The data orchestration tools include:
- Open-source tools, which offer flexibility and community-driven development, with top examples:
- Apache Airflow
- Luigi
- Commercial tools, which provide additional support, features, and enterprise-level scalability, with top tools like:
- All orchestration: ActiveBatch and RunMyJobs
- Workflow orchestration: Shipyard
- ETL orchestration: Keboola and Rivery
These tools can enhance data pipelines, improve data quality, and address version control challenges, enabling companies to use 60-75% of their otherwise unused data, as big data stats show.
Discover the leading data orchestration tools to start orchestrating your data pipelines and data warehouses:
Product | Primary use | # of employees | Rating | Workflow Design | Free trial |
---|---|---|---|---|---|
ActiveBatch | WLA & data orchestration | 533 | 4.4 based on 251 reviews | Workflow design with low-code/no-code | ✅ |
RunMyJobs by Redwood | WLA & job scheduling | 533 | 4.8 based on 140 reviews | Centralized console for managing workflows | ✅ |
Stonebranch | WLA & data orchestration | 152 | 4.8 based on 79 reviews | Drag-and-drop workflow designer | ✅ |
Fortra's JAMS | WLA & data orchestration | 9,941 | 4.7 based on 150 | Scripted and parameter-driven orchestration | ✅ |
Azure Data Factory | Data integration & orchestration | 244,900 | 4.4 based on 71 reviews | Visual pipeline design | ✅ |
Google Cloud Dataflow | Stream & batch data processing | 300,040 | 4.3 based on 61 reviews | Unified model for stream and batch data | ✅ |
Keboola | Data orchestration, open-source | 150 | 4.7 based on 90 reviews | Intuitive design for complex workflows | ✅ |
Prefect | Data orchestration & integration | 93 | - based on - reviews | Visual workflow design | ✅ |
Rivery | Data integration & orchestration | 97 | 4.9 based on 89 reviews | Visual-based data pipeline creation | ✅ |
Shipyard | Workflow orchestration & data operations | - based on - reviews | Workflow orchestration via data management | ✅ | |
Kestra | Workflow orchestration & automation | - based on - reviews | Workflow orchestration, automation and scheduling | Open source |
Note: WLA is short for workload automation.
Shortlisted enterprise data orchestration tools
The shortlisted open source and enterprise data tools are plotted below:
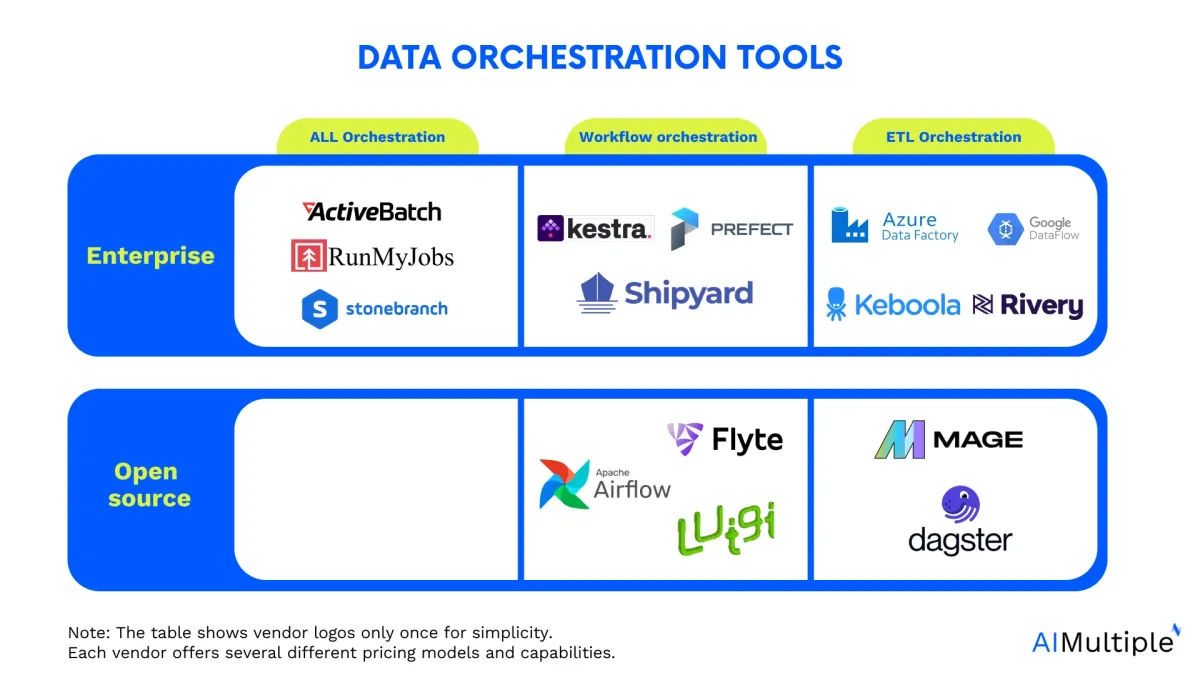
Discover how we shortlisted these tools.
The features below are based on B2B review platforms such as TrustRadius 1 , G2 2 , Peerspot3 , and Capterra 4 .
1. ActiveBatch
ActiveBatch is a workload automation platform designed for data orchestration and ETL pipeline management. ActiveBatch provides:
- Pre-built connectors for Informatica PowerCenter, SAP Crystal Reports, IBM DataStage, Hadoop and more.
- A low-code/no-code interface to design complex workflows that span across cloud, on-premises, and hybrid environments.
- Auto-remediation, customizable alerts and proactive SLA monitoring.
Users can orchestrate data flows, specifically ETL operations, by creating automated workflows and defining dependencies. ActiveBatch allows scheduling tasks, monitoring data pipelines in real-time, and setting up alerts for errors or specific events. The platform supports diverse use cases, from data extraction and transformation to end-to-end business processes.
