real-life example
Brussels Airport used predictive process monitoring to optimize its baggage handling system, focusing on bags causing delays and high costs. The airport struggled with complex baggage routes and large data sets, affecting prediction accuracy. A stacked model combining Random Forest and LSTM neural networks was developed to improve completion time predictions, balancing interpretability and precision.
Results:
- Reduced mean absolute error (MAE) to 56 minutes.
- Improved prediction accuracy by 5 minutes compared to static models.
- Identified key factors affecting baggage delays (e.g., flight schedules, time of arrival).
- Enabled proactive measures to minimize delays and operational costs.2
2. Cost prediction
Businesses can leverage cost prediction to help identify present necessities and shortcuts based on insights gained from historical data. Cost prediction is a numeric prediction that consider production, volume and time to predict the cost of the ongoing process. As a result, it can reduce costs once businesses implement the shortcuts or correspond to the needs indicated by predictions.
For example, manufacturing companies can apply cost prediction method to forecast the cost of ongoing process considering production time and volume.
3. Recommendation generations
Businesses can leverage predictive process mining to generate recommendations by forecasting future activities and outcomes. For example:
1. Next- activities
Predictive process mining can be used for next-activity predictions in ongoing cases, estimating the sequence of future events and their probabilities. Analysts can apply deep learning by converting event logs into words and using natural language processing to classify process instances, improving prediction accuracy.
For example, this method can be implemented in automobile insurance to estimate the potential fraudulent claims. To do so, predictive process mining tools profile customers and categorize them by leveraging the insights discovered from customer behavior and organizational process data.
2. Case outcomes
Predictive process mining can predict outcomes for all cases considering key performance indicators (KPI). This type of predictive process mining is named as outcome predictions which analyzes the sequence of events that are already performed in the same case and the latest activity from the current case. Based on these outcomes, it provides suggestions for the following events for each case.
For example, customer satisfaction is a major KPI for many industries. A bank that can predict an issue in front and back offices can react in time to the issues and can improve their customer satisfaction significantly. Another example, healthcare facilities can leverage predictive process mining to estimate the failing cases in staff planning and optimize their strategy.
Predictive process mining solutions
Predictive process mining leverages different machine learning algorithm, such as:
Supervised machine learning algorithm
Supervised ML algorithms that support vector machines can be trained on historical data in order to predict the final result. These algorithms’ results can be easy to predict as the algorithm provides in detail the steps taken to obtain the final result.
Some of these algorithms include:
- Support vector machines (SVM): Used for classification and predictive tasks in complex workflows.
- Regression models: Predicts outcomes such as cycle times, costs, and risks.
- Decision trees and random forests: Predict process outcomes and classify process instances based on historical data.
- Clustering algorithms: Groups similar process variants to enable targeted predictive insights.
Unsupervised machine learning
Unsupervised machine learning algorithms such as deep learning and neural networks have a black box approach for predicting the final results, making it harder for process mining analysts to understand the root cause of a prediction. However, despite the difficulty, studies have shown that deep-learning-based models are more successful at predicting accurate results compared to supervised machine learning models.3
Some of the deep learning models used in predictive process mining includes:
- Recurrent Neural Networks (RNNs): For sequence-based prediction tasks like activity prediction.
- Long Short-Term Memory Networks (LSTMs): Specialized for predicting the next steps or completion times in processes with temporal dependencies.
The future of predictive process mining
The interest over predictive process mining has increased in academia over the years. Recently, it became a common feature that all top process mining vendors deliver as Gartner identifies.4
Process mining tools aim to deliver what-if process mining and prescriptive process monitoring, leading to fully automated process mining tools in the long-run.
Predictive process mining vs predictive process monitoring
Since predictive process monitoring is a subfield of process mining, they have various similarities in their approach. Yet, they differ in terms of scope, purpose and focus.
Similarities
Both of them:
- Use historical event data and predictive analytics
- Leverage machine learning and statistical methods
- Aim to identify patterns in process execution to foresee future outcomes (e.g., next activity, time predictions, or risks).
- Facilitate data-driven decision-making to optimize business processes.
Differences
- Scope:
- Predictive process mining focuses on entire business processes, analyzing trends, bottlenecks, and inefficiencies.
- Predictive process monitoring focuses on specific ongoing process instances, providing real-time predictions.
- Purpose:
- Process mining informs strategic, long-term decisions and overall process redesign.
- Process monitoring supports operational, case-level decisions during process execution.
- Focus:
- Process mining addresses “what happened” and “why.”
- Process monitoring addresses “what will happen next” in a specific context.
Which one to choose?
- Predictive process mining is better suited for long-term, strategic improvements because it offers a systemic, comprehensive understanding of process flows and historical performance.
- Predictive process monitoring is ideal for agile, case-specific operational decision-making because it provides more immediate, real-time predictions that allow for quick interventions.
Further Reading
To learn more about process mining use cases, machine learning and AI applications, feel free to read our in-depth articles:
- 33 Use Cases and Applications of Process Mining
- Top 6 Applications of Machine Learning (ML) in Process Mining
- Top 4 Use Cases of AI-enabled Process Mining
If you believe your business can benefit from process mining tools, you can check our data-driven list of process mining software and other automation solutions.
Check out our comprehensive and constantly updated process mining case studies list to understand predictive process mining with real-life examples.
And you can let us find you the right vendor:
External sources
- 1. ResearchGate - Temporarily Unavailable.
- 2. ResearchGate - Temporarily Unavailable.
- 3. ResearchGate - Temporarily Unavailable.
- 4. Gartner Login.
- 5. Spree, F.”Predictive Process Monitoring: A Use-Case-Driven Literature Review.“Technical University of Sofia. Retrieved at January 23, 2025.
Comments
Your email address will not be published. All fields are required.