AI-generated images are becoming increasingly common from social media to news outlets and creative industries. One recent example is the viral trend of AI-generated “Ghibli-style” images, which sparked debate over artistic ethics, generative AI copyright issues via the unauthorized use of Studio Ghibli’s distinctive aesthetic.1
As these synthetic visuals grow more realistic and accessible, the ability to detect them has become a critical concern for upholding generative AI ethics, combating misinformation, and ensuring image authenticity.
We compared the top 4 AI image detectors and seen that most detectors are no better than a coin toss. See insights into their accuracy, limitations, and readiness for real-world applications:
AI image detector benchmark results
For more: Detailed methodology for image detector benchmark
Detailed evaluation of AI image detectors
Wasit AI
Wasit AI provides tools for analyzing low-level pixel inconsistencies and statistical patterns. It is designed for use cases where image authenticity is critical, such as legal, journalistic, or academic contexts.
The results from Wasit AI are indicated on a color-coded scale ranging from red (likely AI-generated) to green (likely human-made). If the pointer lands in the green zone, it suggests high confidence that the image is authentic.
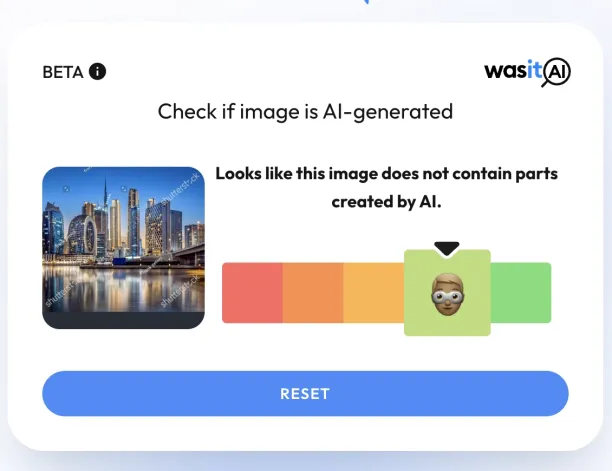
Figure 1: The figure shows that the uploaded photo was detected as not containing any AI-generated elements, indicating it is likely a real photograph.
Brandwell
Brandwell focuses on detecting the misuse of brand elements in AI-generated images, such as counterfeit logos or unauthorized adaptations of brand identity. It also includes AI-generated text detection, useful for identifying synthetic content in both visual and written formats.
Decopy AI
Decopy AI is designed to identify AI-generated copies of existing copyrighted images. It includes a reverse image search function to trace images’ origins and check for potential replication or misuse.
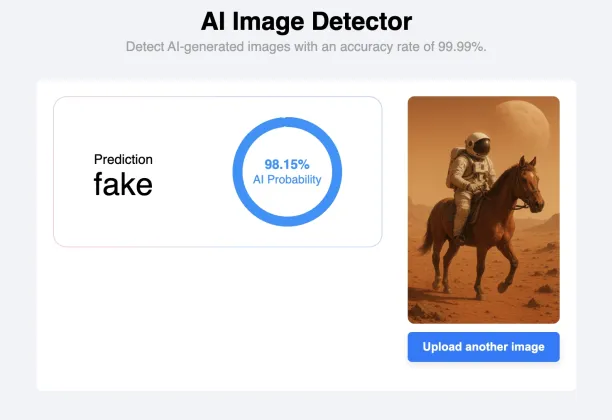
Figure 2: Decopy AI accurately identified the image as AI-generated, assigning it a high AI probability of almost 99%.
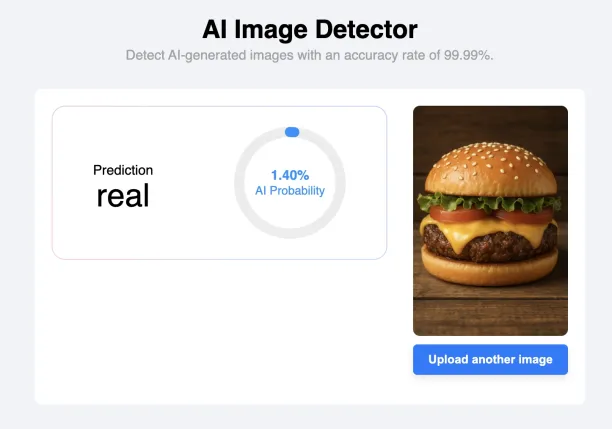
Figure 3: Decopy AI misclassified this AI-generated image of a cheeseburger as real, with an AI probability of only 1.40%. Despite the image’s hyper-realistic style, the result illustrates a false negative where synthetic content was undetected.
Illuminarty
Illuminarty detects AI-generated image manipulations and deepfakes, emphasizing spotting subtle alterations in visual media. It also supports AI text detection and offers a browser extension to analyze content directly during web browsing.
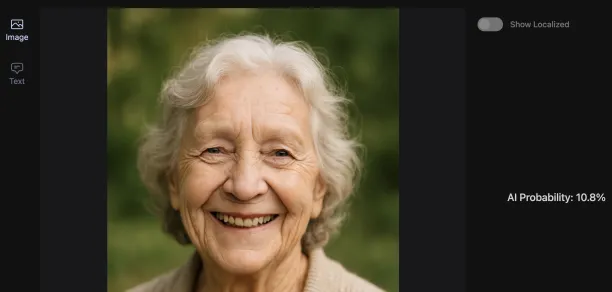
Figure 4: An AI-generated image of an elderly woman that was incorrectly classified as likely real, with an AI probability score of only 10.8%. It illustrates a false negative, where the system failed to detect the synthetic nature of the image.