Pros
- ActiveBatch Workload Automation offers a large number of functions and improved connectivity with other software like ERP and CRM systems.
- The tool is user-friendly, offering drag-and-drop features for workflow creation, pre-defined steps for task automation, and support for various programming languages and cloud platforms.
- Many users appreciate the tool’s integration capabilities, error-handling mechanism, and the option for real-time visibility into status.
Cons
- ActiveBatch’s installation process is complex and requires additional resources.
2. RunMyJobs
RunMyJobs is a workload automation tool that can automate data movement and integration across different environments, supporting a wide range of orchestration scenarios. RunMyJobs manage ETL workflows, by simplifying ETL pipeline orchestration and handling the process of handling large volumes of data efficiently.
RunMyJobs offers:
- SaaS architecture that minimizes the need for installation and upkeep
- Automated load balancing feature that manages cloud operations
- Lightweight and self-updating agents to manage servers and run scripts.
Pros
- RunMyJobs offers a user-friendly GUI interface, 24/7 vendor support and comprehensive troubleshooting guides.
- Users appreciate its cross-platform capabilities, flexibility in workflow creation, and reliable uptime since migration from MS Orchestrator.
- RunMyJobs is praised for its automation of complex workflows, compliance with ITIL and ISO20000, and its ability to run parallel jobs with load balancing.
Cons
- Users report issues with manual daylight saving changes and complex integration with incident management tools.
- Users express a need for better documentation, especially with practical examples.
The visual below shows how RunMyJobs can coordinate and integrate various data flows and system activities, integrating across on-premises environments, operating system tasks, API adapters, and cloud service providers:
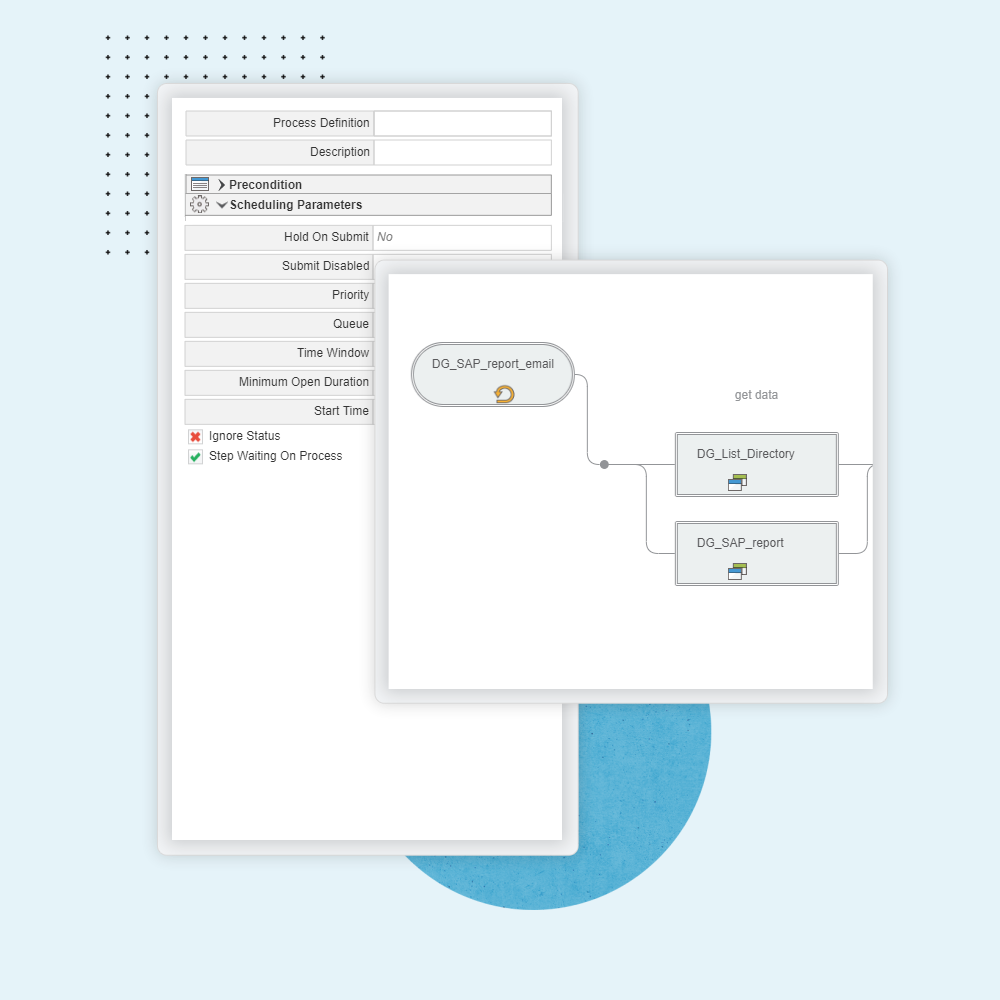
3. Stonebranch
Stonebranch UAC is a centralized SOAP platform that orchestrates data pipelines efficiently, enabling real-time data flow across hybrid IT environments. Stonebranch UAC offers:
- Drag-and-drop workflow designer to simplify workflow creation and management.
- Built-in managed file transfer to secure, encrypted, and fault-tolerant data movement.
- Pre-built integrations to Connect with Hadoop, Snowflake, Kubernetes, and more.
- Lifecycle management to support pipelines-as-code with versioning and Dev/Test/Prod promotion.
Pros
- The tool provides an intuitive graphical interface and enables teams to manage workflows, automate tasks and integrate custom KPIs.
- Stonebranch UAC’s support team assists users in migrating from other platforms and in setting up applications on environments like AWS.
Cons
- Users find the display of multiple-layer nested workflows in a single diagram lacking, making visualization of interconnected processes difficult.
- The product’s authentication methods are limited to basic auth, which some users believe is outdated, and its error messages are considered too generic, leading to a dependency on customer support.
