Advancements in deep learning techniques have paved the way for successful computer vision and image recognition applications in fields such as automotive, healthcare, and security. Computers that can derive meaningful information from visual data enable numerous applications such as self-driving cars and highly accurate detection of diseases.
The challenge with deep neural networks and their applications in computer vision is that these algorithms require large, correctly labeled datasets for better accuracy. Collecting and annotating significant amounts of high-quality photos and videos to train a deep-learning model is time-consuming and expensive.
Synthetic (i.e., artificially generated) images and videos can solve both the collection and annotation problems of working with visual data.
How can synthetic data help computer vision?
Enables creating datasets faster and cheaper
Collecting real-world visual data with desired characteristics and diversity can be prohibitively expensive and time-consuming. After collection, annotating data points with correct labels is crucial because mislabeled data would lead to inaccurate model outcomes. These processes can take months and consume valuable business resources. For more on image annotation, follow the link.
Synthetic data is generated programmatically which means it does not require manual data collection efforts and it can contain nearly perfect annotations. The image below by Unity demonstrates the difference between computer vision projects with real data and synthetic data. Unity states that they created a better model while saving about 95% in both time and money.
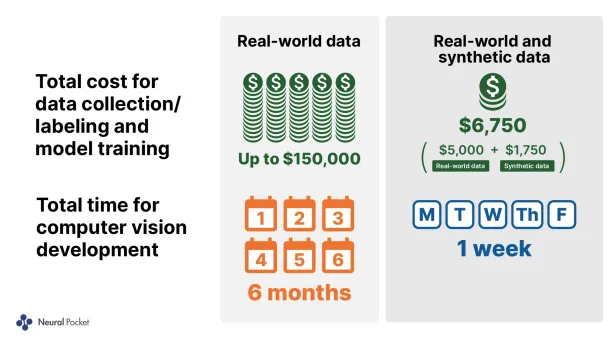
Enables rare event prediction
Datasets collected from real-world are often imbalanced which means some events are rarer than others. However, this does not mean they are negligible. For example, the computer vision system of a self-driving car that learns from road events may lack enough examples of car accidents because collecting visual data for it is difficult. Rare diseases or counterfeit money are some other examples of rare events that can be encountered in computer vision applications.
Instead, training deep learning algorithms of self-driving cars with synthetic images or videos of car accidents under a diverse set of circumstances (different times of day, number of vehicles, types of vehicles, number of pedestrians, environment, etc.) can enable safer and more reliable autonomous vehicles.
Thus, synthetic data offers a way to generate datasets that represent the diversity of real-world events more accurately.
Prevents data privacy problems
Collecting and storing visual data is also challenging because of data privacy regulations such as GDPR. Non-compliance with such regulations can lead to serious fines and damage business reputation. Working with datasets that contain sensitive information has its risks because data breaches can occur even through model outcomes. For example, researchers managed to extract recognizable face images from the training set with only API access to the facial recognition system and person’s name.
Synthetic data eliminates the risks of privacy violations because a synthetic dataset would not contain information about real persons while preserving the important characteristics of a real dataset.
What are some case studies?
- Caper is a startup making intelligent shopping carts that enable customers to shop without waiting in checkout line. Image recognition model deployed in their shopping carts requires 100 to 1000 images for each item and there can be thousands of different items in a store. Caper used synthetic images of store items that capture different angles and trained the deep learning algorithm with it. The company states that their shopping carts have 99% recognition accuracy.
- NVIDIA created a robotics simulation application and synthetic data generation tool called Isaac Sim for developing, testing, and managing AI-based robots working in real world.
- Training an object detector with synthetic images containing random objects and non-realistic scenes is showed to improve deep neural network model performance. The technique is called domain randomization and researchers conclude that the real world may appear to the model as just another variation. The object detector could locate physical objects in a cluttered environment with 1.5 cm accuracy.
If you want to learn more about synthetic data and its applications, check our other articles on the topic:
- Top 20 Synthetic Data Use Cases & Applications
- Synthetic Data Generation: Techniques, Best Practices & Tools
If you are looking for synthetic data generation software, check our data-driven, sortable/filterable list of vendors.
Comments
Your email address will not be published. All fields are required.