We benchmarked 4 no-code machine learning platforms across key metrics: data processing (handling missing values, outliers), model setup and ease of use, accuracy metrics output, availability of visualizations, and any major limitations or notes observed during testing.
Tool | Best For | |
---|---|---|
1. | ![]() ChatGPT Data Analyst |
Guided analysis and model evaluation for quick data exploration |
2. | ![]() Gemini |
Complex ML tasks with natural language, no coding needed |
3. | ![]() Akkio |
Fast predictive modeling with basic prep, great for analysts |
4. | ![]() Gigasheet |
Simple filtering and exploration for non-technical spreadsheet users |
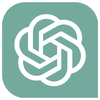
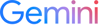
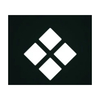
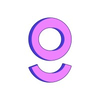
No Code Machine Learning Tools Comparison
Gemini | ChatGPT Data Analyst | Akkio | Gigasheet | |
---|---|---|---|---|
Data Processing | Fills missing data, handles outliers, encodes categories, standardizes numbers | Auto preprocessing and train-test split; missing/outlier steps unclear | Cleans data, handles missing/outliers, merges rare categories, gives summary | No summary or smart cleaning; only manual filters |
Ease of Use | Upload data and describe task in plain language | Upload data and ask in plain language | Very easy UI, just select target and features | Excel-like interface, only simple queries work |
Accuracy Metrics | Full report: accuracy, precision, recall, F1, confusion matrix | Accuracy, precision, recall, F1, confusion matrix | Accuracy, precision, recall, F1 | No metrics, model doesn’t work |
Visualizations | Rich charts like heatmaps and distributions | Text summaries and tables only | Auto-generated basic plots | Manual plots, column by column |
Limitations | Low accuracy in first run, sensitive to class imbalance, no personal queries | Limited charts, average performance, needs prompting for deeper insights | Limited selection of algorithm or split; complex prompts may fail | 100-row limit in free version, model feature fails, weak with complex tasks |
No Code Machine Learning Tools
1- Akkio AI Analytics for Media Agencies
Akkio is a no-code machine learning platform that allows users to build and deploy predictive models quickly, with automated data cleaning and a simple interface. While it offers clear evaluation metrics like accuracy and F1 score, it lacks customization options and advanced control over model training.
Pros
- User-friendly interface. No coding skills required, ideal for non-data scientist users.
- Smart, automated cleaning. Handles missing values, outliers, and redundant data efficiently.
- Built-in chatbot. Guides users interactively through data exploration and modeling.
Cons
- Limited customization. No control over algorithm selection or training process.
- No advanced modeling options. Missing tuning tools, reflecting limited machine learning expertise focus
- Limited model transparency. Users can’t view or modify how the model is built or trained.
2- Gigasheet: Self-Service Analytics, Spreadsheet Easy
Gigasheet functions more like a browser-based spreadsheet than a complete AI-driven data analysis platform. It provides basic filtering and manual charting but lacks automated machine-learning features or support for complex predictive tasks.
Pros
- Web-based tool with a familiar spreadsheet interface—easy for Excel users.
- Suitable for simple data analysis tasks without writing code.
- No-code platform accessible to business analysts or non-technical users.
Cons
- Lacks machine learning models and predictive analytics capabilities.
- Limited data analysis features—only basic filtering and charting.
- There is no support for natural language processing or AI applications.
3- Gemini
Gemini is a no-code AI tool that supports natural language queries to automate feature engineering, model training, and evaluation. Despite offering a full ML pipeline and rich visualizations, its predictive performance is limited by data imbalance and model constraints.
Pros
- Enables users to build custom machine learning models without code.
- Supports natural language processing for fully conversational workflows.
- Handles data analysis, feature engineering, and model evaluation end-to-end.
Cons
- Limited performance if input data quality is low or features are weak.
- No control over hyperparameters suits those with limited machine-learning expertise.
- Best results require balanced datasets for successful model performance.
4- ChatGPT Data Analyst
ChatGPT Data Analyst lets users build machine learning models through plain-language instructions, automating everything from preprocessing to model evaluation. It performs well in basic classification tasks and offers privacy-aware, conversational data analysis.
Pros
- Predictive models are built from natural language; no machine learning programming is needed.
- Strong at exploratory data analysis and visual summaries.
- AI and machine learning tools are fully integrated in a no-code environment.
Cons
- Some models perform modestly—and may need better feature selection or class balancing.
- Doesn’t allow complete customization of ML model training.
- Still dependent on clear prompts to deliver accurate analysis results.
Statistical Comparison of No-Code Machine Learning Tools
Note: Scores represent average performance across kNN and Logistic Regression where applicable. Results may vary based on dataset complexity.
