Machine Learning Outsourcing in 2024: Benefits & Challenges
According to the “AI adoption in the Enterprise 2022” survey by O’Reilly, around 70% of organizations are using or evaluating to use of AI. Other statistics on AI adoption clearly show that there is a significant interest in AI and ML in businesses as AI/ML provides numerous benefits through a diverse set of applications.
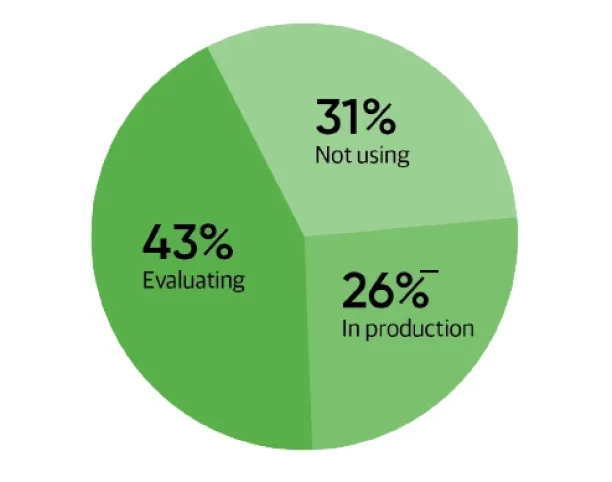
However, successful applications require expertise in areas such as data processing and model building, and not every business has the resources to hire, train, and maintain in-house teams of machine learning professionals. Outsourcing machine learning projects to ML outsourcing companies or consultants is an alternative approach to implementing ML applications in your business.
Why is AI/ML outsourcing relevant now?
One of the most common questions faced by businesses that are planning to embark on a machine learning application is whether to implement it with an in-house team or outsource their ML project to an external AI/ML company. Similar to building in-house AI solutions, building an in-house team for ML projects has some disadvantages:
- There is a shortage of skilled machine learning professionals. According to Global AI Talent Report 2019, the number of AI experts worldwide was only 22,400 at the end of 2018. There is a growing demand for data scientists and AI experts, but the supply of talent does not meet it.
- Machine learning and especially deep learning models require large amounts of high-quality data for better performance. Data discovery and cleaning can be time consuming processes. Even if your organization has access to clean, large scale data relevant to the model, working with big data is time-consuming and requires experience.
- Hiring and training an in-house team is costly and the process can take months.
Outsourcing is thus a good alternative for businesses that need cost-effective and fast ML project delivery.
How is ML outsourcing different than AI outsourcing?
Machine learning is the most common commercial approach to build AI solutions today. Therefore these 2 terms mean the same thing in almost all cases.
There could be AI consultants that do not rely on machine learning, they could rely on symbolic AI which is another artificial intelligence approach. However, as of today, almost no AI consultant would solely rely on symbolic AI since machine learning is broadly applicable and commercially more successful than symbolic AI approaches.
How is ML outsourcing different than ML consulting?
ML outsourcing is exclusively focused on building machine learning models to satisfy client’s requirements while ML consulting has a broader scope. ML consultants help businesses
- formulate their AI/ML strategy considering their strategic goals, challenges and the regulatory and competitive landscape
- identify problems that can be solved with contemporary machine learning approaches
- design projects to transform their businesses with ML
- run all aspects of these projects including stakeholder management and tracking progress
- building machine learning models which is also performed by ML outsourcing companies
Therefore, companies that have the capacity to handle other aspects of ML projects can outsource the model development part to AI/ML outsourcing companies. This allows them to pay lower fees to an ML outsourcing company compared to an AI/ML consultant.
For more on AI/ML consulting, please read our article on AI consulting.
What are the benefits of ML outsourcing?
- Access to expertise: Building and launching a successful ML application is a complicated process and as we mentioned above, hiring and training data science staff and providing the necessary resources to them is costly. Outsourcing enables businesses to find and choose experts based on the necessities of their specific ML application.
- Reduced development time and cost: Outsourcing ML projects to experienced professionals can speed up the development and deployment process. ML outsourcing companies and consultants usually focus on specific sectors or applications. So, there is a good chance that they have already built similar models and have the necessary experience and resources in hand. This saves businesses from experimenting and resource-building processes with in-house teams.