4. Fortra’s JAMS
Fortra’s JAMS is a centralized workload automation and job scheduling solution that orchestrates data pipelines across diverse IT environments. It offers:
- Secure File Transfer Solutions through the GoAnywhere Execution Method, JAMS integrates with GoAnywhere MFT to facilitate secure, encrypted, and reliable data transfers.
- REST API and PowerShell module that leverages APIs to build integrations and connectors to any application or service.
Pros
- Centralized job management: JAMS centralizes job management, enhancing scheduling and automation efficiency for data processing.
Cons
Search functionality: Search capabilities in JAMS are reported as inadequate, requiring users to perform database queries for tasks instead of having a straightforward search function.
5. Azure data factory
Azure Data Factory is a cloud-based data integration service from Microsoft that enables users to create, schedule, and orchestrate complex data pipelines across cloud and on-prem environments, with support for diverse data sources and destinations.
Azure Fata Factory allows users to:
- Design data pipelines
- Set up data transformations
- Orchestrate data movements across Azure cloud platforms.
Azure Data Factory provides a visual interface for creating workflows, along with real-time monitoring, error handling, and extensive integration options.
Pros
- Azure Data Factory allows copying data from various types of sources, running SSIS & SSMS packages, making it an easy-to-use ETL & ELT tool.
- Azure Data Factory is user-friendly with drag & drop functionality for creating pipelines, automating across platforms, and has a wide range of connectors for various servers.
- Users appreciate the UI, frequent feature updates, automation capabilities, and the ability to create complex ETL pipelines without code.
Cons
- Users find it challenging to flatten complex JSON and map nested attributes in Azure Data Factory.
- Some users reported limitations in Azure Data Factory, such as:
- Errors without clear reasons
- Difficulty in integrating with non-Azure services
- Lack of flexibility in moving pipelines between environments.
- Many users mentioned issues with the usability of Azure Data Factory, including:
- A steep learning curve
- Confusing user interface
- Lack of intuitive error notifications
- Outdated documentation.
This image from Azure Data Factory demonstrates its ability to monitor triggered pipeline runs within a specified time frame. Users can adjust the time range and filter by status, pipeline name, or annotation to manage and track pipeline activities:
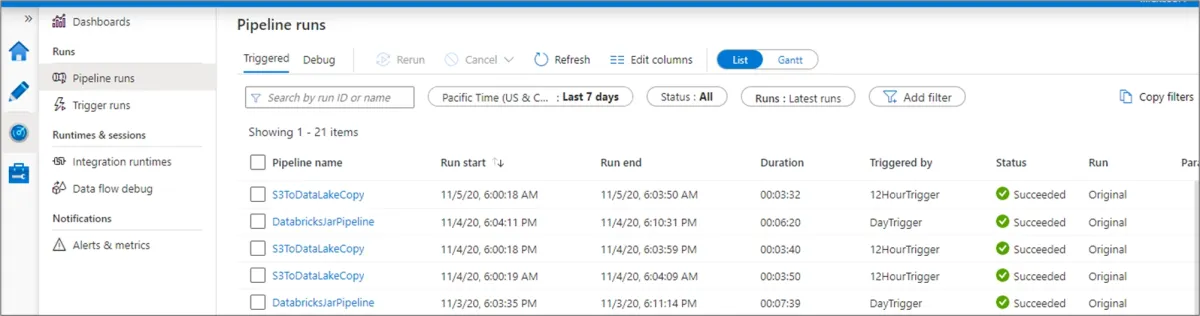
6. Google Cloud Dataflow
Google Cloud Dataflow is a cloud-based data processing service from Google Cloud. It provides a unified model for processing large-scale data in real-time or in batches. Google Dataflow users can:
- Create data pipelines for real-time data processing and integrate with other Google Cloud services like BigQuery.
- Orchestrate complex data workflows, apply transformations, and process data from various sources with automatic resource provisioning and monitoring.
Pros
- Google Dataflow offers easy data loading in both batch and streaming, big data processing, and also data migration.
- Users appreciate its developer-friendly interface due to:
- The ability to create custom apps
- Design APIs based on the Apache Beam framework.
- Its scalability, quick processing of large amounts of data, and the support system are also highlighted positively by users.
Cons
- Users find the platform’s documentation insufficient and the learning curve steep, particularly for beginners.
- Users express dissatisfaction with the limited API for third-party applications.
- Some users complained about the inconsistent features between Java and Python SDKs.
- For some users, slow system performance and connectivity were the major problems.
7. Prefect
Prefect is an open-source data orchestration tool to build, manage, and monitor complex workflows. It provides a flexible and extensible framework for defining and scheduling workflows with features like task retries, error handling, and comprehensive monitoring.
- Create and manage workflows using API and UI.
- Orchestrate tasks, schedule job execution, and handle errors.
- Monitor and alert system to maintain data pipelines.
Pros
- Prefect is appreciated for its straightforward setup, Python-native design, and clean code approach.
- Users highlight Prefect’s usability across various platforms, and the supportive community.
- The product offers easy automation of data pipelines, management of multiple versions of a pipeline.
Cons
- Prefect lacks comprehensive integration with data governance tools and versatile language support.
- Users find Prefect’s documentation inconsistent and its frequent API changes challenging to keep up with.
- Some users reported difficulty with site layout changes, handling queues, and limitations with concurrency and parallelism.