Detector evaluation criteria
We evaluated AI image detectors based on the criteria below:
1. Ease of use (2 points)
- How intuitive is the interface?
- Can a non-expert easily upload and analyze images?
- Are instructions and feedback clear?
2. Detection accuracy (Practical test) (10 points)
- How often does it correctly identify:
- Are real images real?
- Are AI-generated images fake?
3. Feature set (4 points)
- Batch upload (multiple images at once)?
- File format support (JPG, PNG, WebP, etc.)?
- Does it give any confidence score or explanation?
- Can it highlight why it thinks something is AI-generated?
4. Speed (2 points)
- How fast is the result after uploading?
- Does it lag with multiple images?
5. Output clarity (2 points)
- Are the results clear and understandable? e.g., “AI-generated (85% confidence)” vs. vague statements.
- Any visual aids (heatmaps, labels)?
Detector evaluation methodology
- Choose 5 images from ShutterStock with these keywords: portrait of an elderly woman smiling, Golden retriever in a park, futuristic city skyline at night, close-up of a cheeseburger on a wooden table, and astronaut riding a horse on Mars.
- Create 5 images with the keywords above using ChatGPT image generation.
- Check both ShutterStock and AI-generated images using the tools.
Prompts & why we choose them
“Portrait of an elderly woman smiling”
To test human facial features, skin texture, and age-related details. Useful for checking how detectors handle photorealism vs uncanny valley in humans.
“Golden retriever in a park”
A common dog breed; allows checking fur texture, background blending, and anatomical correctness.
“Futuristic city skyline at night”
Non-living, complex structures and lighting effects can be a good test for architectural coherence and lighting realism.
“Close-up of a cheeseburger on a wooden table”
A common food imagery can be useful for testing texture realism (melted cheese, grill marks, etc.) and depth of field.
“Astronaut riding a horse on Mars”
A surreal, imaginative prompt can be good for testing how detectors handle fantasy or absurd but visually realistic compositions.
Limitations of AI image detectors
Based on our evaluation of four AI image detection tools, we identified several key limitations that raise concerns about their effectiveness. Most notably, all tested tools tended to misclassify AI-generated images as real, which is particularly problematic when accurate detection is essential. While they performed slightly better at recognizing real images, their overall consistency remains uncertain.
Another recurring issue is the lack of transparency around confidence scores. Although some tools indicate their confidence in their classifications, none provide insight into the rationale behind their decisions. This lack of clarity makes it difficult to interpret the results and undermines user trust.
While our findings are based on a limited sample, they suggest that the current tools may not yet be reliable or mature enough for use in applications that require high accuracy, accountability, and interpretability.
Here are some of the possible causes behind these issues:
Evasion by advanced AI generators
Modern AI image generators are constantly improving. As these AI platforms evolve, they can create increasingly difficult images for detectors to flag.
Techniques like image post-processing, resizing, format conversion (e.g., converting to .png or compressing), or adding noise can help AI-generated content evade detection.
The race between detectors and generators
There’s an ongoing cat-and-mouse game between AI detectors and AI generators. As image generators become more sophisticated, AI image detection models must be constantly updated.
Lags in updates can reduce the ability to detect AI-generated images accurately, especially when popular image generators release new versions.
Confidence score isn’t always conclusive
AI detectors usually provide a confidence score indicating how likely an image is AI-generated. However, this score can sometimes be misleading or overly cautious.
Users may interpret low or medium scores as inconclusive, which would make it hard to make informed decisions without additional human reviewers or context.
Over-reliance on models and training data
The detector’s model is only as good as the database it was trained on. If the training data lacks diversity or doesn’t include images from newer AI generators, it may fail to detect or identify images accurately.
There’s also the risk of bias in detection when certain styles or content types are more easily flagged than others. Human-in-the-loop practices can help mitigate this over-reliance issue.
False positives and negatives
AI detectors can:
- Flag real images as AI-generated (false positives), which can undermine trust in authentic content.
- Miss AI-generated images cleverly altered (false negatives), which may allow fake photo evidence or deepfake images undetected.
Data privacy concerns
Due to data privacy issues, some users may hesitate to upload images to online detection services. Storing or analyzing images on third-party servers can be risky if privacy policies are unclear or user data is reused.
Lack of explainability
Most detectors don’t offer insight into why an image was flagged. Without transparent reasoning or visual cues, users must trust the detection output without fully understanding its image analysis. Check out explainable AI to learn more.
Image detection: Why is it important?
AI image detection is critically important in today’s digital landscape, where AI-generated content is becoming increasingly common and harder to distinguish from real media.
With the rise of large vision models and AI image generators like DALL-E and Stable Diffusion, users can easily create hyperrealistic images that blur the line between authentic visuals and synthetic media.
An AI image detector helps detect AI-generated images by using advanced image analysis techniques. These detectors analyze metadata, pixel patterns, and other digital signatures often left behind by AI generation models.
The goal is to identify AI-generated images with higher accuracy, providing clear results and a confidence score to help users make informed decisions.
AI-generated and manipulated images are increasingly used in fake news, fraudulent accident reports, fake IDs, and even fake photo evidence, all of which can harm users and erode public trust.
By using image detectors, individuals, platforms, and organizations can determine whether an image was created by humans or through AI platforms. This protects against plagiarism, safeguards data privacy, and prevents the spread of misinformation. To learn more, check out AI ethics and responsible AI.
AI image detection tools are helpful in detecting deepfake images and are essential for plagiarism detection, content moderation, and image authenticity.
Some platforms offer browser extensions or online tools where users can upload an image or paste an image URL, and the detector will provide a detailed analysis of whether the image is AI-generated.
Comments
Your email address will not be published. All fields are required.