Top 7 Deep Learning Applications in Manufacturing in 2024
Deep learning solutions are transforming manufacturing companies into high-efficiency organizations by these benefits:
- improve productivity
- decrease production defects
- increase capacity utilization
- reduce maintenance costs
What is the level of interest in using deep learning in manufacturing?
Deep learning is a good fit for manufacturing because manufacturing produces significant levels of data (e.g. time-series data from sensors) however most manufacturing companies do not use this data effectively.
According to McKinsey, deep learning models have the potential to create between $1-2 trillion annually in supply-chain management and manufacturing as you can see below.
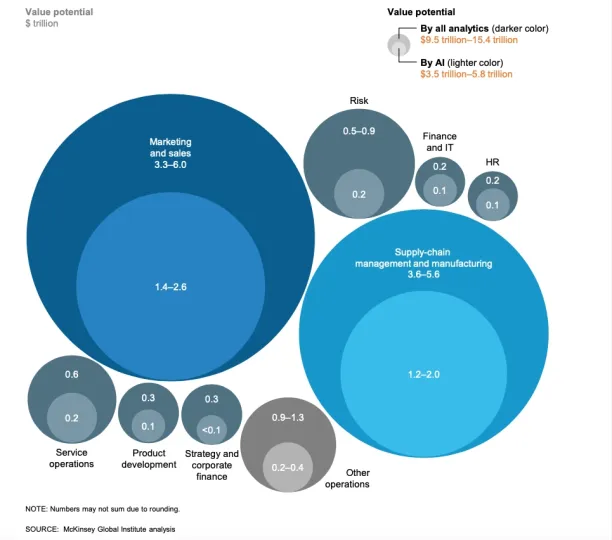
What are deep learning use cases in manufacturing?
Maintenance
Predictive maintenance is increasing in popularity vs preventative maintenance. This could be partially due to more effective predictive maintenance thanks to deep learning solutions.
Predictive analyses based on deep learning are used to prevent failures of a machine by identifying the next potential issues more accurately. Deep learning algorithms analyze real-time sound, image, and other data from sensors installed on equipment to reduce downtime of systems.
Predictive analytics
Deep learning algorithms can accurately predict operational outcomes. It allows companies to optimize their processes.
Deep learning models use real-time sensor data from cameras to create data-driven insights by monitoring production lines, waiting time of machines, inventory, the technical condition of the machines, and unsafe behaviors of workers.
Companies can examine the effectiveness of their processes by analyzing data about raw materials, quality issues, maintenance activities, and other production factors such as temperature and humidity. This ability helps to find non-value added activities, unprofitable lines, and bottlenecks in the operations.
Some of these predictions can be embedded in IoT devices like cameras for real-time decision-making. For more on this, please read how edge analytics is improving operations.
Supply chain management
Deep learning is a novel, data-hungry, and high-accuracy analytics approach. Therefore, it can add value in the complex supply chain management space where simple algorithms are not able to achieve high levels of accuracy. Using deep learning, companies can
- Forecast real-time demand
- Optimize their supply chain operations and production schedules
- Achieve efficient inventory management help to reduce purchasing costs of raw material
These capabilities allow companies to respond quickly to market demand changes like a tanker getting stuck in the Suez channel.
You can also check our supply chain and logistics hub, which includes a variety of supply chain and logistics optimization solutions and tools to find the option that best suits your business needs.
Product development
Machine learning is increasingly used in product design. High-performance product design uses deep learning algorithms for
- product development: Generative design software enables users to input relevant information including KPIs to be optimized and other important aspects of design decisions (e.g. material costs and durability). These software then propose designs and help users make design trade-offs.
- product testing: More realistic testing can be completed by deep learning models without having to build expensive real life prototypes
Engineers in automotive design claim that machine learning will be key to designing faster race cars and neural networks can be used to create more realistic simulations and calculate necessary results to reach optimal performance.
Quality assurance
Reducing production losses/errors is vital for manufacturing firms. Deep learning-powered computer vision capabilities are used to automatically detect defective products on time. Consultants claim that such solutions quality testing can identify 90% of defects in specific use cases.
This enables production teams to fix quality problems earlier. And in general, the earlier the defects are resolved, the cheaper they are to resolve. For example, fixing a car’s wiring is much easier when the wiring is freshly deployed vs after the whole vehicle has been assembled and painted.
As an example, Audi has an image recognition system based on deep learning which was trained using several million test images. The system uses pressed sheet metal images from cameras and analyzes them. It is claimed to be successful in identifying fine cracks on the metal sheets.
Robotics
Companies use industrial robots to handle complicated and dangerous processes. Now, deep learning architectures enable robots to learn on their own. For example, Fanuc, a Japanese industrial robot maker company, is experimenting with deep reinforcement learning models. A robot can train itself for new tasks by object and pattern recognition capabilities of deep learning models.
Logistics
For logistics operations, deep learning models can boost fuel efficiency and delivery time by analyzing real-time data about vehicles and drivers.
If you are ready to use deep learning in your firm, we prepared a data-driven list of companies offering deep learning platforms.
Feel free to read our AI applications in manufacturing research.
If you need help in choosing among deep learning vendors who can help you get started, let us know:
This article was drafted by former AIMultiple industry analyst Ayşegül Takımoğlu.

Cem has been the principal analyst at AIMultiple since 2017. AIMultiple informs hundreds of thousands of businesses (as per similarWeb) including 60% of Fortune 500 every month.
Cem's work has been cited by leading global publications including Business Insider, Forbes, Washington Post, global firms like Deloitte, HPE, NGOs like World Economic Forum and supranational organizations like European Commission. You can see more reputable companies and media that referenced AIMultiple.
Throughout his career, Cem served as a tech consultant, tech buyer and tech entrepreneur. He advised businesses on their enterprise software, automation, cloud, AI / ML and other technology related decisions at McKinsey & Company and Altman Solon for more than a decade. He also published a McKinsey report on digitalization.
He led technology strategy and procurement of a telco while reporting to the CEO. He has also led commercial growth of deep tech company Hypatos that reached a 7 digit annual recurring revenue and a 9 digit valuation from 0 within 2 years. Cem's work in Hypatos was covered by leading technology publications like TechCrunch and Business Insider.
Cem regularly speaks at international technology conferences. He graduated from Bogazici University as a computer engineer and holds an MBA from Columbia Business School.
To stay up-to-date on B2B tech & accelerate your enterprise:
Follow on
Comments
Your email address will not be published. All fields are required.