The visual below displays Prefect’s capabilities:
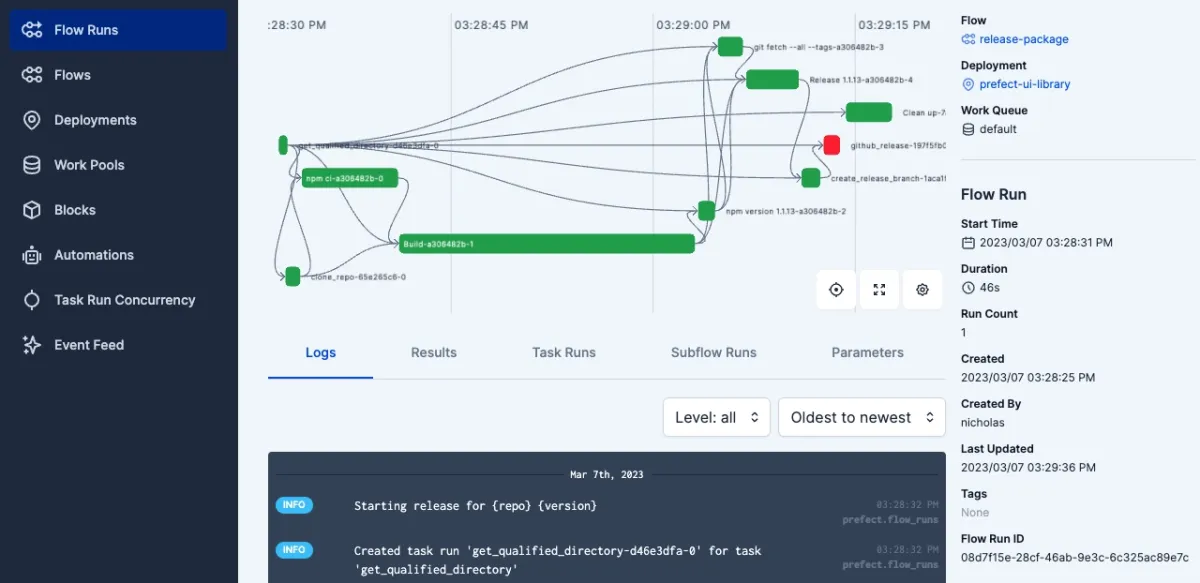
8. Rivery
Rivery is a cloud-based data orchestration platform designed for building and managing data pipelines. It focuses on data integration and ETL, providing a visual interface to create, schedule, and automate complex data workflows.
Rivery users can:
- Build data pipelines by dragging and dropping tasks into a visual workflow
- Schedule, monitor and set alerts to manage the orchestration process
- Integrate with data sources and destinations to automate data extraction, transformation, and loading tasks across different platforms.
Pros
- Rivery users appreciate its automation of common ETL challenges, such as managing target schema and incremental extraction from systems like Salesforce or NetSuite.
- The product’s responsive and professional support is praised, along with its integration and data pipelines management capabilities.
- Users find Rivery’s user interface intuitive and its learning curve flat, allowing the creation of scalable ETL systems in a few hours with just SQL knowledge.
Cons
- Users found difficulties in managing multiple environments and variables due to Rivery’s UI, and experienced minor bugs.
- The product lacks certain integrations and a functionality for tracking API releases.
- The documentation could be improved.
- Some users expressed difficulty in managing dependencies between processes.
- Some users complains about the error messages as they are not user-friendly.
The video below shows how Rivery can serve as a DataOps management tool:
9. Keboola
Keboola is a data platform that integrates, transforms, and orchestrates data. It simplifies the creation of complex data workflows and automates processing tasks, aiming to streamline data operations for business users.
Users can:
- Create, schedule, and manage data pipelines with visual interface
- Orchestrate data workflows and automate ETL processes through flexible scheduling, error handling, and real-time monitoring.
Pros
- Keboola provides a range of connectors and allows for flexible ETL pipeline architecture.
- Keboola’s setup is easy and infrastructure-independent with multiple language support for transformations.
- Users appreciate Keboola’s support team and its data security standards.
Cons
- Users find Keboola’s error messages unclear and its extractors limited in customization, leading to excessive data downloads.
- Users find the sandbox interface complicated.
- Users criticise the speed of data pipeline processing as it needs improvement for handling incremental data requirements.
The image below shows an overview of Keboola platform:
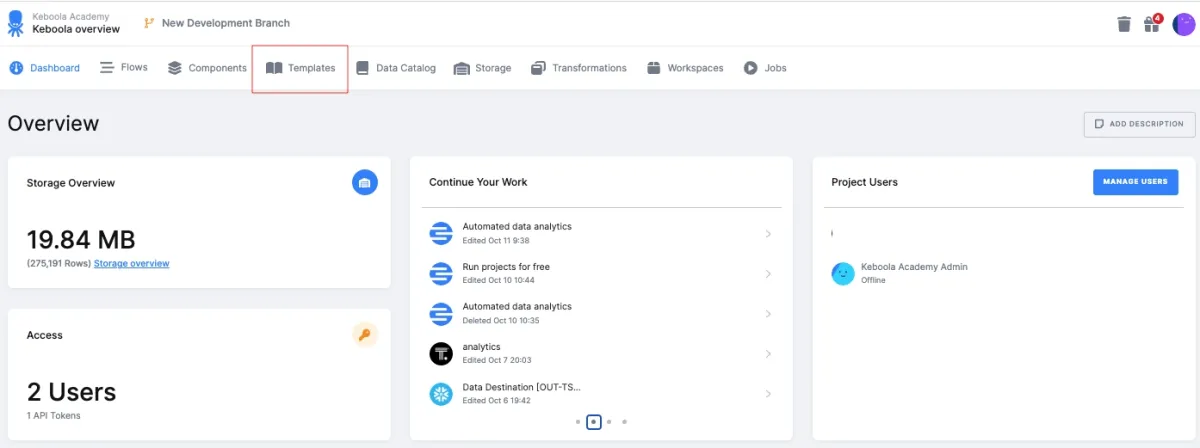
10. Shipyard
Shipyard is a data orchestration tool that connects various data tools and processes to enhance data operations, offering workflow orchestration and infrastructure development from scratch. It provides:
- Low-code templates for visually designing data workflows, accelerating production deployment without coding requirements.
- Internal or external procedures using scripts in their preferred language.
