Large language models, as part of generative AI, can predict protein structures and characteristics for use in healthcare and other industries. Generative AI in life sciences is transforming the field, driving advancements in drug discovery, personalized medicine, and diagnostics.
Explore 10 use cases of generative AI in life sciences, such as biology, and 3 real-life examples.
10 Use Cases of Generative AI in Life Sciences
Generative AI has found numerous applications in life sciences, helping to drive research and development, optimize processes, and generate new insights. Some use cases include:
1- Novel molecule generation
Generative models, such as variational autoencoders (VAEs) and generative adversarial networks (GANs), can design novel drug-like molecules with desired properties, such as high binding affinity to a target protein or low toxicity.
2- Protein sequence design
Generative models can create novel protein sequences with specific functionalities or properties, which can be useful in protein engineering, enzyme design, and developing novel therapeutics.
Real-life example: Google Deepmind released AlphaProteo to design novel proteins that are likely to bind to target molecules.1
3- Synthetic gene design
Generative AI can be employed to design synthetic gene sequences for applications in synthetic biology, such as creating new biosynthetic pathways or optimizing gene expression for biomanufacturing purposes.
4- Data augmentation for model training
Generative models can generate synthetic data to augment existing datasets, helping improve AI models’ performance in tasks like the analysis of medical images, drug discovery, and accurate diagnosis.
5- Imputation of missing data
Generative AI can help fill in missing medical data in life science datasets, allowing researchers to work with more complete and reliable information for downstream analysis and modeling.
6- Virtual patient generation
Generative models can be used to create synthetic patient and healthcare data, which can be useful for training AI models, simulating clinical trials, or studying rare diseases without access to large real-world datasets.
7- Single-cell RNA sequencing (scRNA-seq) data denoising
Single-cell RNA sequencing (scRNA-seq) is a powerful technique used to study the gene expression profiles of individual cells. Single-cell RNA sequencing (scRNA-seq) data denoising refers to the process of removing noise or unwanted variations from the raw gene expression data obtained through scRNA-seq experiments.
Denoising scRNA-seq data is crucial for obtaining accurate and reliable gene expression profiles of individual cells. This, in turn, enables the correct identification of cell types, differentiation trajectories, and other biologically meaningful insights. Generative models can be used to denoise scRNA-seq data, improving the accuracy of downstream analysis, such as cell-type identification and gene expression quantification.
8- Image-to-image translation
Generative AI models can be employed to convert one type of biological image to another, such as transforming fluorescence microscopy images into electron microscopy images, which can help researchers gain insights from different imaging modalities.
9- Text-to-image generation
Generative models can be used to generate images of biological structures or processes based on textual descriptions, which can be helpful in visualizing complex phenomena or generating data for hypothesis testing.
10- Simulating biological processes
Generative AI can create realistic simulations of biological processes, such as cellular signaling or metabolic pathways, helping researchers better understand these biological systems and predict their behavior under different conditions.
Real Life Examples
Biomatter
Biomatter, a company specializing in synthetic ai biology, employs ProteinGAN, a GPU-powered algorithm, on their Intelligent Architecture™ platform, which combines generative AI and physical modeling (Figure 1). This approach enables them to create entirely novel and functional enzymes.
Figure 1. The working principle of the Intelligent Architecture platform
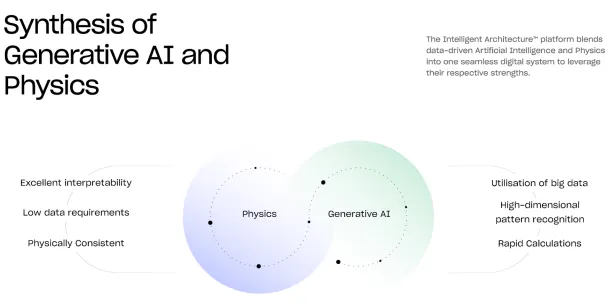
Source: Biomatter
Evozyne
Evozyne integrates engineering and deep learning techniques to develop highly functional synthetic proteins. They utilize the NVIDIA BioNeMo framework to expedite the creation of ProT-VAE, a transformer-based model designed explicitly for protein engineering.
The company works on many bioengineering use cases, such as:
- Gene optimization
- Gene editing
- Generating therapeutic antibodies
Figure 2. Evolutionary data-driven protein engineering by Evozyne
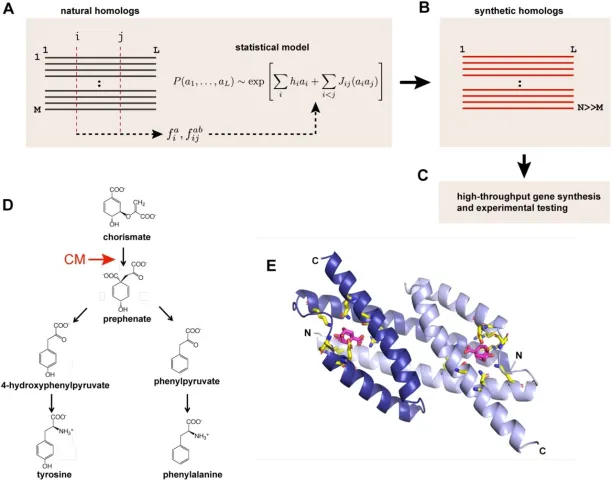
Source: Science
Peptilogics
The Nautilus™ generative AI platform by Peptilogics facilitates peptide drug design and lead optimization across various therapeutic fields and biological targets. Powered by Peptilogics’ custom-built N4 supercomputer, which utilizes NVIDIA GPUs, Nautilus incorporates the company’s proprietary peptide representation and generative algorithms along with computational chemistry and biophysics. This integration helps to decrease the expenses, duration, and risks associated with drug design.