Our benchmark tested three no-code machine learning platforms: ChatGPT Data Analyst, Akkio, and Gemini. All tools were evaluated using the same dataset and two basic classification models, k-Nearest Neighbors and Logistic Regression.
ChatGPT Data Analyst achieved the best overall performance, showing the highest average accuracy and F1 score. These results may reflect more effective data preprocessing or better-balanced input data.
Akkio provided clear accuracy scores and class-wise metrics, making it suitable for basic predictive tasks and understanding feature impact, although its performance remained close to the baseline.
Gemini offered a complete end-to-end pipeline with full preprocessing and detailed metric reporting but produced very low accuracy, likely due to class imbalance or dataset complexity.
These findings highlight that model performance depends heavily on data quality, appropriate model choice, and balanced inputs, even in no-code ML platforms. While such tools simplify machine learning workflows, thoughtful data preparation and evaluation are key to building reliable predictive models.
Methodology
To evaluate the usability and capabilities of no-code machine learning platforms, we selected four widely accessible tools: Akkio, Gigasheet, Gemini, and ChatGPT Data Analyst. Each platform was tested using the same dataset and guided through a consistent set of tasks, including data cleaning, exploratory analysis, model training (using kNN and Logistic Regression), and performance evaluation based on accuracy, precision, recall, F1 score, and confusion matrix outputs.
We focused on three key criteria:
- Ease of use: How intuitive and accessible (drag and drop interface, data preparation) the interface is for non-technical people.
- Analytical depth: The platform’s ability to process data, build models, and deliver useful metrics.
- Flexibility and guidance: Users can interact naturally, explore alternatives, and receive meaningful feedback.
All tests were conducted under free or standard access levels to reflect real-world user experiences.
Benefits of No-Code ML Platforms
No-code machine learning tools make it easy to build and deploy automated machine learning models with no code solutions, bringing data science capabilities to non-technical users. They handle feature engineering, model training, and deployment automatically. Business users can create code machine learning tools without needing technical skills. Many platforms also offer ready-to-use models for tasks like language processing, image recognition, and deep learning.
Overview of Machine Learning Models
No-code tools help business users and analysts create ML models and manage model deployment without writing a single line of code. These platforms support basic machine learning techniques like classification or deep learning with just a few clicks. While they simplify model training and speed up deployment, they may not be ideal for complex AI-based tasks like object detection or recommender systems. Compared to traditional code ML tools, they offer less control and may not fully replace data scientists; especially when working with historical data or advanced neural networks. Still, they play a key role in helping democratize data science.
Conclusion
No-code machine learning platforms offer a powerful way to build and deploy machine learning models without any coding. With tools for automated feature engineering, model training, analyzing data and deployment, they make AI and machine learning accessible to everyone, including business analysts and non-data scientists.
The results in this comparison are based on a large, diverse dataset. Since even small differences in input data can affect model performance, these results are not universally applicable. Simpler or smaller datasets may actually produce more accurate predictive models.
Users should consider the size and complexity of their data when selecting a no-code ML platform, to ensure meaningful data analysis and successful model outcomes.
FAQ about No Code Machine Learning Tools
What are the limitations of no-code ML tools?
No-code ML tools often provide limited control over model selection, hyperparameter tuning, and pipeline customization. They may not be suitable for complex AI tasks and typically require high-quality, well-structured input data to deliver accurate results.
Can I use no-code ML for advanced machine-learning tasks?
Most no-code platforms are not designed for object detection, deep learning, or building multi-step machine learning pipelines. These advanced use cases usually require greater flexibility and coding expertise.
How do I get started with no-code ML?
You can start by uploading tabular data, selecting a pre-trained machine learning algorithm, and using automated faster model training and deployment features—no coding needed.
Are there free no-code ML tools available?
Yes. Many no-code machine learning platforms offer free trials or limited-access versions, allowing business analysts and citizen data scientists to explore their capabilities before committing to a paid plan.
What resources can help me learn no-code ML?
Numerous online tutorials, video courses, and user communities cover topics such as machine learning models, data preparation, feature engineering, and model deployment within no-code platforms.
What are the best practices for using no-code ML?
Use clean, well-structured data, choose the right pre-trained model, and monitor model performance regularly. Leverage automated tools for model training and deployment.
Is no-code machine learning models only for beginners?
No. While no-code platforms are great for beginners and business users, they help experienced data scientists accelerate prototyping and automate repetitive workflows, freeing time for more advanced tasks.
Can no-code platforms replace data scientists?
Not completely. These platforms are excellent for automating routine tasks, but advanced functions like custom model tuning, deep learning, and algorithm selection still require expert knowledge and programming skills.
Comments
Your email address will not be published. All fields are required.