- Observability and alerting features to detect breakages proactively, ensuring data reliability before impacting downstream business teams.
Open-source data orchestration tools
Here is a list of top open-source data orchestration tools with GitHub stars:
Tool | Primary Use | GitHub Star |
---|---|---|
Apache Airflow | Workflow orchestration | 34.5k |
Airbyte | ETL / ELT data pipeline orchestration | 17k |
Prefect | Workflow orchestration | 18.1k |
Dagster | Data orchestration | 10.2k |
Mage | Data pipeline orchestration | 3.9k |
Luigi | Data pipeline orchestration | 17.3k |
Apache NiFi | GenAI data pipeline processing | 5.1k |
Flyte | Data and ML workflow orchestration | 4.8k |
Apache Airflow
Apache Airflow is an open-source platform for authoring, scheduling, and monitoring workflows as Directed Acyclic Graphs (DAGs). Its Python-based design offers flexibility, while the web interface simplifies visualization and management. Airflow integrates with tools like Hadoop, Spark, and Kubernetes, providing scalability for large-scale workflows.
Key features:
- Web UI for monitoring and debugging.
- Python-based workflow creation with task dependency management.
- Directed Acyclic Graphs (DAGs) for pipeline structure.
- Scalable, distributed architecture for large workloads.
- Plugins and operator libraries.
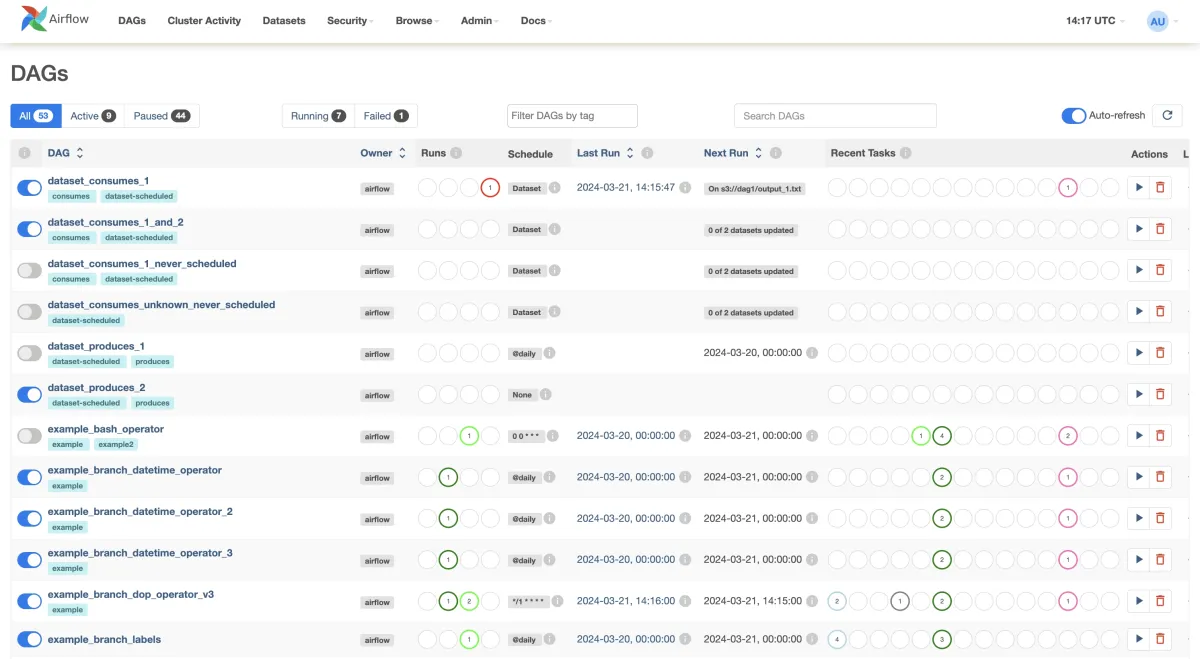
Dagster
Dagster is an open-source platform for managing data pipelines, focusing on data assets, observability, and integration. It introduces Software-Defined Assets (SDAs) for reusable workflows and pipeline control. Its web interface (Dagit) allows users to visualize, debug, and monitor pipelines, making it suitable for ETL, analytics, and machine learning. Dagster supports both local and distributed execution, offering deployment flexibility.
Key features:
- Integration with frameworks like dbt, SQL, and Pandas.
- Data-aware orchestration with asset management and versioning.
- Support for pipeline testing to ensure data quality.
- Modular architecture for local or distributed execution.
- Visual tools for debugging and monitoring.
