Synthetic data offers solutions to common challenges in data science, including data privacy concerns and limited dataset sizes. Synthetic data is gaining widespread popularity and applicability across industries, including machine learning, deep learning, generative AI (GenAI), and large language models. We listed the capabilities and most common use cases of synthetic data in different industries and departments/business units.
What are industry-agnostic use cases enabled by synthetic data?
Data sharing with third parties
- Innovation in many sectors relies on partnering with third-party organizations such as fintechs or medtechs. Synthetic data enables enterprises to evaluate third-party vendors and share private data with them without security or compliance risks. You might want to check supply chain data sharing if you want to learn more about data sharing.
Internal data sharing
- Data privacy regulations not only restrict data sharing between organizations but also prevent the flow of data within an organization. Getting data access permissions can take weeks which can hinder collaboration. Organizations can speed up innovation with enhanced collaboration between teams by leveraging synthetic data.
Cloud migration
- Cloud services offer a range of innovative products for many sectors. However, moving private data to cloud infrastructures involves security and compliance risks. In some cases, moving synthetic versions of sensitive data to the cloud can enable organizations to take advantage of the benefits of cloud services. This is not possible for all use cases. For example,
- It wouldn’t be useful for salespeople to have synthetic data in their CRM, they should see the correct customer information not modified information.
- In cloud machine learning pipelines, synthetic data could be used instead of real data
Data retention
- Synthetic data provides a way to comply with data retention regulations without undermining long-term analytics capabilities. Regulations also limit how long a business can store personal data. This is a problem for long-term analyses such as detecting the seasonality of data over several years
You can refer to our data governance tools article to get an overview of the tools offered.
What are synthetic data use cases in different industries and sectors?
Financial services
- Fraud identification is a major part of any financial service, but fraudulent transactions are rare. With synthetic fraud data, new fraud detection methods can be tested and evaluated for their effectiveness.
- Customer intelligence: Synthetic customer transaction data can be used to perform analysis on customer data to understand customer behavior. This is similar to the use case on “internal data sharing” however it is applicable more widely in finance where most customer data is private.
Refer to our article for more information on the use cases of synthetic data in finance.
Manufacturing
- Quality assurance: It is hard to test a system to see whether it identifies anomalies since there are infinitely many anomalies. Synthetic data enables more effective testing of quality control systems, improving their performance. As Leo Tolstoy states at the beginning of Anna Karenina: “All happy families are alike; each unhappy family is unhappy in its own way.”
Healthcare
- Healthcare analytics: Synthetic data enables healthcare data professionals to allow the internal and external use of record data while still maintaining patient confidentiality. This is similar to the use case on “internal data sharing” however it is applicable more widely in healthcare where most customer data is private.
- Clinical trials: Synthetic data can be used as a baseline for future studies and testing when no real data yet exists.
Automotive and Robotics
Autonomous Things (AuT): Research to develop autonomous things such as robots, drones, and self-driving car simulations pioneered the use of synthetic data. This is because real-life testing of robotic systems is expensive and slow. Synthetic data enables companies to test their robotics solutions in thousands of simulations, improving their robots and complementing expensive real-life testing.
- Self-driving cars
- Autonomous robots
Security
Synthetic data can be used to secure organizations’ online & offline properties. Two methods are commonly used:
- Training data for video surveillance: To take advantage of image recognition, organizations need to create and train neural network models, but this has two limitations: Acquiring the volumes of data and manually tagging the objects. Synthetic data can help train models at a lower cost compared to acquiring and annotating training data.
- Deep fakes: Deepfakes, which are becoming an increasingly important AI cybersecurity topic, can be used to test face recognition systems.
Social Media
Social networks are using synthetic data to improve their various products:
- Testing content filtering systems: Social networks are fighting fake news, online harassment, and political propaganda from foreign governments. Testing with synthetic data ensures that the content filters are flexible and can deal with novel attacks.
What are synthetic data use cases in specific departments or functions?
