AI Transformation in 2024: In-Depth guide for executives
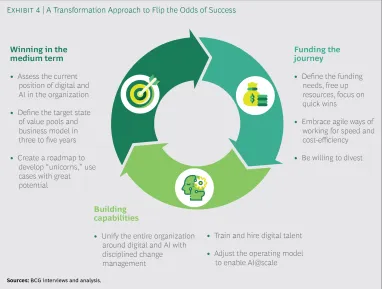
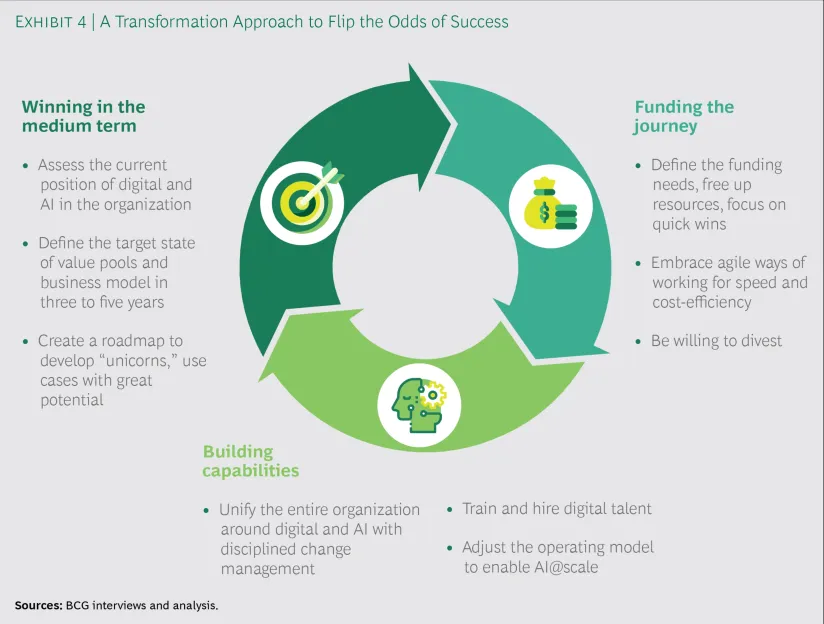
AI transformation is the next phase of digital transformation. Businesses are willing to invest in AI technologies to stay ahead of competitors. According to Andrew Ng, AI Transformation is a process that may take 2-3 years, but organizations can start to see the returns within 6 to 12 months.
Digital transformation is required before companies can start their AI transformation because digital data is necessary for AI training and digital processes are necessary to roll-out AI solutions in most cases. Feel free to read about what digital transformation is and our extensive digital transformation guide if you believe that your company has not yet progressed on its digital transformation journey.
What is AI Transformation?
AI transformation is the next step after digital transformation. After a company adopts digital processes, the next step is to improve the intelligence of those processes. This would increase the level of automation as well as the effectiveness of those processes.
AI transformation touches all aspects of the modern enterprise including both commercial and operational activities. Tech giants are integrating AI into their processes and products. For example, Google is calling itself an “AI-first” organization. Besides tech giants, IDC estimates that at least 90% of new organizations will insert AI technology into their processes and products by 2025.
What are the steps to AI transformation?
We have listed below a set of the top 6 steps for Fortune 500 firms. Smaller firms could skip having in-house teams and strive for less risky and less investment heavy approaches such as relying on consultants for targeted projects.
1. Outline your company’s AI strategy
An AI strategy should include initiatives which will be uncovered as a result of these exercises:
- Identify your company’s most valuable unique data sources
- Identify the most important processes which can benefit from automation
- Identify internal resources to drive the AI transformation
- Set ambitious, time-bound business targets
2. Execute pilot projects to gain momentum
First few projects should create measurable business value while being attainable. This is important for the transformation to gain trust across the organization with achieved projects and it creates momentum that will lead to AI projects with greater success.