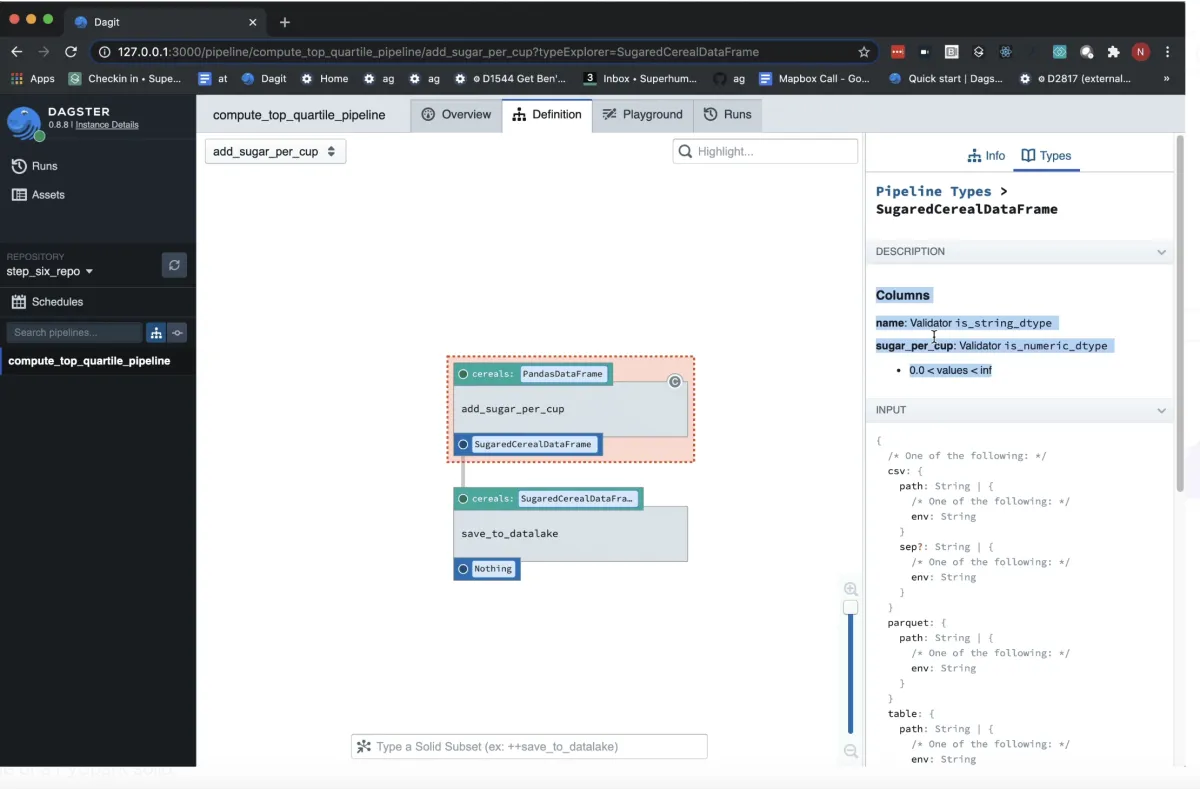
Mage
Mage is an open-source data integration tool focused on creating and managing real-time and batch data pipelines with minimal complexity. Its low-code interface and multi-language support (Python, SQL, and R) make it accessible for diverse teams. Mage stands out with an interactive notebook UI, offering instant feedback and seamless testing for streamlined development.
Key features:
- Monitoring and alerts to proactively address pipeline issues.
- Multi-language support to build pipelines using Python, SQL, or R.
- Interactive notebooks to test and debug code in real-time.
- Cloud integration to deploy pipelines with Terraform on platforms like AWS or GCP.
- Data as assets to version, partition, and catalog pipeline outputs.
Luigi
Luigi is an open-source Python framework designed for building and managing complex data workflows. Originally developed by Spotify, it excels in orchestrating tasks with intricate dependencies, ensuring efficient execution of batch processes. Luigi’s lightweight and extensible design makes it a go-to tool for small to medium-scale pipelines.
Key features:
- Workflow management with error handling and monitoring.
- Dependency resolution to automatically manage task execution order.
- Python API to simplify task definition with minimal coding.
- Batch processing for ETL jobs and large data workflows.
- Integration with Hadoop, Spark, and other big data tools.
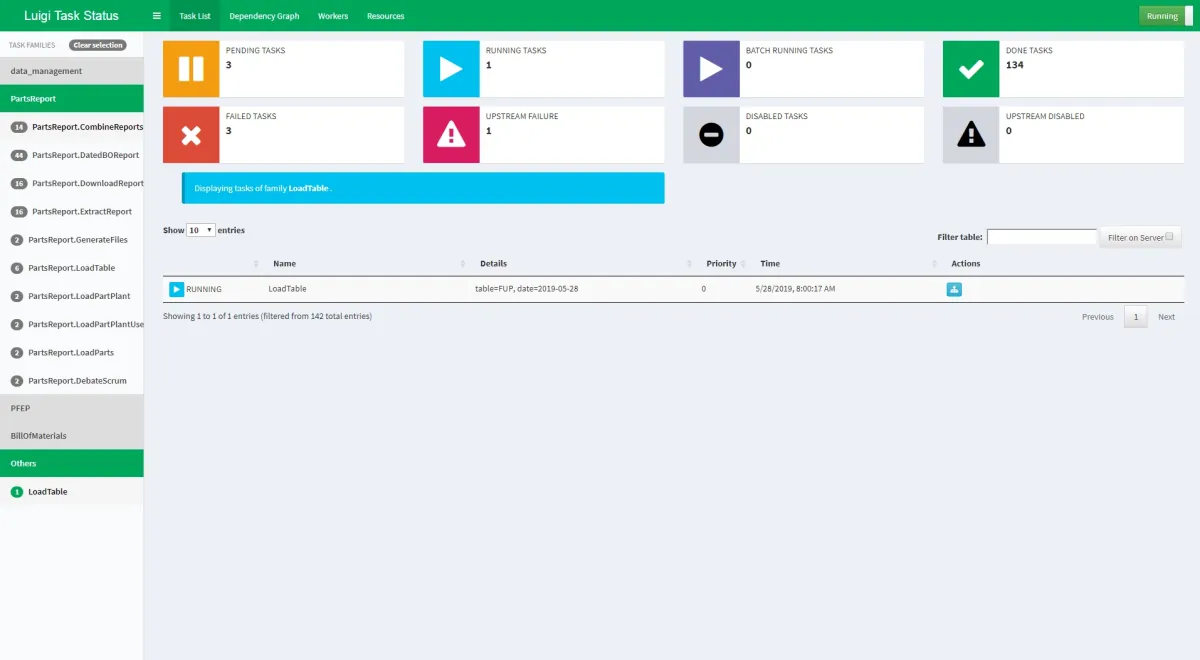
Flyte
Flyte is an open-source, Kubernetes-native platform for orchestrating complex workflows in data processing and machine learning (ML). Designed for scalability, reproducibility, and collaboration, it simplifies the development and management of production-ready pipelines.