Agile development and DevOps
- For software testing and quality assurance, artificially generated data is often the better choice as it eliminates the need to wait for ‘real’ data. Often referred to under this circumstance as ‘test data’. This can ultimately lead to decreased test time and increased flexibility and agility during development
HR
- Employee datasets of companies contain sensitive information and are often protected by data privacy regulations. In-house data teams and external parties may not have access to these datasets but they can leverage synthetic employee data to conduct analyses. It can help companies to optimize HR processes.
Marketing
- Synthetic data allows marketing units to run detailed, individual-level simulations to improve their marketing spend. Such simulations would not be allowed without user consent due to GDPR. However synthetic data, which follows the properties of real data, can be reliably used in simulation.
Machine learning
- Most ML models require large amounts of data for better accuracy. Synthetic data can be used to increase training data size for ML models.
- Prediction of rare events such as fraud or manufacturing defects is hard since small data size leads to inaccuracies for ML models. Generating synthetic instances of such events increases model accuracy.
- Synthetic data generation creates labeled data instances, ready to be used in training. This reduces the necessity for time-consuming data labeling efforts.
Synthetic data has emerged as a crucial asset across various industries, with wide-ranging applications. Its popularity (Figure 1) is driven by its ability to replicate real-world data with high accuracy, while simultaneously addressing data privacy concerns and reducing costs associated with data collection.
Figure 1: Popularity of Synthetic Data
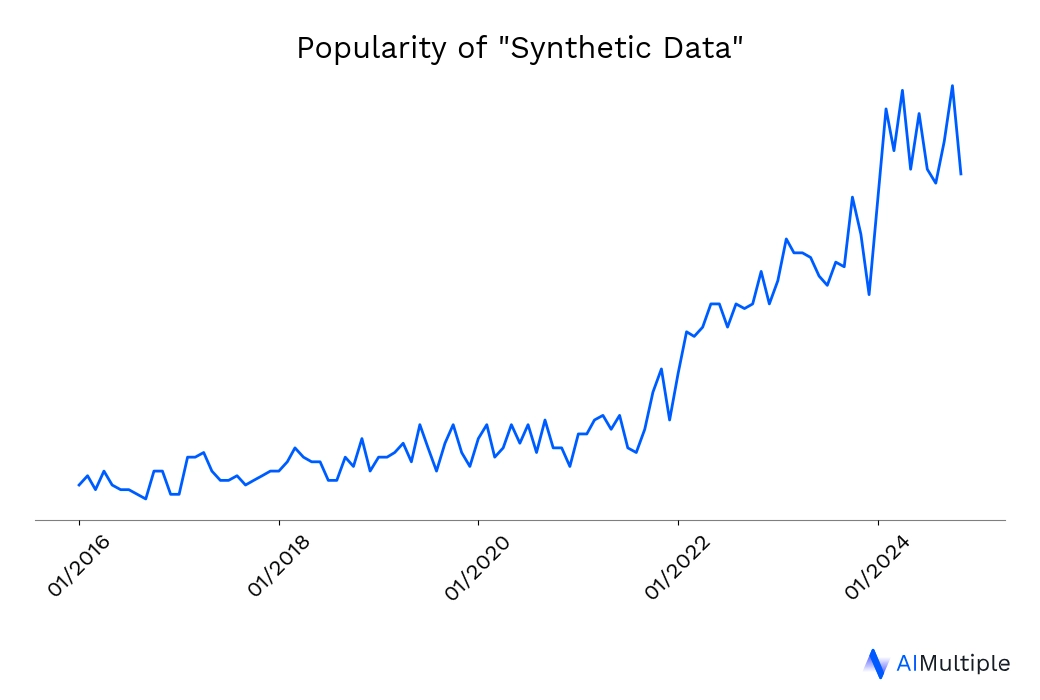
As industries such as healthcare, finance, autonomous driving, and retail continue to adopt synthetic data, it is proving invaluable for training advanced AI models, pushing the boundaries of innovation, and overcoming the limitations of real-world data constraints.
Check AIMuliple’s data-driven list of synthetic data generator vendors.
Comments
Your email address will not be published. All fields are required.