These projects can rely on AI/ML powered tools in the marketplace or for more custom solutions, your company can run a data science competition and rely on the wisdom of hundreds of data scientists. These competitions use encrypted data and provide a low cost way to find high performing data science solutions.
Implementing process mining is one of those easy-to-achieve and impactful projects. With a process mining tool, your business can identify existing inefficiencies and automate or improve those processes to achieve savings or customer experience improvement. Thus, some process mining tools generate a digital twin of an organization (DTO) which provides an end-to-end overview of the processes in the company and offers simulation capabilities to compare actual and hypothetical scenarios.
Another easy-to-deploy and impactful project is automating document based processes. While digital transformation projects in the 2000s just dealt with removing paper from processes, a modern AI/digital transformation project would reduce manual labor and automate data extraction and processing of document data.
3. Build an in-house AI transformation team
Outsourcing the AI work eases the start of the AI transformation process but building an in-house AI transformation team can be more advantageous in the long run. If necessary, outsourced partners can help train your staff for upcoming projects.
4. Provide broad AI training
Organizations should not expect adequate knowledge about AI technologies from their staff. In order to have a successful AI transformation, training each employee in accordance with their role can be beneficial to achieve objectives.
- Executives and seniors should have knowledge about what AI can do for the enterprise, how to develop an AI strategy and make proper resource allocation decisions.
- Leaders of AI project teams should learn how to set direction for AI projects, allocate resources, monitor and track progress.
- AI engineers should learn how to gather data, train AI models, and deliver specific AI projects.
5. Develop internal and external communications
For the road to success in AI transformation, the organization should ensure alignment across the business by improving internal and external communication.
6. Update the company’s AI strategy and continue with AI transformation
When the team gains momentum from the initial AI projects and forms a deeper understanding of AI, the organization will have a better understanding of improvement areas where AI can create the most value. An updated strategy that takes into account the company’s track record can set a better direction for the company.
Thanks to Andrew Ng for outlining the step of AI transformation steps in his AI Transformation Playbook. We expanded his list with an initial target setting step.
Which industries are most available for AI transformation?
Artificial Intelligence is starting to have a major impact on markets. Industries such as automotive, high-tech, communication, energy and financial services have already covered a substantial amount of ground in the AI adoption process. Such differences are likely to persist as more digitized industries are likely to take better advantage of AI technologies.
According to O’Reilly AI Adoption in the Enterprise 2021 survey,
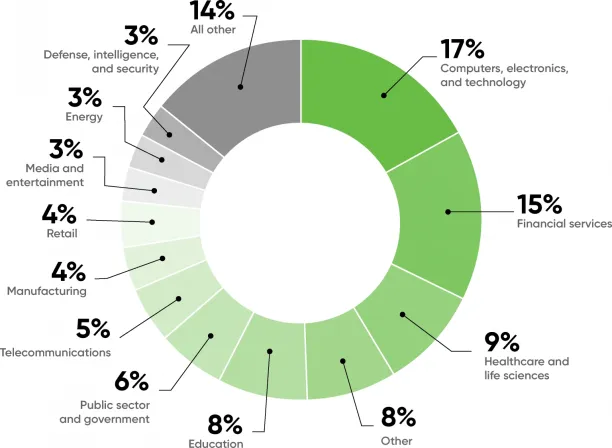
What are the obstacles to AI transformation?
According to the same survey from O’Reilly, the top obstacles facing AI transformation are:
- Insufficient AI talent and experience in the organization.
- Data quality issues and lack of sufficient data
- Difficulties in identifying applicable business use cases
- Company culture does not recognize the value of AI
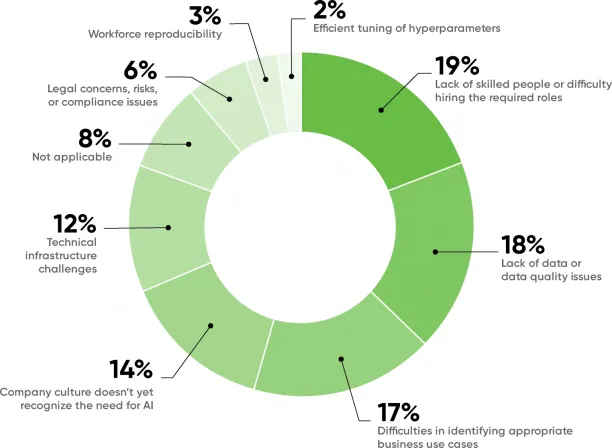
What are the best practices?