Key features:
- Kubernetes-native design
- Integrations with diverse data and ML tools for flexibility.
- Multitenancy to enable decentralized development on a shared infrastructure.
- Dynamic execution to support fault-tolerant and high-availability pipelines.
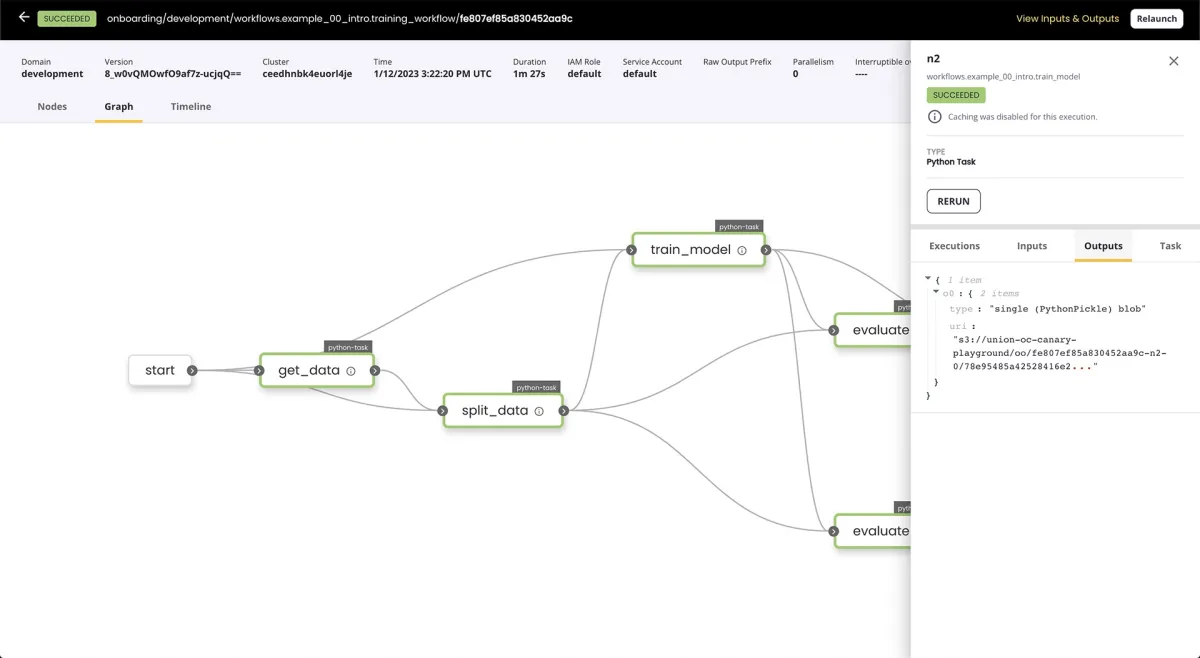
Screening data orchestration tools
We shortlisted companies for this benchmark based on two key criteria:
- The number of employees: 30+ employees on their LinkedIn profile.
- Presence in B2B review sites: 10+ reviews on all platforms for enterprise tools.
FAQs
What is data orchestration?
Data orchestration is the process of coordinating, integrating, and automating data workflows across different sources and systems to ensure seamless data movement and consistency. It involves managing data pipelines, transformations, and dependencies to deliver accurate and timely data for business insights.
A data orchestration tool is a category under orchestration tools to streamline management tasks by providing features like workflow design, scheduling, monitoring, and error handling. These tools help maintain data quality, reduce manual intervention, and support collaboration among data engineers, analysts, and data scientists.
Learn other relevant concepts to data orchestration such as:
- DataOps to learn data operations practices and tools
- DataOps vs MLOps to learn differences between the two concepts
- Data governance to learn how to comply with privacy and security rules and regulations
- Machine learning and data governance to learn its applications with ML
4 steps to orchestrate your data
Data collection
When a customer engages with an organization’s service or product, each touchpoint can generate new data. The data generated can be stored in silos or siloed over time. Siloed data is not fully accessible to other departments and creates information barriers between departments.
Data orchestration tools automatically collect real-time data from various sources, centralizing access and supporting data governance. They connect data systems across the organization, ensuring incoming data complies with governance rules, blocking non-compliant sources.
Data preparation and transformation
Data orchestration tools collect data from different types of sources, and these sources may contain different types of data. In this case, not all collected data can be used in the same system, so they need to be handled differently. Data from diverse systems is transformed into a compatible and consistent format by an orchestration tool to ensure that it works within a specific task. If the properties of the collected data are not standardized, orchestration tools check the properties of the incoming data and standardize its properties and values.
For example, customer names are one of the values of data, and all names should be checked and transformed based on an internal standard data schema. If there are outliers, they are removed by the orchestration tools.
Data unification
After converting the collected data into a compatible and consistent format, the orchestration system creates a single and unified view of all customer profile data. It ingests customer data in real-time and keeps the data updated to show the current state of the customer profile.
It brings together all the data collected from all the company’s sources, such as websites, applications, and other touchpoints.
Activation
Once unified profile data is created, data orchestration makes this information available to the tools used by the company’s teams on a daily basis. The transformed data is sent to data storage systems such as data warehouses, databases, or data lakes. From here, the orchestration tools make the data available to all teams and their internal systems. There is no need to load data into your system.
What is modern data stack?
The “Modern Data Stack” (MDS) is a cloud-based data management and analysis approach which incorporates key elements of data infrastructure, such as:
- Data infrastructure refers to the architecture that supports data operations. It includes cloud-based platforms and scalable storage solutions like Snowflake, BigQuery, and Amazon S3, which help centralize data and allow for easy scalability.
- Data catalog tools play a crucial role in organizing and documenting datasets, providing a centralized resource for metadata and ensuring easy data discovery. This is key to preventing data silos and promoting collaboration across teams.