- Better data management: In addition to model development, collecting and working with large datasets also requires experience and time. For instance, data labeling is an indispensable part of supervised learning model development processes, and it is a labor-intensive and time-consuming task. Outsourcing companies would have the necessary experience to mine and process the raw data to make it suitable and efficient for the machine learning model.
- Higher flexibility: Different ML applications require different skills and outsourcing provides flexibility. Businesses with in-house teams that want to execute multiple ML projects would need to spend some time on preparation and training for each application. Instead, companies can work with different outsourcing companies for different ML projects according to their expertise. Furthermore, outsourcing companies can offer different engagement models. For instance, if an application requires more cooperation between data scientists and other staff, outsourcing companies can form a team that would work as an internal team temporarily.
What are the challenges to ML outsourcing?
Sharing private data with outsourcing companies and finding professionals with the necessary domain knowledge are two challenges of outsourcing machine learning projects.
Data sharing
Sharing your company’s data with external parties can be problematic because of data privacy regulations such as GDPR or CCPA. In the case of a data breach, businesses can face serious fines and suffer from reputation damage. There are some solutions to this problem but these methods also have some drawbacks. For more, you can check our articles on data masking, synthetic data and federated learning.
Domain knowledge
If your business objective is too specific, you may find it hard to find experienced professionals with the necessary domain knowledge. Domain knowledge is crucial because raw data from the real world most likely requires processing before ML model training for better performance. This processing requires a good understanding of the domain of the specific application and the sector, in addition to the model and the dataset.
For example, hundreds of COVID-19 diagnosis applications were built by developers who were not aware of biases in the dataset. For example, tomography scans of patients that are lying down tend to be worse than those that are standing up just because people too sick to stand up and that exhibit COVID-19 symptoms tend to have COVID-19. But this can lead a model to identify lying down people and misdiagnose lying down patients without COVID-19. Knowing hospital operating procedures would help data scientists avoid such mistakes
How to choose an ML outsourcing vendor?
- Domain expertise: The vendor should have the necessary experience with projects relevant to your business’ industry or business objective and has expertise in relevant machine learning technologies.
- References: A reputable vendor would have a portfolio to document its previous projects. References are always a good source of information.
- Team: You can also check individual team members’ backgrounds. Knowledge of a diverse set of tools and technologies and teams with diverse backgrounds such as data scientists, programmers, statisticians, designers, etc. can be signs of high-quality work.
- TCO: Cost to build, deploy and maintain the model should be considered.
- Support model: Model accuracies degrade over time and the team should be available for model improvement if model accuracy degrades
In addition to these, procurement best practices would need to be followed as usual.
For more, feel free to check our articles on AI-related consulting:
- AI Consulting: In-depth Guide with Top AI Consulting Firms
- Machine Learning Consulting: In-Depth Guide
- Data Science Consulting & Consultants: In-depth Guide
If you are looking for companies and consultants to outsource your machine learning project, check our data-driven lists of ML software vendors, data science consultants, and AI consultants.
If you still have questions about machine learning outsourcing, we would like to answer them:

Cem has been the principal analyst at AIMultiple since 2017. AIMultiple informs hundreds of thousands of businesses (as per similarWeb) including 60% of Fortune 500 every month.
Cem's work has been cited by leading global publications including Business Insider, Forbes, Washington Post, global firms like Deloitte, HPE, NGOs like World Economic Forum and supranational organizations like European Commission. You can see more reputable companies and media that referenced AIMultiple.
Throughout his career, Cem served as a tech consultant, tech buyer and tech entrepreneur. He advised businesses on their enterprise software, automation, cloud, AI / ML and other technology related decisions at McKinsey & Company and Altman Solon for more than a decade. He also published a McKinsey report on digitalization.
He led technology strategy and procurement of a telco while reporting to the CEO. He has also led commercial growth of deep tech company Hypatos that reached a 7 digit annual recurring revenue and a 9 digit valuation from 0 within 2 years. Cem's work in Hypatos was covered by leading technology publications like TechCrunch and Business Insider.
Cem regularly speaks at international technology conferences. He graduated from Bogazici University as a computer engineer and holds an MBA from Columbia Business School.
To stay up-to-date on B2B tech & accelerate your enterprise:
Follow on
Comments
Your email address will not be published. All fields are required.