Based on our review of existing research and interviews:
- AI initiatives should not be limited to investments in technology alone. Process and people aspects of transformation should not be underestimated.
- Strategy cannot be sacrificed on the altars of AI deployment. AI investments should be in line with company’s business goals to achieve business impact
- Deploying Artificial Intelligence across business operations requires restructuring of the entire technology strategy and infrastructure in the organization
- Professionals who are proficient in machine learning and artificial intelligence deployments should lead the AI functions.
What are recent case studies?
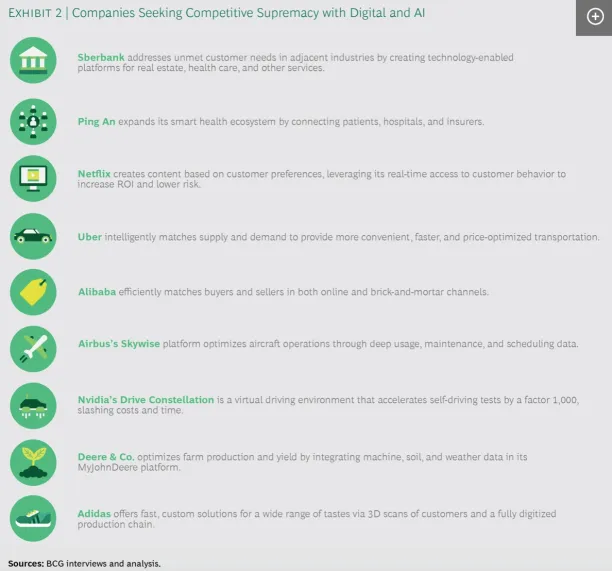
For more on AI
Feel free to check our other AI articles to learn more about how AI can transform your business:
- AI in Automation: Which tasks can we automate?
- Top AI Use Cases / Applications
- State of AI technology: Comprehensive Guide
If you have a data source which can be used to build a machine learning model and improve your business’ performance, don’t hesitate to contact us:
If you want to learn more about custom AI solutions, feel free to read our whitepaper on the topic:
We can also help if you have questions about how AI transformation can impact your business and how you can get started:

Cem has been the principal analyst at AIMultiple since 2017. AIMultiple informs hundreds of thousands of businesses (as per similarWeb) including 60% of Fortune 500 every month.
Cem's work has been cited by leading global publications including Business Insider, Forbes, Washington Post, global firms like Deloitte, HPE, NGOs like World Economic Forum and supranational organizations like European Commission. You can see more reputable companies and media that referenced AIMultiple.
Throughout his career, Cem served as a tech consultant, tech buyer and tech entrepreneur. He advised businesses on their enterprise software, automation, cloud, AI / ML and other technology related decisions at McKinsey & Company and Altman Solon for more than a decade. He also published a McKinsey report on digitalization.
He led technology strategy and procurement of a telco while reporting to the CEO. He has also led commercial growth of deep tech company Hypatos that reached a 7 digit annual recurring revenue and a 9 digit valuation from 0 within 2 years. Cem's work in Hypatos was covered by leading technology publications like TechCrunch and Business Insider.
Cem regularly speaks at international technology conferences. He graduated from Bogazici University as a computer engineer and holds an MBA from Columbia Business School.
To stay up-to-date on B2B tech & accelerate your enterprise:
Follow onNext to Read
Generative AI Data in 2024: Importance & 7 Methods
Great insight on AI and the transformation progression. I found the industries currently impacted interesting also.
Comments
Your email address will not be published. All fields are required.