- Data governance ensures that data is managed according to regulations and best practices. It involves setting policies, standards, and procedures for data use, ensuring compliance, and maintaining data quality. Tools for data observability, like Monte Carlo or Great Expectations, can aid in monitoring data quality and lineage.
- Data engineering encompasses the processes and techniques used to prepare data for analysis. This includes data integration, transformation, and orchestration, with tools like Fivetran, dbt, and Apache Airflow. Effective data engineering ensures that data is consistent and ready for use in business intelligence and analytics.
Some of the tools that are utilized in MDS include:
- Data orchestration tools connects various components of the MDS, ensuring that data flows seamlessly, is transformed correctly, and is available for analysis in a reliable and automated manner.
- Data integration tools that extract, load, and transform data from various sources into a central repository.
- Data warehousing tools which are centralized storage solutions to support large-scale data analysis.
- Business intelligence (BI) and analytics tools that enable data exploration, visualization, and reporting.
- Data Observability tools that can monitor and ensure data quality, lineage, and accuracy.
7 benefits of data orchestration
Data orchestration transforms how businesses manage, process, and utilize their data by automating and streamlining data workflows. This enables companies to extract actionable insights quickly and efficiently. Here are the primary benefits:
1. Increased Efficiency
- Automates repetitive data tasks, reducing manual intervention and minimizing errors.
- Frees up resources, allowing teams to focus on strategic initiatives rather than operational bottlenecks.
2. Improved Scalability
- Handles large and complex datasets with ease, enabling organizations to grow without compromising performance.
- Adapts to increased data volume and new data sources as business needs evolve.
3. Enhanced Data Quality
- Standardizes, cleans, and validates data from diverse sources, ensuring consistency and accuracy.
- Provides a unified view of data, eliminating silos and enabling informed decision-making.
4. Better Security and Governance
- Centralizes data management to enforce strict security protocols and ensure compliance.
- Facilitates access control, allowing only authorized users to retrieve sensitive data.
5. Faster Time to Insights
- Streamlines the flow of data from collection to analysis, accelerating access to actionable insights.
- Enables businesses to respond swiftly to market dynamics and seize opportunities.
6. Improved Collaboration
- Democratizes data access, enabling teams across departments to work seamlessly on shared datasets.
- Enhances communication and coordination by automating data sharing and reducing dependencies on IT teams.
7. Simplified Cloud Migrations
- Facilitates the transition of on-premises data to cloud environments with minimal disruption.
- Supports incremental migrations, ensuring data integrity and reducing complexity.
Data orchestration vs ETL orchestration tools
Similarities
- Data Processing: Both ETL orchestration and data orchestration involve processing data to make it ready for analysis or other business uses.
- Automation: Both concepts emphasize automating workflows to streamline data management processes and reduce manual intervention.
- Data Integration: They both focus on integrating data from different sources to create a unified view.
Differences
- Scope: ETL is a specific process involving extracting data from sources, transforming it into a desired format, and loading it into a target system. Data orchestration has a broader scope, covering the coordination and automation of data workflows, which may include ETL processes but can also manage more complex data pipelines.
- Purpose: ETL is designed primarily for data movement and transformation, while data orchestration focuses on orchestrating and managing multiple processes or workflows, which may involve ETL and other tasks like data validation, cleaning, or merging.
- Complexity: Data orchestration can manage complex dependencies and workflows involving multiple data pipelines, while ETL typically handles individual data flows.
- Tools: ETL orchestration tools are designed specifically for ETL tasks. Data orchestration tools provide a framework for orchestrating complex workflows, which can include ETL tasks alongside others.
Aspect | ETL | Data Orchestration | Similarities |
---|---|---|---|
Scope | Focuses on extracting data from sources, transforming it, and loading it into a target system. | Coordinates and automates multiple data processes, often including ETL but also other tasks. | Both involve data workflows and automation. |
Purpose | Designed to move and transform data. | Ensures seamless coordination of complex workflows, involving multiple processes. | Aimed at providing consistent and reliable data flows. |
Complexity | Typically handles individual data flows and processes. | Manages complex dependencies and workflows involving multiple data pipelines. | Focus on reducing manual intervention through automation. |
Tools | Uses specialized ETL tools like Talend, Informatica, etc. | Utilizes orchestration tools like Apache Airflow, Prefect, Dagster, etc. | Both tool types support automation and scheduling. |
Flexibility | ETL tools are tailored for specific tasks, with less focus on broader coordination. | Data orchestration tools offer more flexibility in managing complex workflows and dependencies. | Both aim to improve efficiency and scalability in data management. |
Error Handling | Basic error handling within the scope of data extraction, transformation, and loading. | Provides robust error handling, monitoring, and recovery for complex workflows. | Both aim to ensure consistent and accurate data processes. |
Further reading
Explore more on orchestration and automation software that can help manage and orchestrate data:
External sources
- 1. TrustRadius: Software Reviews, Software Comparisons and More. TrustRadius
- 2. ActiveBatch Workload Automation Reviews 2025: Details, Pricing, & Features | G2.
- 3. Peerspot. Revisited July 11, 2024.
- 4. Capterra: Find The Right Software and Services. Capterra
- 5. Enterprise ETL Automation Solution.
- 6. Visually monitor Azure Data Factory - Azure Data Factory | Microsoft Learn.
- 7. Introduction - Prefect.
- 8. Accelerate Your End-to-End Data Pipelines With Keboola Data Templates.
- 9. GitHub - apache/airflow: Apache Airflow - A platform to programmatically author, schedule, and monitor workflows.
- 10. Dagster: The Data Orchestrator | Dagster Blog.
- 11. GitHub - spotify/luigi: Luigi is a Python module that helps you build complex pipelines of batch jobs. It handles dependency resolution, workflow management, visualization etc. It also comes with Hadoop support built in..
- 12. Mitigate the trade-off between scalability and ease of use with Flyte.
Comments
Your email address will not be published. All fields are required.