Figure 3. The discovery process of Peptilogics
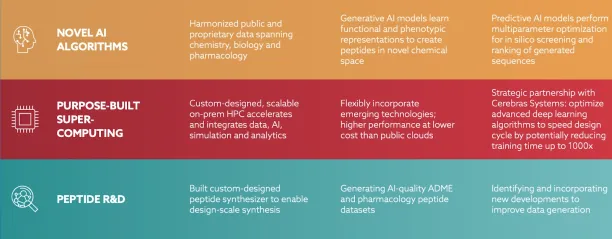
Applications of Generative AI in Life Sciences
1. Drug Discovery and Design
Molecule Generation: Generative AI can design new molecules by analyzing chemical and biological datasets. These models predict molecular structures that meet specific criteria, such as binding affinity, solubility, or toxicity. This process accelerates the traditionally lengthy and expensive drug discovery timeline, enabling the quicker identification of potential drug candidates.
Target Identification: Generative AI helps researchers identify potential targets for drug development by analyzing protein structures and mapping interactions. This capability reduces the time spent on early-stage research by narrowing down viable targets for further study.
2. Protein Structure Prediction
AI models like AlphaFold, developed by DeepMind, have revolutionized protein structure prediction. Using generative techniques, these models predict the 3D shapes of proteins based on their amino acid sequences. This understanding is crucial for designing therapies for diseases associated with specific proteins, thereby accelerating research in enzyme design and vaccine development.
3. Genomics and Personalized Medicine
DNA and RNA Sequence Generation: Generative AI can simulate genetic sequences, enabling researchers to explore genetic variations, potential mutations, and their impact on diseases. These simulations are beneficial in genetic engineering and evolutionary studies.
Personalized Treatment Plans: Generative AI analyzes large datasets of patient-specific information to create tailored treatment recommendations. For example, it can identify the most effective drug based on an individual’s genetic makeup or predict disease progression to optimize care strategies.
4. Synthetic Biology
Generative AI in life sciences is crucial in designing synthetic organisms or modifying biological systems for specific purposes. For example, it can engineer microbes that produce biofuels, develop crops with higher yields, or create synthetic cells for pharmaceutical production. This has broad implications for healthcare, agriculture, and environmental sustainability.
5. Medical Imaging and Diagnostics
Generative AI in life sciences models can create high-quality synthetic medical images, such as CT scans or MRIs, which train diagnostic models. These synthetic datasets help overcome the challenge of limited labeled medical data and improve diagnostic accuracy in identifying conditions like cancer, heart disease, or neurological disorders.
6. Clinical Trials Optimization
Patient Recruitment: Generative AI in life sciences analyzes health records, genetic data, and patient demographics to identify suitable candidates for clinical trials, ensuring that trials are diverse and representative.
Simulation Models: Generative AI predicts the potential outcomes of new therapies by creating virtual trials, allowing researchers to refine trial designs and reduce costs. This approach facilitates the assessment of drug efficacy and safety without the immediate need for large-scale human trials.
FAQ
What is Generative AI in life sciences?
Generative AI in life sciences refers to the application of artificial intelligence models that generate new insights, data, or solutions based on existing biological, chemical, or clinical data. This includes generating novel drug compounds, predicting protein structures, and simulating clinical trial outcomes, among other applications.
How is Generative AI used in drug discovery?
Generative AI in life sciences is used in drug discovery to analyze large datasets of chemical compounds, biological interactions, and genetic information. It generates potential drug candidates by predicting how molecules will interact with biological targets. AI-driven platforms like Insilico Medicine and Exscientia are currently identifying promising drug candidates for diseases like cancer and fibrosis.
Can Generative AI help in personalized medicine?
Yes, Generative AI in life sciences is used to create personalized treatment plans by analyzing a patient’s genetic, molecular, and lifestyle data. By generating predictive models of treatment responses, AI helps healthcare providers tailor therapies to individual patients, improving outcomes and reducing side effects. Companies like Tempus use AI to offer personalized oncology treatments.
How does Generative AI assist with clinical trials?
Generative AI helps optimize clinical trials by simulating patient outcomes and trial scenarios. AI can predict the success of a trial, assist in designing efficient protocols, and even generate digital twins of patients, reducing the need for large control groups. Tools like Unlearn.AI use AI to shorten trial times and improve success rates.
What are the challenges of using Generative AI in life sciences?
Some key challenges include:
Data privacy and security: Handling sensitive patient data in compliance with regulations like GDPR and HIPAA.
Bias in AI models: AI systems may inherit biases from their training data, potentially leading to unequal treatment outcomes.
Regulatory approval: AI-generated insights must meet strict regulatory requirements for safety and efficacy.
Model interpretability: Many AI models are “black boxes,” meaning their decision-making process is difficult to explain or interpret. Explore more on AI explainability.
Mitigate these challenges by using:
1. AI governance tools
2. Responsible AI platforms.
These tools can ensure AI compliance, manage an AI inventory, mitigate AI bias, and other generative AI risks.
Comments
Your email address will not be published. All fields are required.